Scale-invariant corner keypoints
ICIP(2014)
摘要
Effective and efficient generation of keypoints from images is the first step of many computer vision applications, such as object matching. The last decade presented us with an arms race toward faster and more robust keypoint detection, feature description and matching. This resulted in several new algorithms, for example Scale Invariant Features Transform (SIFT), Speed-up Robust Feature (SURF), Oriented FAST and Rotated BRIEF (ORB) and Binary Robust Invariant Scalable Keypoints (BRISK). The keypoint detection has been improved using various techniques in most of these algorithms. However, in the search for faster computing, the accuracy of the algorithms is decreasing. In this paper, we present SICK (Scale-Invariant Corner Keypoints), which is a novel method for fast keypoint detection. Our experiment results show that SICK is faster to compute and more robust than recent state-of-the-art methods.
更多查看译文
关键词
image matching,scale-space,scale-invariant corner keypoints,sick,fast keypoint detection,edge detection,keypoint detection,computer vision,transforms,corner detection,object matching,signal processing,scale space
AI 理解论文
溯源树
样例
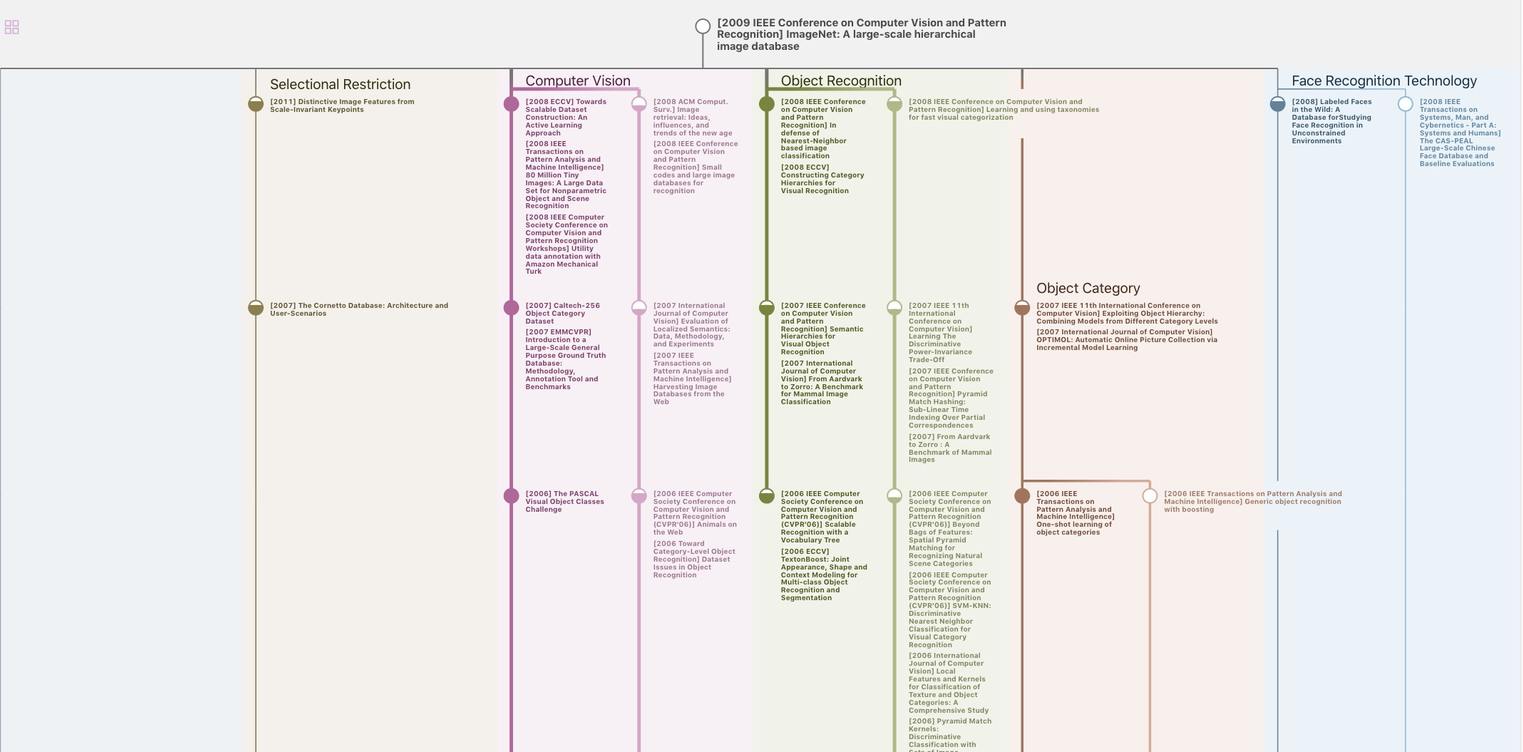
生成溯源树,研究论文发展脉络
Chat Paper
正在生成论文摘要