On regression methods for virtual metrology in semiconductor manufacturing
Irish Signals & Systems Conference 2014 and 2014 China-Ireland International Conference Information and Communications Technologies(2014)
摘要
Virtual metrology (VM) aims to predict metrology values using sensor data from production equipment and physical metrology values of preceding samples. VM is a promising technology for the semiconductor manufacturing industry as it can reduce the frequency of in-line metrology operations and provide supportive information for other operations such as fault detection, predictive maintenance and run-to-run control. The prediction models for VM can be from a large variety of linear and nonlinear regression methods and the selection of a proper regression method for a specific VM problem is not straightforward, especially when the candidate predictor set is of high dimension, correlated and noisy. Using process data from a benchmark semiconductor manufacturing process, this paper evaluates the performance of four typical regression methods for VM: multiple linear regression (MLR), least absolute shrinkage and selection operator (LASSO), neural networks (NN) and Gaussian process regression (GPR). It is observed that GPR performs the best among the four methods and that, remarkably, the performance of linear regression approaches that of GPR as the subset of selected input variables is increased. The observed competitiveness of high-dimensional linear regression models, which does not hold true in general, is explained in the context of extreme learning machines and functional link neural networks.
更多查看译文
关键词
gaussian processes,learning (artificial intelligence),manufacturing processes,neural nets,production engineering computing,regression analysis,semiconductor device manufacture,semiconductor device measurement,shrinkage,gpr,gaussian process regression,lasso,mlr,nn,vm,extreme learning machines,fault detection,frequency reduction,functional link neural networks,high-dimensional linear regression models,in-line metrology operations,least absolute shrinkage and selection operator,metrology values prediction,multiple linear regression,nonlinear regression methods,physical metrology values,prediction models,predictive maintenance,production equipment,run-to-run control,semiconductor manufacturing industry,semiconductor manufacturing process,sensor data,virtual metrology,cross validation,gaussian process regression (gpr),least absolute shrinkage and selection operator (lasso),multiple linear regression (mlr),neural networks (nn),virtual metrology (vm)
AI 理解论文
溯源树
样例
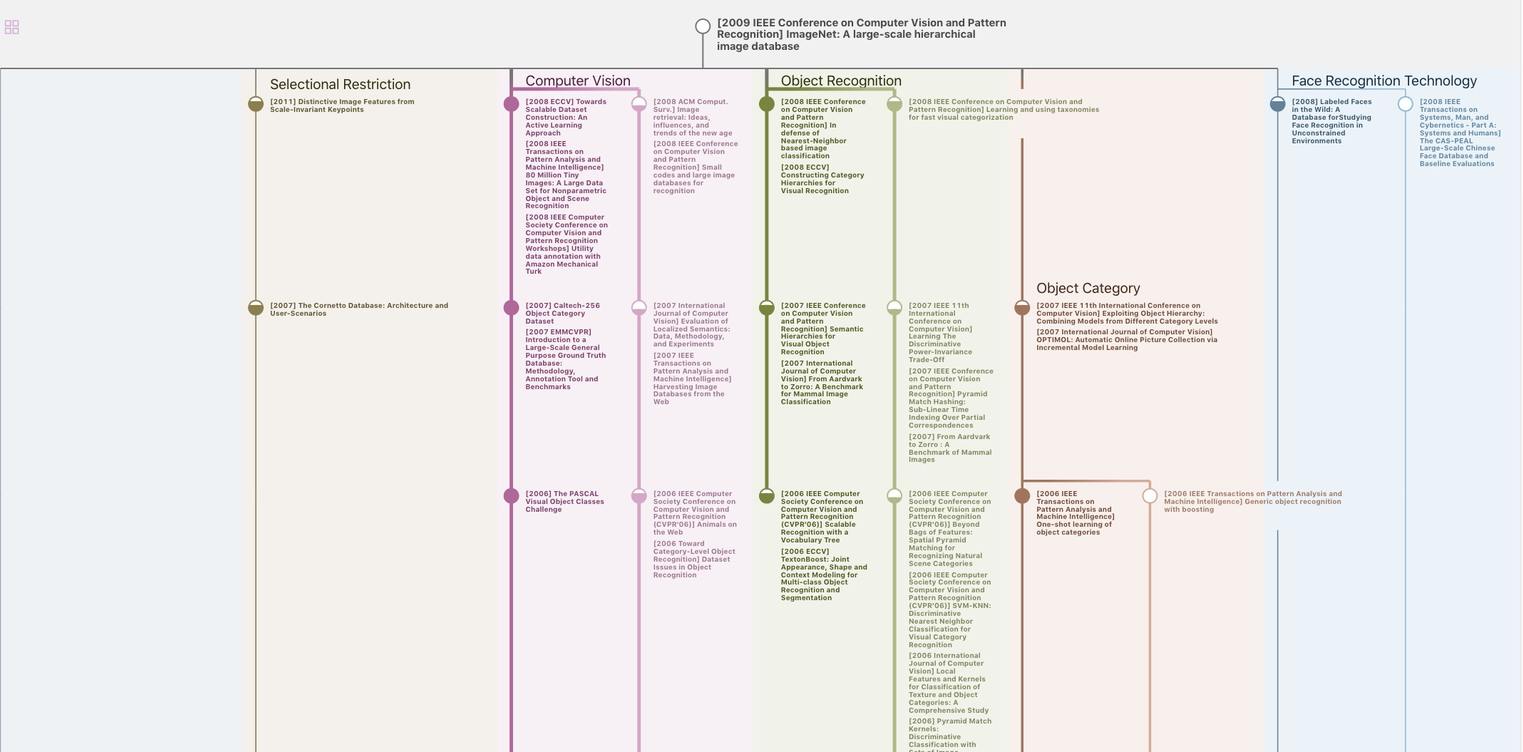
生成溯源树,研究论文发展脉络
Chat Paper
正在生成论文摘要