Self-adaptive memetic algorithm: an adaptive conjugate gradient approach
Cybernetics and Intelligent Systems, 2004 IEEE Conference(2004)
摘要
Combining hill climbing methods that search for the optimum points in a bounded region of search space with genetic algorithm is effective in the cases that the search space or optimization problem is complicated. However, parameter setting of selected HC can influence the performance of the algorithm significantly. In this paper a run-time self-adaptation strategy is utilized to discover the most appropriate HC parameters for the problem at hand. The HC method is used in this work is conjugate gradient that is an efficient gradient based hill climber for a wide range of problems. Traditionally, key parameters of the conjugate gradient are tuned by some deterministic or predetermined adaptive rules. But in our self-adaptation approach these parameters are encoded in genotypes and coevolved alongside the solutions and adjusted based on regional or generational conditions of individuals in the evolution process. Another advantage of this individualistic approach is that it puts forth different hill climbing capabilities to each individual and this prevents undesirable convergence of solutions to a local optimum that is a side effect of ordinary memetic algorithm. This proposed method not only adds no extra computation load to the genetic algorithm but also eliminates computation burden of parameter adjustment of hill climbing operator. Results of applying this approach on several test functions are demonstrated to illustrate improvements achieved using our self adaptive memetic algorithm in comparison with ordinary memetic algorithm.
更多查看译文
关键词
conjugate gradient methods,genetic algorithms,search problems,conjugate gradient approach,genetic algorithm,hill climbing methods,optimization problem,self adaptive memetic algorithm,search space,hill climbing,side effect,conjugate gradient,memetic algorithm
AI 理解论文
溯源树
样例
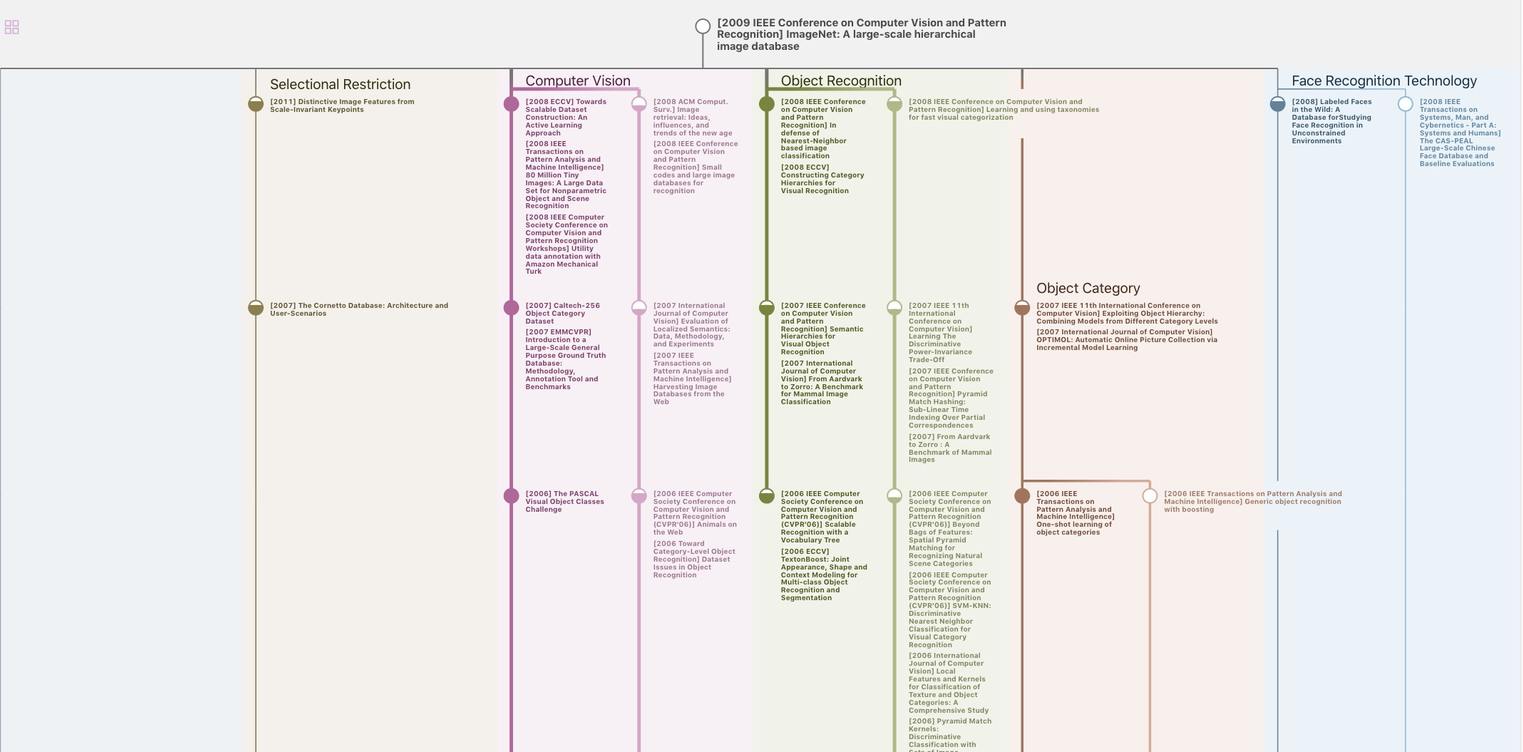
生成溯源树,研究论文发展脉络
Chat Paper
正在生成论文摘要