Deep Network Cascade For Image Super-Resolution
COMPUTER VISION - ECCV 2014, PT V(2014)
摘要
In this paper, we propose a new model called deep network cascade (DNC) to gradually upscale low-resolution images layer by layer, each layer with a small scale factor. DNC is a cascade of multiple stacked collaborative local auto-encoders. In each layer of the cascade, non-local self-similarity search is first performed to enhance high-frequency texture details of the partitioned patches in the input image. The enhanced image patches are then input into a collaborative local auto-encoder (CLA) to suppress the noises as well as collaborate the compatibility of the overlapping patches. By closing the loop on non-local self-similarity search and CLA in a cascade layer, we can refine the super-resolution result, which is further fed into next layer until the required image scale. Experiments on image super-resolution demonstrate that the proposed DNC can gradually upscale a low-resolution image with the increase of network layers and achieve more promising results in visual quality as well as quantitative performance.
更多查看译文
关键词
Super-resolution, Auto-encoder, Deep learning
AI 理解论文
溯源树
样例
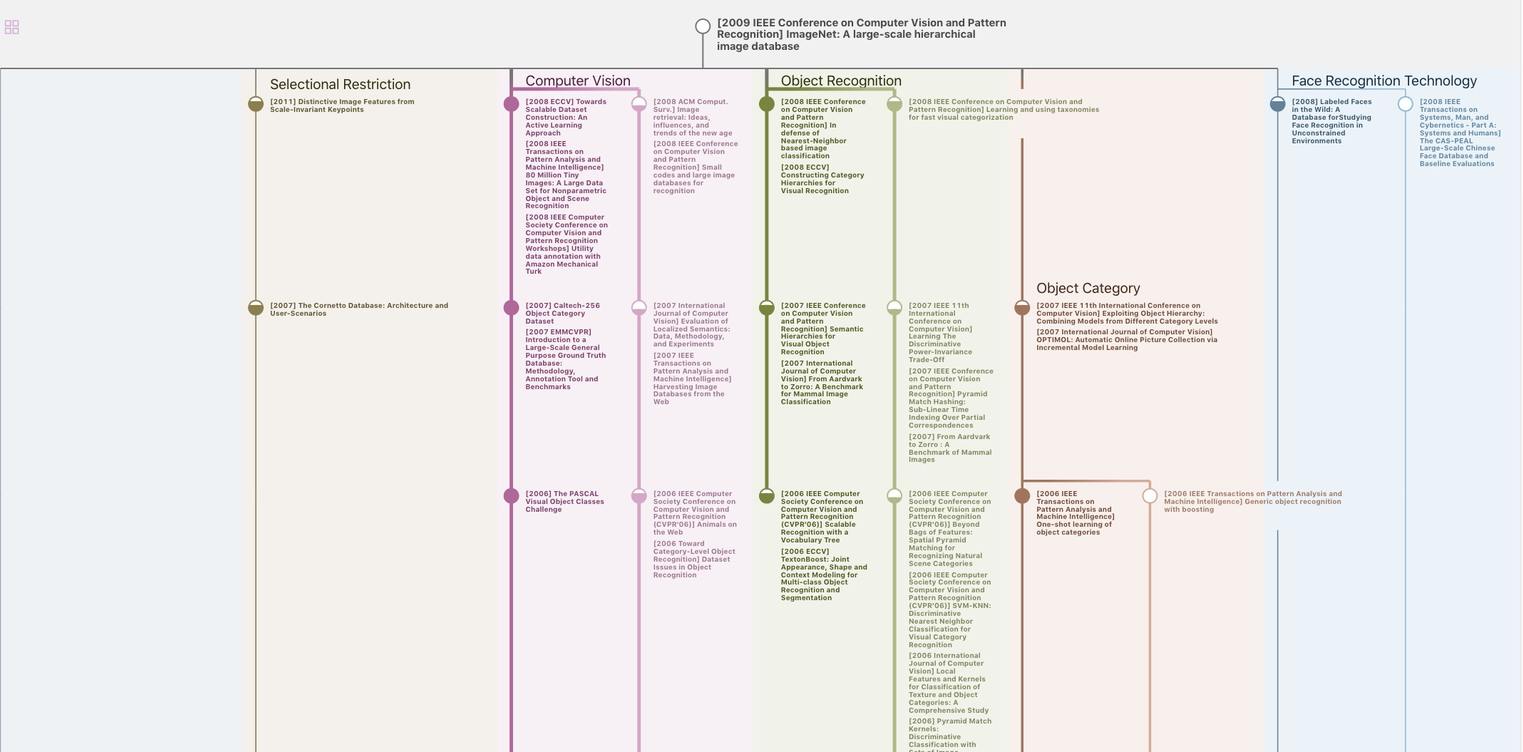
生成溯源树,研究论文发展脉络
Chat Paper
正在生成论文摘要