Fine-Grained Visual Comparisons with Local Learning
Computer Vision and Pattern Recognition(2014)
摘要
Given two images, we want to predict which exhibits a particular visual attribute more than the other -- even when the two images are quite similar. Existing relative attribute methods rely on global ranking functions, yet rarely will the visual cues relevant to a comparison be constant for all data, nor will humans' perception of the attribute necessarily permit a global ordering. To address these issues, we propose a local learning approach for fine-grained visual comparisons. Given a novel pair of images, we learn a local ranking model on the fly, using only analogous training comparisons. We show how to identify these analogous pairs using learned metrics. With results on three challenging datasets -- including a large newly curated dataset for fine-grained comparisons -- our method outperforms state-of-the-art methods for relative attribute prediction.
更多查看译文
关键词
computer vision,learning (artificial intelligence),analogous training comparisons,computer vision system,curated dataset,fine-grained visual comparisons,global ranking functions,local learning,local ranking model,relative attribute methods,relative attribute prediction,visual attribute,visual cues,fine-grained,learning-to-rank,local learning,relative attributes
AI 理解论文
溯源树
样例
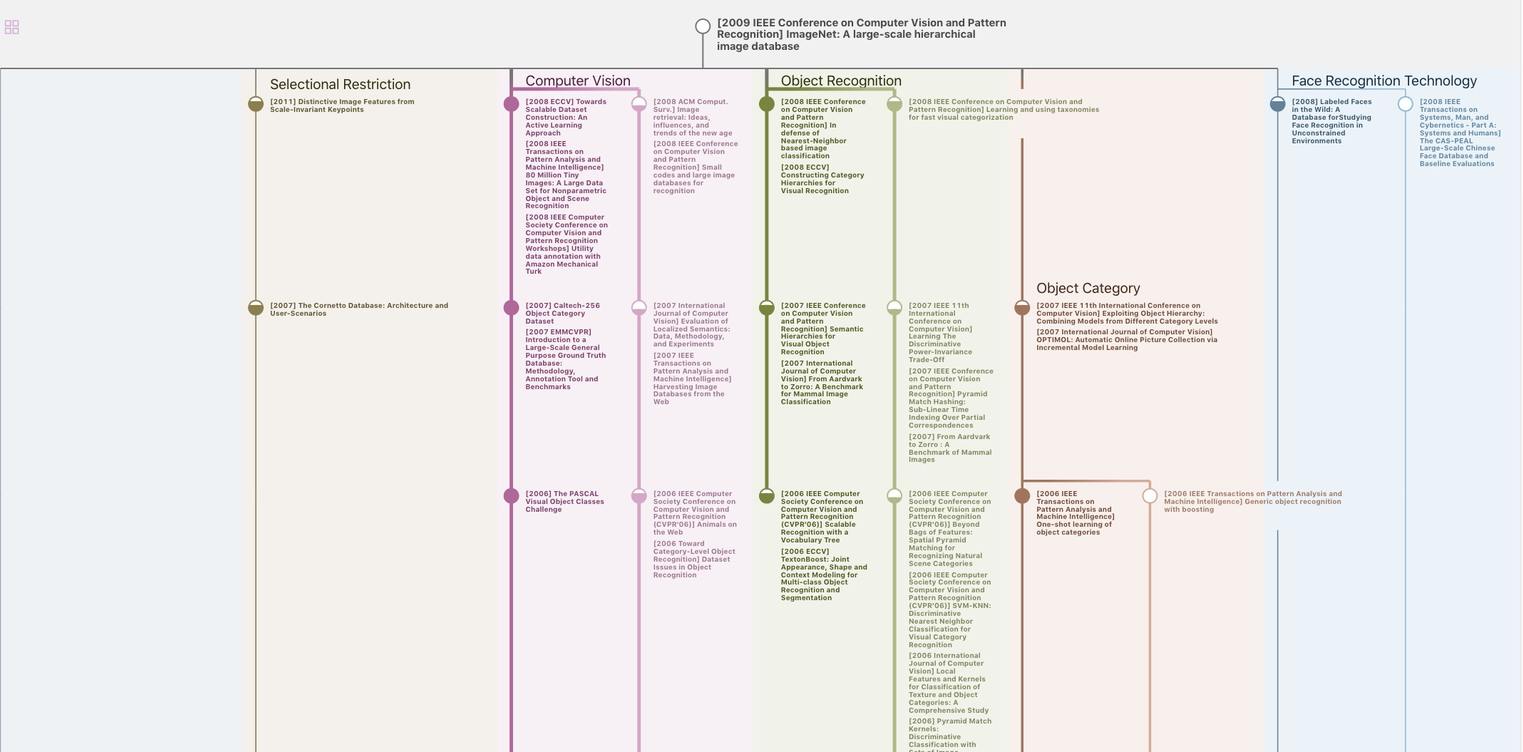
生成溯源树,研究论文发展脉络
Chat Paper
正在生成论文摘要