18.3 A multi-parameter signal-acquisition SoC for connected personal health applications
Solid-State Circuits Conference Digest of Technical Papers(2014)
摘要
Connected personal healthcare, or Telehealth, requires smart, miniature wearable devices that can collect and analyze physiological and environmental parameters during a user's daily routine. To truly support emerging applications (Fig. 18.3.1), a generic platform is needed that can acquire a multitude of sensor modalities and has generic energy-efficient signal processing capabilities. SoC technology gives significant advantages for miniaturization. But meeting low-power, medical grade signal quality, multi-sensor support and generic signal processing is still a challenge. For instance, [1] demonstrated a multi-sensor interface but it lacks support for efficient on-chip signal processing and doesn't have a high performance AFE. [2] showed a very low power signal processor but without support for multi-sensor interfacing. [3] presented a highly integrated SoC but lacking power efficiency. This paper demonstrates a highly integrated low-power SoC with enough flexibility to support many emerging applications. A wide range of sensor modalities are supported including 3-lead ECG and bio-impedance via high-performance and low-power AFE. The ARM Cortex™ M0 processor and matrix-multiply-accumulate accelerator can execute numerous biomedical signal processing algorithms (e.g. Independent Component Analysis (ICA), Principal Component Analysis (PCA,) CWT, feature extraction/classification, etc.) in an energy efficient way without sacrificing flexibility. The diversity in supported modalities and the generic processing capabilities, all provided in a single-chip low-power solution, make the proposed SoC a key enabler for emerging personal health applications (Fig. 18.3.1).
更多查看译文
关键词
electrocardiography,low-power electronics,medical signal processing,sensor fusion,system-on-chip,telemedicine,afe,arm cortex m0 processor,cwt,ica,pca,telehealth,bioimpedance,biomedical signal processing,energy efficient signal processing,feature classification,feature extraction,independent component analysis,low power signal processor,matrix multiply accumulate accelerator,medical grade signal quality,miniature wearable devices,multiparameter signal acquisition soc,multisensor interface,multisensor support,on chip signal processing,personal health,principal component analysis,sensor modalities,three lead ecg,system on chip,low power electronics
AI 理解论文
溯源树
样例
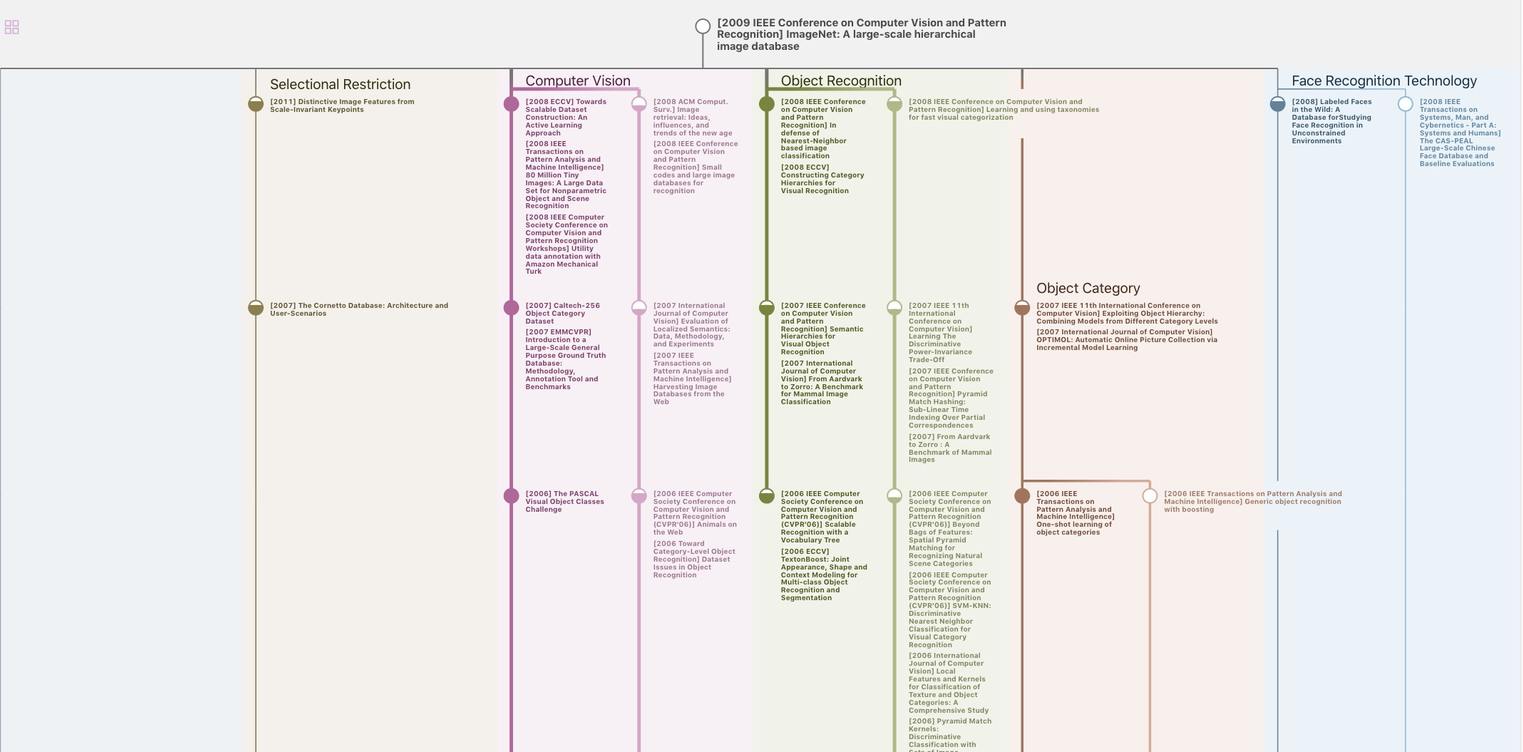
生成溯源树,研究论文发展脉络
Chat Paper
正在生成论文摘要