Low-complexity remote compressive sensing for machine-to-machine networks with stochastic sources
Intelligent Green Building and Smart Grid(2014)
摘要
In recent years, machine-to-machine (M2M) networks have been widely considered in wireless communication systems. Machines typically have constrained power, and their processing and communication capabilities are limited. To avoid the transmission of redundant information in order to save the transmission power, compressive sensing (CS) is a promising tool to consider. In this paper, under the two-tier architecture, we propose a remote CS scheme for the M2M networks with stochastic sources. We first formulate a stochastic CS problem and verify that the selected power exponential model for the stochastic source is compressible, i.e., satisfies the power law. Then we propose to use the minimum mean square error (MMSE) estimator at the gateway to transform the problem into a noiseless stochastic CS one where a low-complexity sensing matrix is adopted. After that we derive an estimation error upper bound for the proposed scheme and prove that it is asymptotically optimal in the MMSE sense compared to the one without CS. We then use simulation to show that the proposed system is still approximately optimal when the observation length is finite.
更多查看译文
关键词
compressed sensing,estimation theory,least mean squares methods,matrix algebra,radio networks,stochastic processes,m2m networks,mmse estimator,estimation error upper bound,low-complexity remote compressive sensing,low-complexity sensing matrix,machine-to-machine networks,minimum mean square error,noiseless stochastic cs problem,power law,redundant information transmission,remote cs scheme,selected power exponential model,stochastic sources,transmission power,two-tier architecture,wireless communication systems,sensors,signal reconstruction,logic gates,decoding
AI 理解论文
溯源树
样例
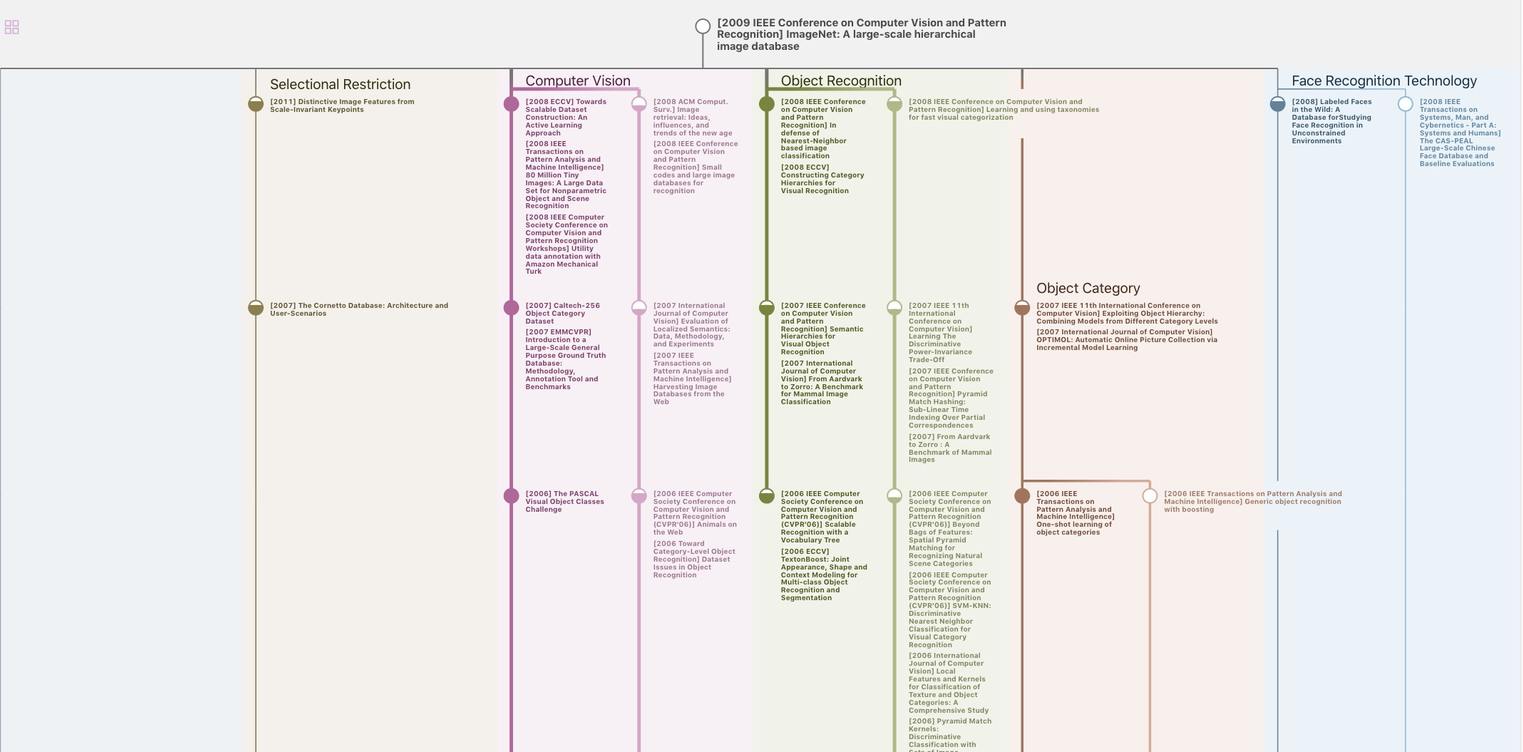
生成溯源树,研究论文发展脉络
Chat Paper
正在生成论文摘要