Optimal control of partially observable discrete time stochastic hybrid systems for safety specifications
American Control Conference(2013)
摘要
This paper describes a theoretical framework for the design of controllers to satisfy probabilistic safety specifications for partially observable discrete time stochastic hybrid systems. We formulate the problem as a partial information stochastic optimal control problem, in which the objective is to maximize the probability that the state trajectory remains within a given safe set in the hybrid state space, using observations of the history of inputs and outputs. It is shown that this optimal control problem, which has a multiplicative payoff structure, is equivalent to a terminal payoff problem when the state space is augmented with a binary random variable capturing the safety of past state evolution. This allows us to derive a sufficient statistic for the probabilistic safety problem as a set of Bayesian filtering equations updating a conditional distribution on the augmented state space, as well as an abstract dynamic programming algorithm for computing the maximal probability of safety and an optimal control policy.
更多查看译文
关键词
Bayes methods,control system synthesis,discrete time systems,dynamic programming,observability,optimal control,state-space methods,statistical distributions,stochastic systems,Bayesian filtering equations,abstract dynamic programming algorithm,augmented state space,binary random variable,conditional distribution,controller design,hybrid state space,input history observation,maximal safety probability,multiplicative payoff structure,output history observation,partial information stochastic optimal control problem,partially-observable discrete time stochastic hybrid systems,probabilistic safety specifications,state trajectory,sufficient statistics,terminal payoff problem
AI 理解论文
溯源树
样例
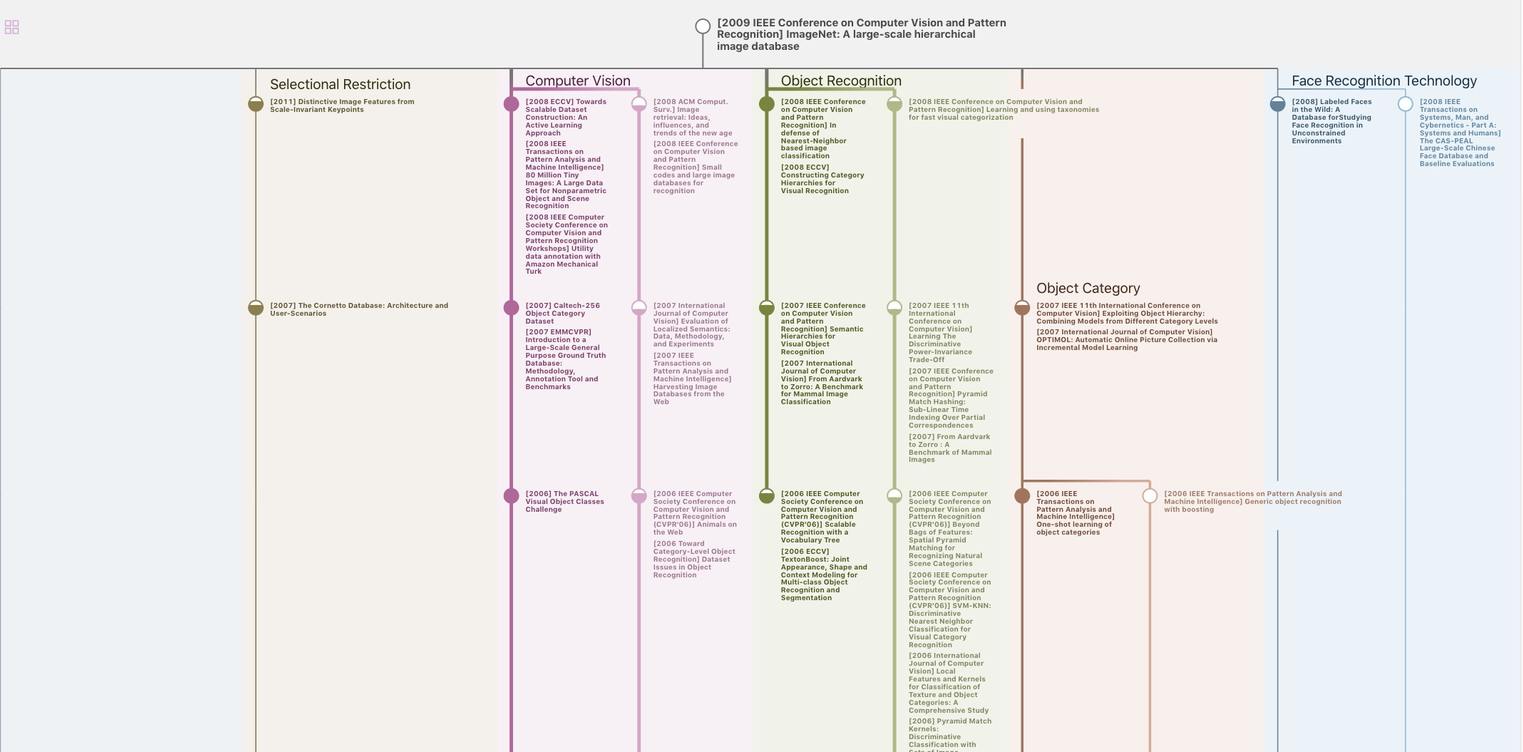
生成溯源树,研究论文发展脉络
Chat Paper
正在生成论文摘要