Automatic fusion and classification of hyperspectral and LiDAR data using random forests
Geoscience and Remote Sensing Symposium(2014)
摘要
In this paper we discuss the use of the random forest algorithm for automatic fusion and classification of hyperspectral and LiDAR data. We demonstrate how relative feature relevance can be used in random forests to perform automatic and unsupervised feature selection. This allows using a large number of features without suffering from the curse of dimensionality. The effectiveness of the proposed approach is demonstrated on two datasets. The first dataset features a combination of hyperspectral and LiDAR data for urban classification whereas the second dataset is the well-known Indian Pines dataset featuring pure hyperspectral imagery. We show that by using the proposed approach classification accuracies can be improved significantly.
更多查看译文
关键词
feature selection,geophysics computing,hyperspectral imaging,optical radar,pattern classification,random processes,sensor fusion,Indian Pines dataset,LiDAR data,automatic data fusion,automatic unsupervised feature selection,data classification accuracies,hyperspectral data,pure hyperspectral imagery,random forest algorithm,urban classification
AI 理解论文
溯源树
样例
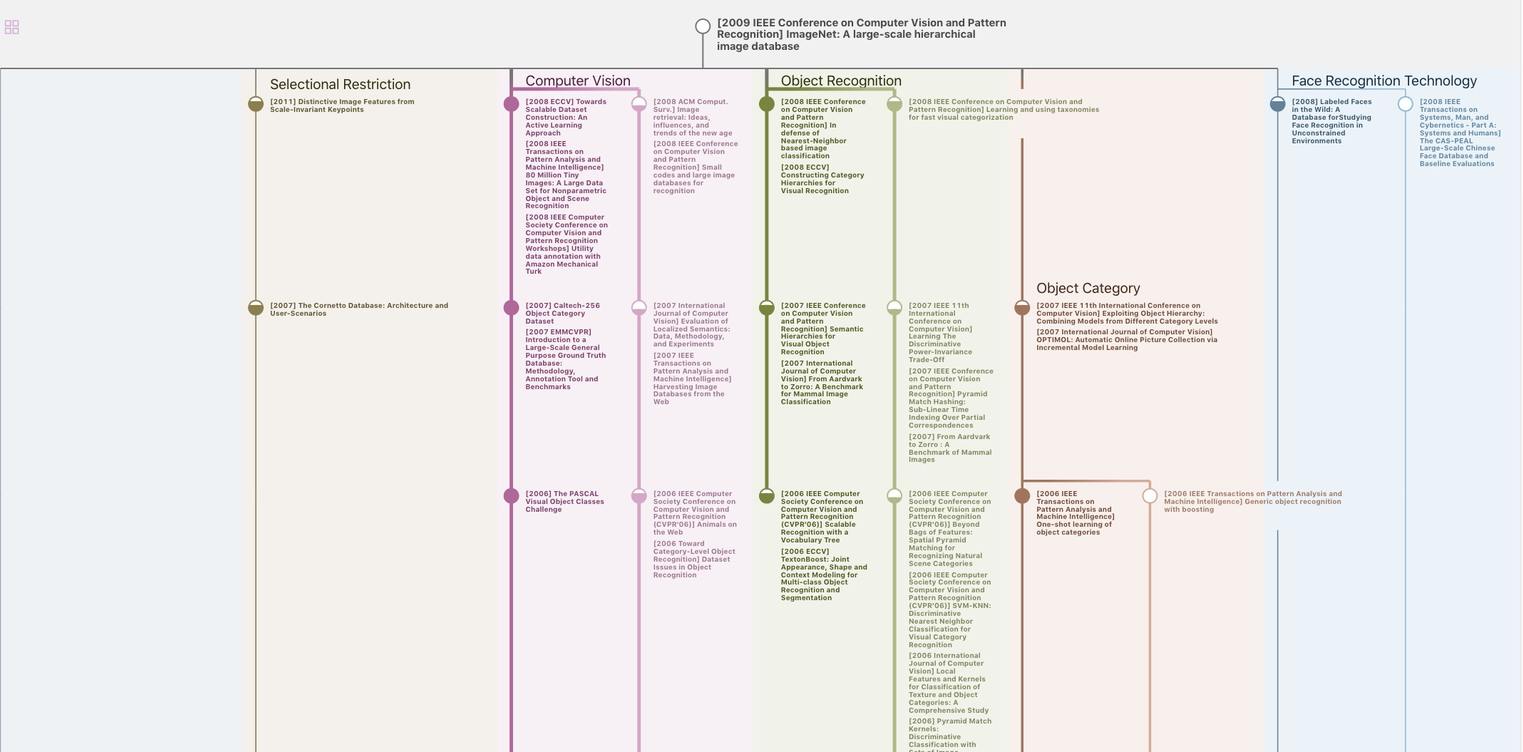
生成溯源树,研究论文发展脉络
Chat Paper
正在生成论文摘要