Prediction of heart failure decompensation events by trend analysis of telemonitoring data.
IEEE journal of biomedical and health informatics(2015)
摘要
This work aims to assess the predictive value of physiological data daily collected in a telemonitoring study in the early detection of heart failure decompensation events. The main hypothesis is that physiological time series with similar progression (trends) may have prognostic value in future clinical states (decompensation or normal condition). The strategy is composed of two main steps: a trend similarity analysis and a predictive procedure. The similarity scheme combines the Haar wavelet decomposition, in which signals are represented as linear combinations of a set of orthogonal bases, with the Karhunen-Loève transform, that allows the selection of the reduced set of bases that capture the fundamental behaviour of the time series. The prediction process assumes that future evolution of current condition can be inferred from the progression of past physiological time series. Therefore, founded on the trend similarity measure, a set of time series presenting a progression similar to the current condition is identified in the historical data set, which is then employed, through a nearest neighbour approach, in the current prediction. The strategy is evaluated using physiological data resulting from the myHeart telemonitoring study, namely blood pressure, respiration rate, heart rate and body weight collected from 41 patients (15 decompensation events and 26 normal conditions). The obtained results suggest, in general, that the physiological data have predictive value and, in particular, that the proposed scheme is particularly appropriate to address the early detection of heart failure decompensation.
更多查看译文
关键词
physiology,wavelet transforms,market research,time series analysis
AI 理解论文
溯源树
样例
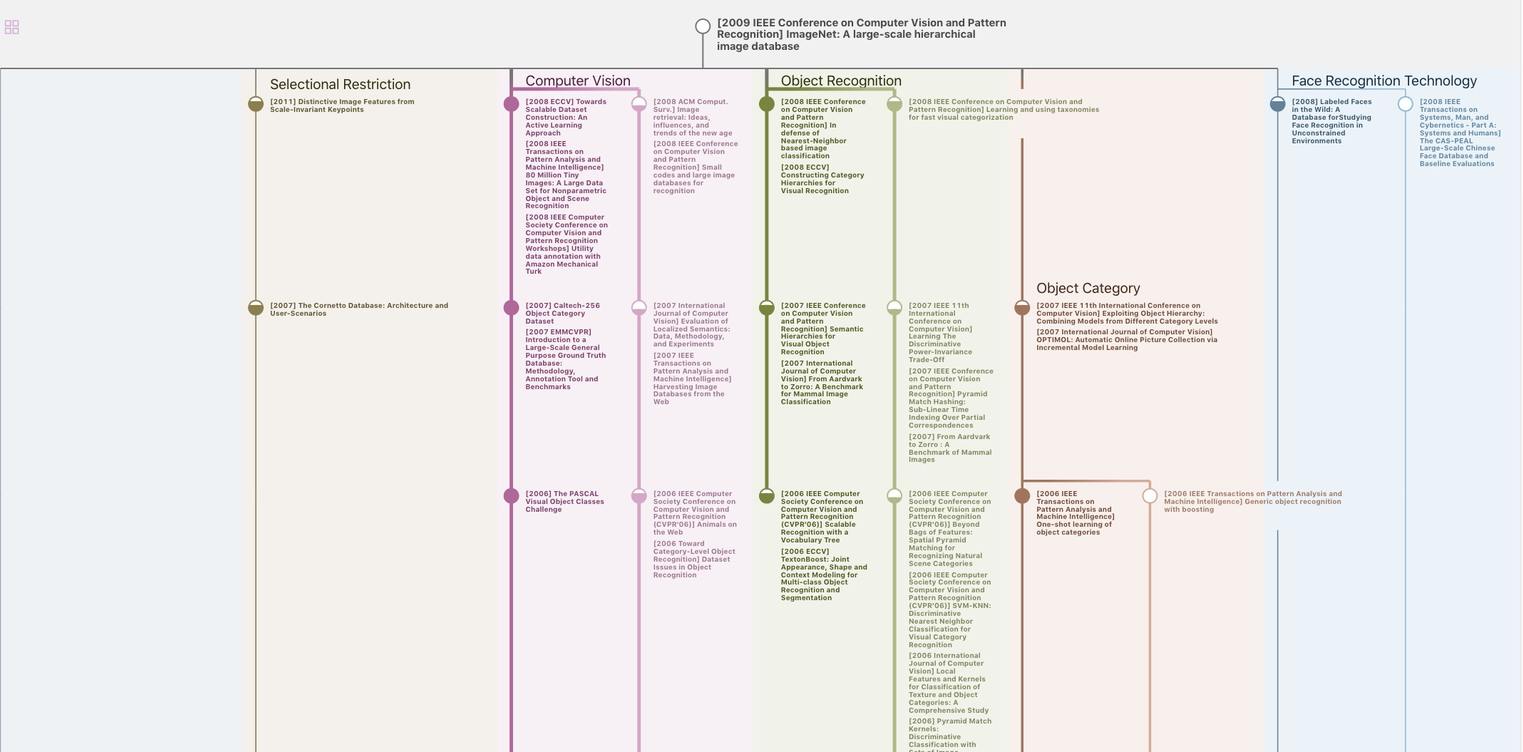
生成溯源树,研究论文发展脉络
Chat Paper
正在生成论文摘要