Spatial function estimation using Gaussian process with sparse history data in mobile sensor networks
Computer Science and Electronic Engineering Conference(2012)
摘要
This paper presents a sparse history data based method for modelling a latent function with mobile wireless sensor networks. It contains two main tasks, which are estimating the latent function and optimising the sensor deployment. Gaussian process (GP) is selected as the framework according to its excellent regression performance. History data can improve the modelling performance with small amount of sensors in static or slowly changed environment. However, the GP kernel size is expended. On the one hand, in other kernel based (or non-parametric) methods, computation cost increases fast with kernel size. To control the size of GP kernel, informative vector machine (IVM) is introduced for history data selection. On the other hand, centroidal Voronoi tessellation (CVT), a gradient based method, is adopted for optimising sensor deployment. Simulation results with different data selection methods and analyses are given. It's proved that the data selection is effective in reducing computation cost and keeping the precision of the estimated model.
更多查看译文
关键词
gaussian processes,computational geometry,gradient methods,mobile communication,regression analysis,wireless sensor networks,gp kernel,gaussian process,centroidal voronoi tessellation,gradient based method,informative vector machine,latent function,mobile wireless sensor networks,sensor deployment,sparse history data,spatial function estimation
AI 理解论文
溯源树
样例
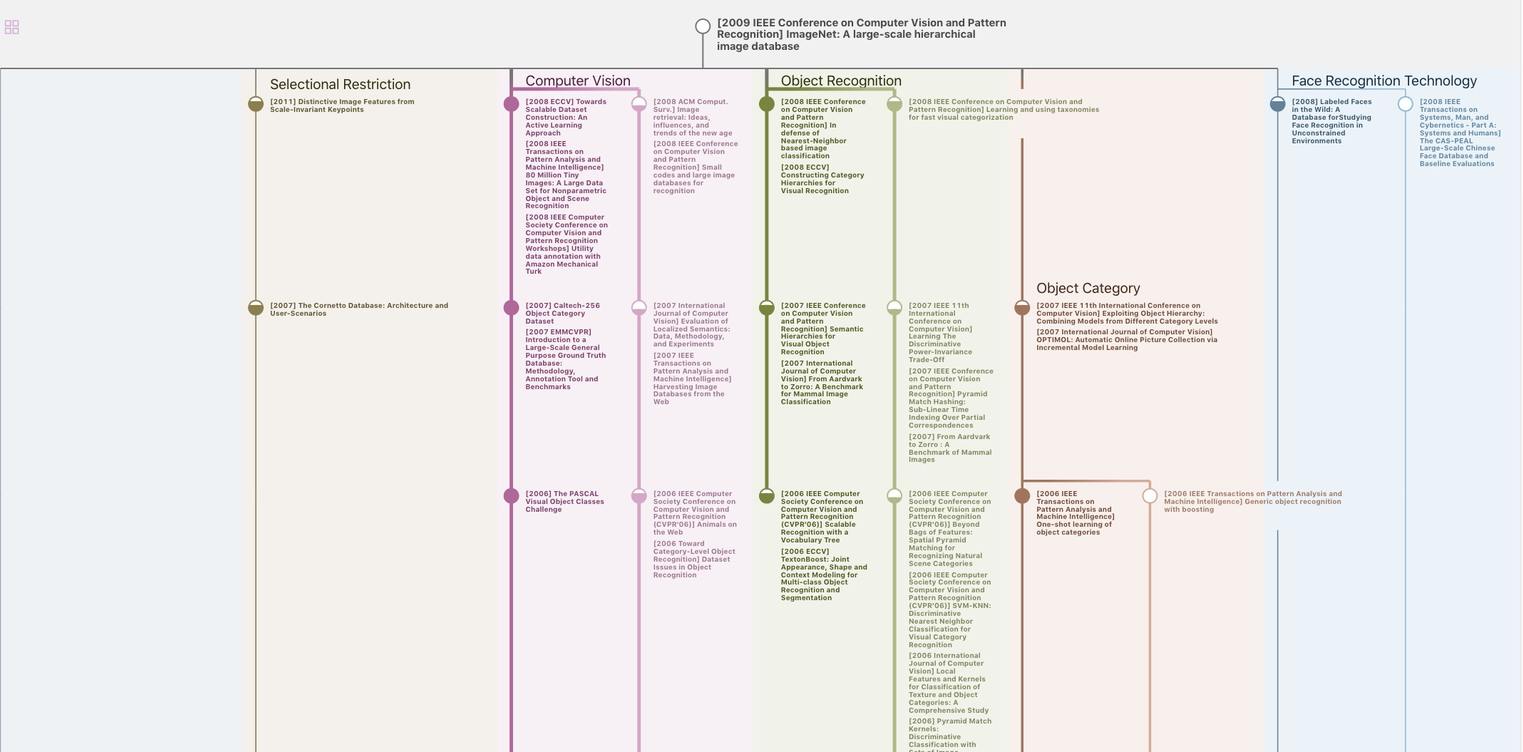
生成溯源树,研究论文发展脉络
Chat Paper
正在生成论文摘要