Motion detection via a couple of auto-encoder networks
ICME(2014)
摘要
Motion detection is a basis step for video processing. Previous works of motion detection based on deep learning need clean foreground or background images which always do not exist in practice. To address this challenge, a novel and practical method is proposed based on auto-encoder neural networks. First, the approximate background images are obtained via an auto-encoder network (called Reconstruction Network) from video frames. Then, a background model is learned based on these images by using another auto-encoder network (called Background Network). To be more resilient, our background model can be updated on-line to absorb more training samples. Our main contributions are 1) the architecture of the couple of auto-encoder networks which can model the background very efficiently; 2) the online learning algorithm in which a method of searching the minimizing effect parameters is adopted to accelerate the training of the Reconstruction Network. Our approach improves the motion detection performance on three data sets.
更多查看译文
关键词
video signal processing,online learning algorithm,approximation theory,learning (artificial intelligence),motion detection,reconstruction network,video processing,deep auto-encoder network,online learning,background model,deep learning,approximate background images,autoencoder neural networks,neural nets,image motion analysis,vectors,learning artificial intelligence,hidden markov models,image reconstruction
AI 理解论文
溯源树
样例
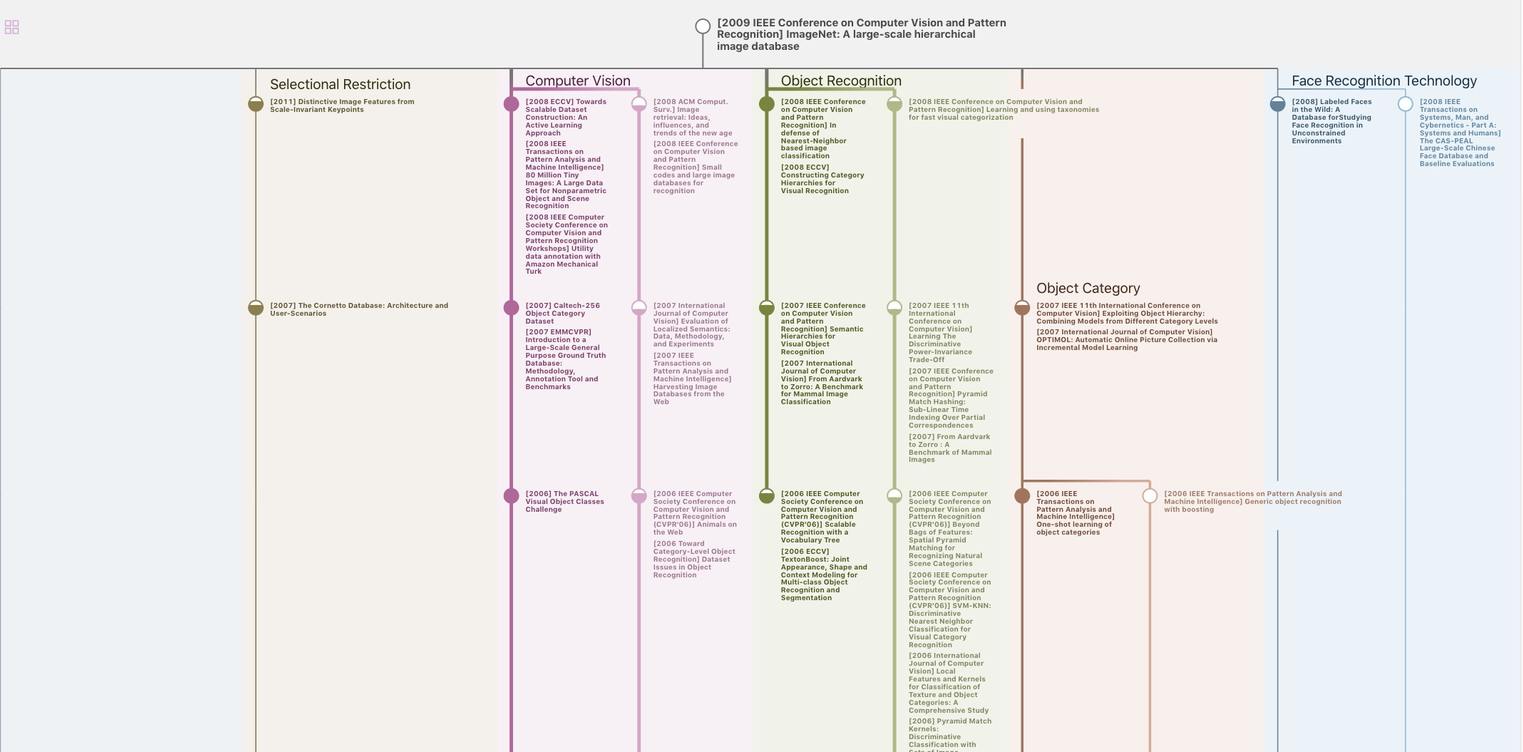
生成溯源树,研究论文发展脉络
Chat Paper
正在生成论文摘要