Classification of Malicious Web Sessions
Computer Communications and Networks(2012)
摘要
The ever increasing number of vulnerabilities and reported attacks on Web systems clearly illustrate the need for better understanding of malicious cyber activities, which will allow better protection, detection, and service recovery in the cyberspace. In this paper we use three supervised machine learning methods, Support Vector Machines (SVM), and decision trees based J48 and PART, to classify attacker activities aimed at Web systems. The empirical analysis is based on four datasets, each in duration of four to five months, collected by high-interaction honeypots. Malicious Web sessions are characterized with forty three different features (i.e., session attributes) extracted from Web server logs. Our results show that the supervised learning methods can be used to efficiently distinguish attack sessions from vulnerability scan sessions, with very high probability of detection and very low probability of false alarms. Furthermore, we follow the principle of Occam's razor, that is, we seek for the simplest possible model that can successfully classify malicious Web sessions. Our results show that attacks differ from vulnerability scans only in a small number of features (i.e., session attributes). In particular, depending on the data set, classification of malicious activities can be performed using from four to six features without significantly affecting learners' performance compared to when all 43 features are used. Decision tree based methods J48 and PART perform better than SVM across all datasets.
更多查看译文
关键词
Internet,authorisation,decision trees,learning (artificial intelligence),Occam razor,PART,SVM,Web server logs,Web system,decision trees based J48,high-interaction honeypots,malicious Web session classification,malicious cyber activity,session attribute,supervised machine learning method,support vector machine,vulnerability scan session
AI 理解论文
溯源树
样例
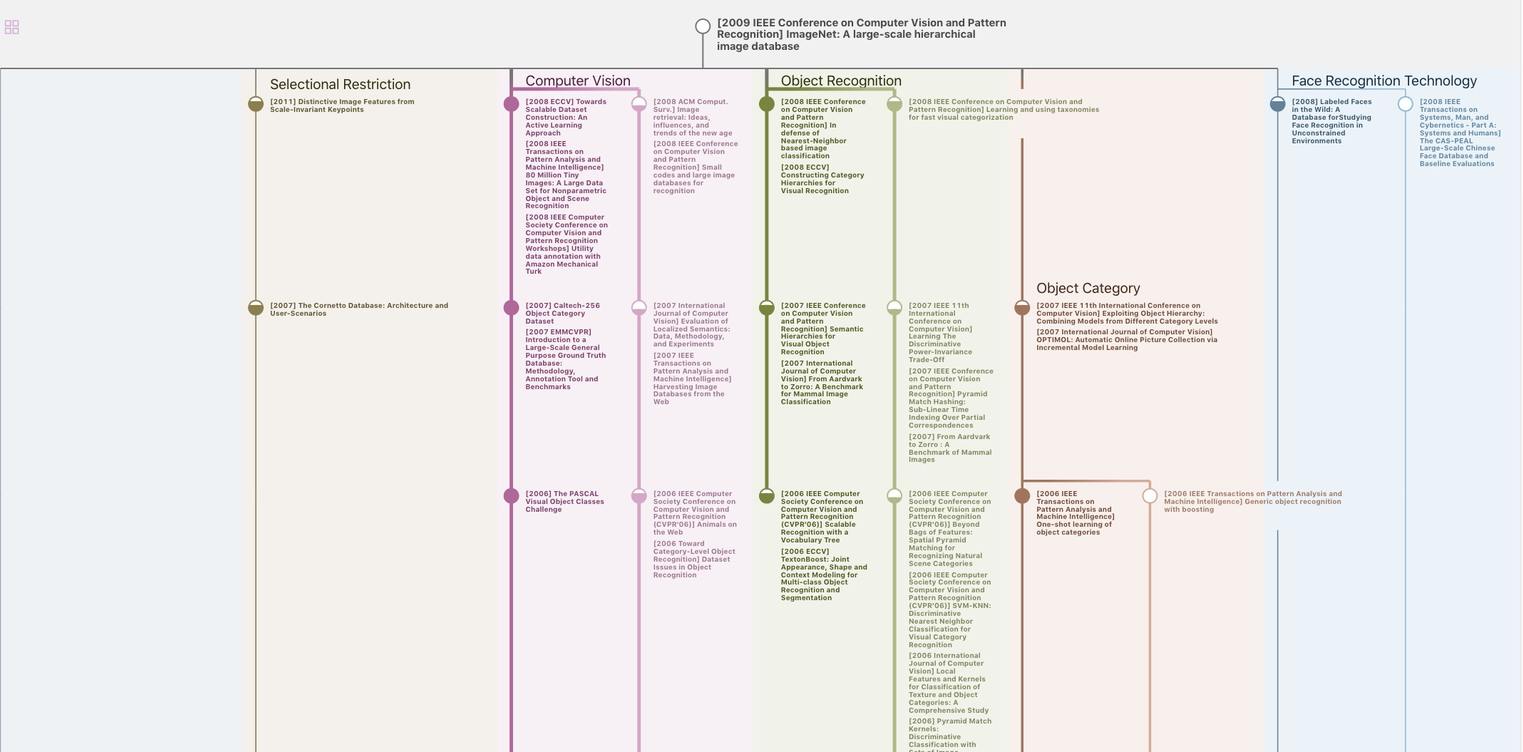
生成溯源树,研究论文发展脉络
Chat Paper
正在生成论文摘要