Ensemble Manifold Rank Preserving for Acceleration-Based Human Activity Recognition.
IEEE Trans. Neural Netw. Learning Syst.(2016)
摘要
With the rapid development of mobile devices and pervasive computing technologies, acceleration-based human activity recognition, a difficult yet essential problem in mobile apps, has received intensive attention recently. Different acceleration signals for representing different activities or even a same activity have different attributes, which causes troubles in normalizing the signals. We thus cannot directly compare these signals with each other, because they are embedded in a nonmetric space. Therefore, we present a nonmetric scheme that retains discriminative and robust frequency domain information by developing a novel ensemble manifold rank preserving (EMRP) algorithm. EMRP simultaneously considers three aspects: 1) it encodes the local geometry using the ranking order information of intraclass samples distributed on local patches; 2) it keeps the discriminative information by maximizing the margin between samples of different classes; and 3) it finds the optimal linear combination of the alignment matrices to approximate the intrinsic manifold lied in the data. Experiments are conducted on the South China University of Technology naturalistic 3-D acceleration-based activity dataset and the naturalistic mobile-devices based human activity dataset to demonstrate the robustness and effectiveness of the new nonmetric scheme for acceleration-based human activity recognition.
更多查看译文
关键词
intrinsic manifold approximation,spectral geometry,frequency domain information,rank order,human activity recognition,acceleration signals
AI 理解论文
溯源树
样例
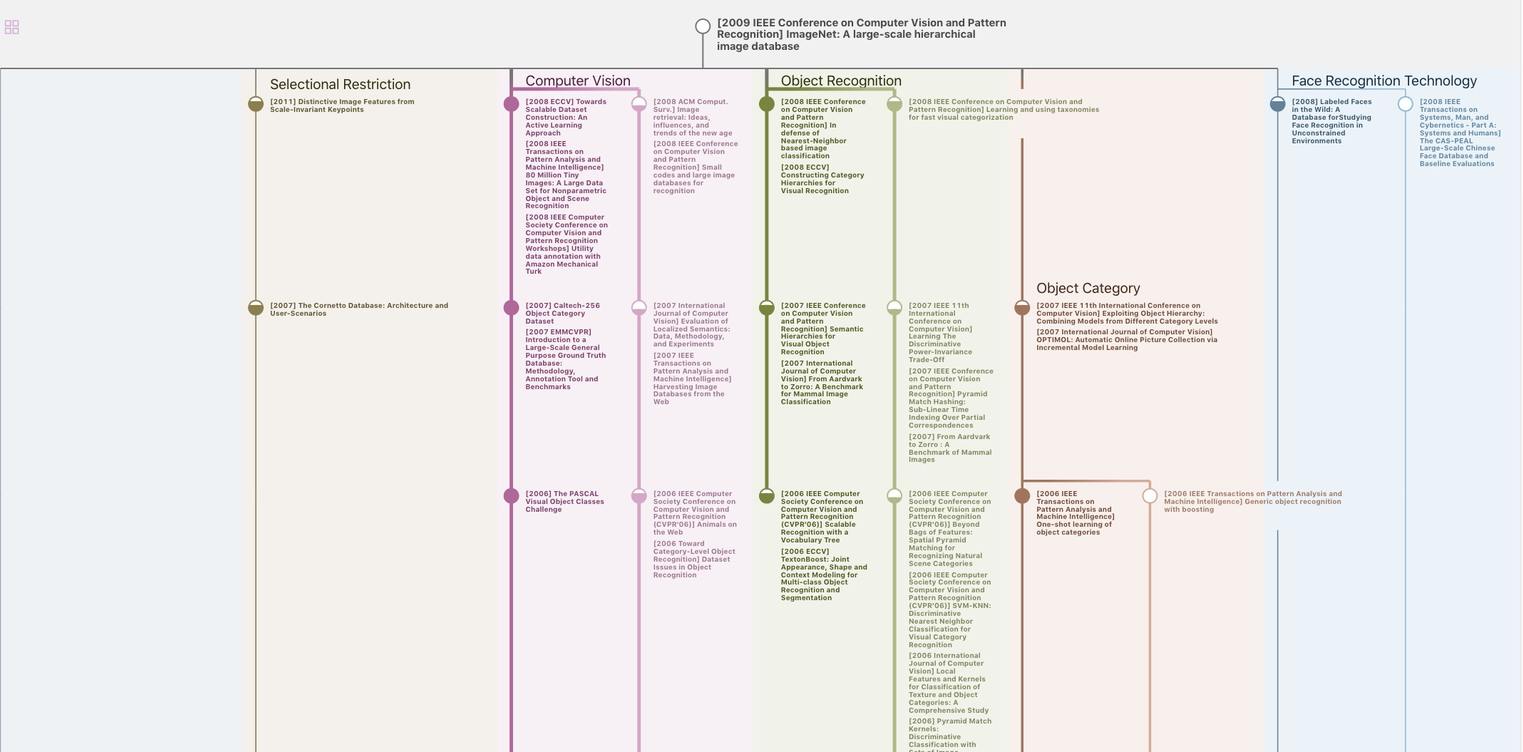
生成溯源树,研究论文发展脉络
Chat Paper
正在生成论文摘要