Learning and Association of Features for Action Recognition in Streaming Video.
ADVANCES IN VISUAL COMPUTING (ISVC 2014), PT II(2014)
摘要
We propose a novel framework which learns and associates local motion pattern manifolds in streaming videos using generalized regression neural networks (GRNN) to facilitate real time human action recognition. The motivation is to determine an individual's action even when the action cycle has not yet been completed. The GRNNs are trained to model the regression function of patterns in latent action space on the input local motion-shape patterns. This manifold learning makes the framework invariant to different sequence length and varying action states. Computation of latent action basis is done using EOF analysis and association of local temporal patterns to an action class at runtime follows a probabilistic formulation. This corresponds to finding the closest estimate the GRNN obtains to the corresponding action basis. Experimental results on two datasets, KTH and the UCF Sports, show accuracy of above 90% obtained from 15 to 25 frames.
更多查看译文
关键词
Empirical Orthogonal Function, Action Recognition, Generalize Regression Neural Network, Empirical Orthogonal Function Analysis, Human Action Recognition
AI 理解论文
溯源树
样例
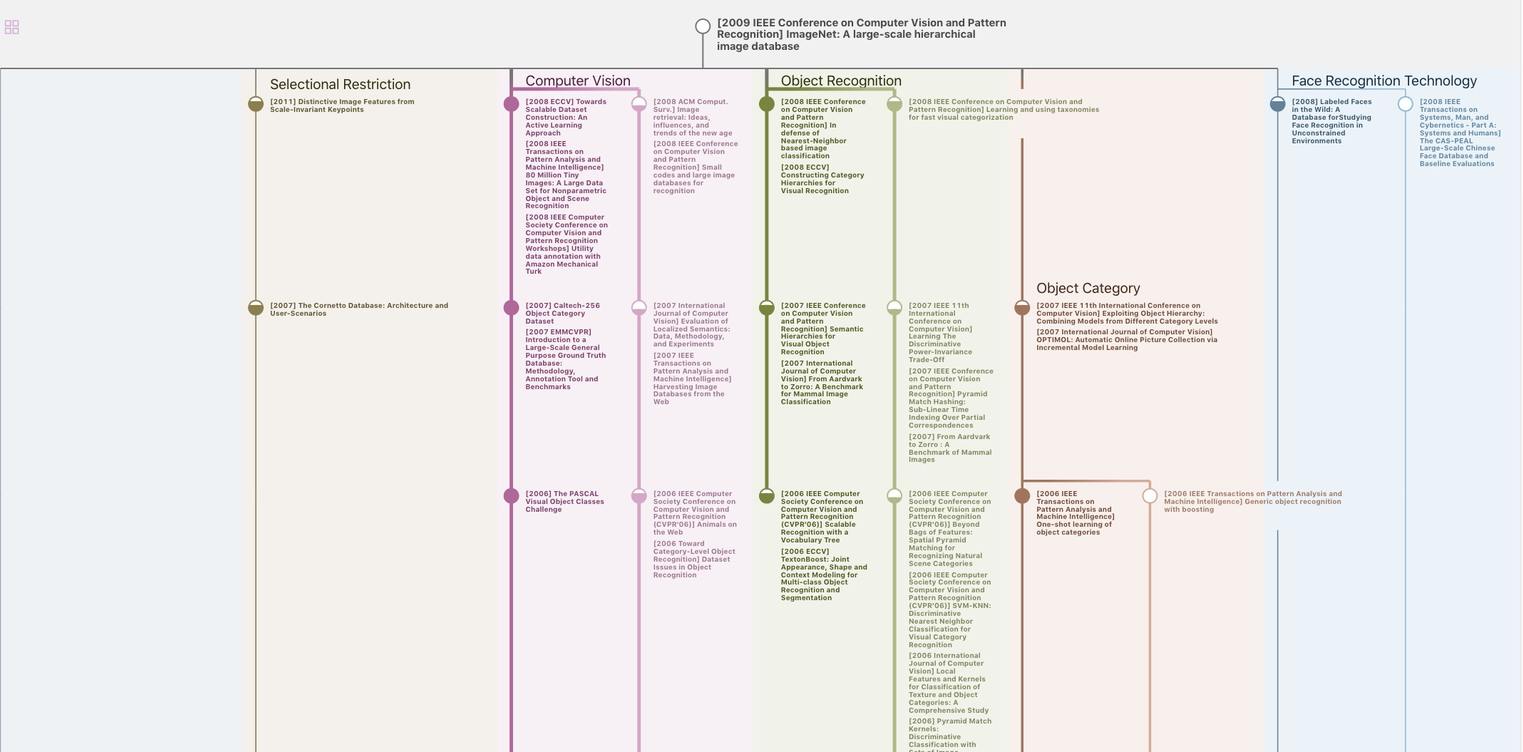
生成溯源树,研究论文发展脉络
Chat Paper
正在生成论文摘要