Online feature subset selection for object tracking
ICIP(2014)
摘要
Online tracking often encounters the drift problem due to factors such as occlusion, motion blur, pose and illumination changes. While much success has been demonstrated, it is still a challenging task to design a robust appearance model for the tracker to effectively solve the drift problem. In this paper, we propose a novel object tracking framework with appearance model based on an effective online feature subset selection scheme which combines a support vector machine recursive feature elimination (SVM-RFE) procedure and a multiple instance learning (MIL) optimization process. The SVM-RFE procedure can help find the most informative subset from a feature pool, while the MIL optimization process helps to solve the ambiguity problem. Experiments on the benchmark dataset and comparisons with the latest state-of-the-art trackers validate the advantage of our approach.
更多查看译文
关键词
optimisation,mil optimization process,learning (artificial intelligence),object tracking,support vector machine recursive feature elimination,appearance model,online feature subset selection,multiple instance learning,svm recursive feature elimination,feature selection,svm-rfe,support vector machines
AI 理解论文
溯源树
样例
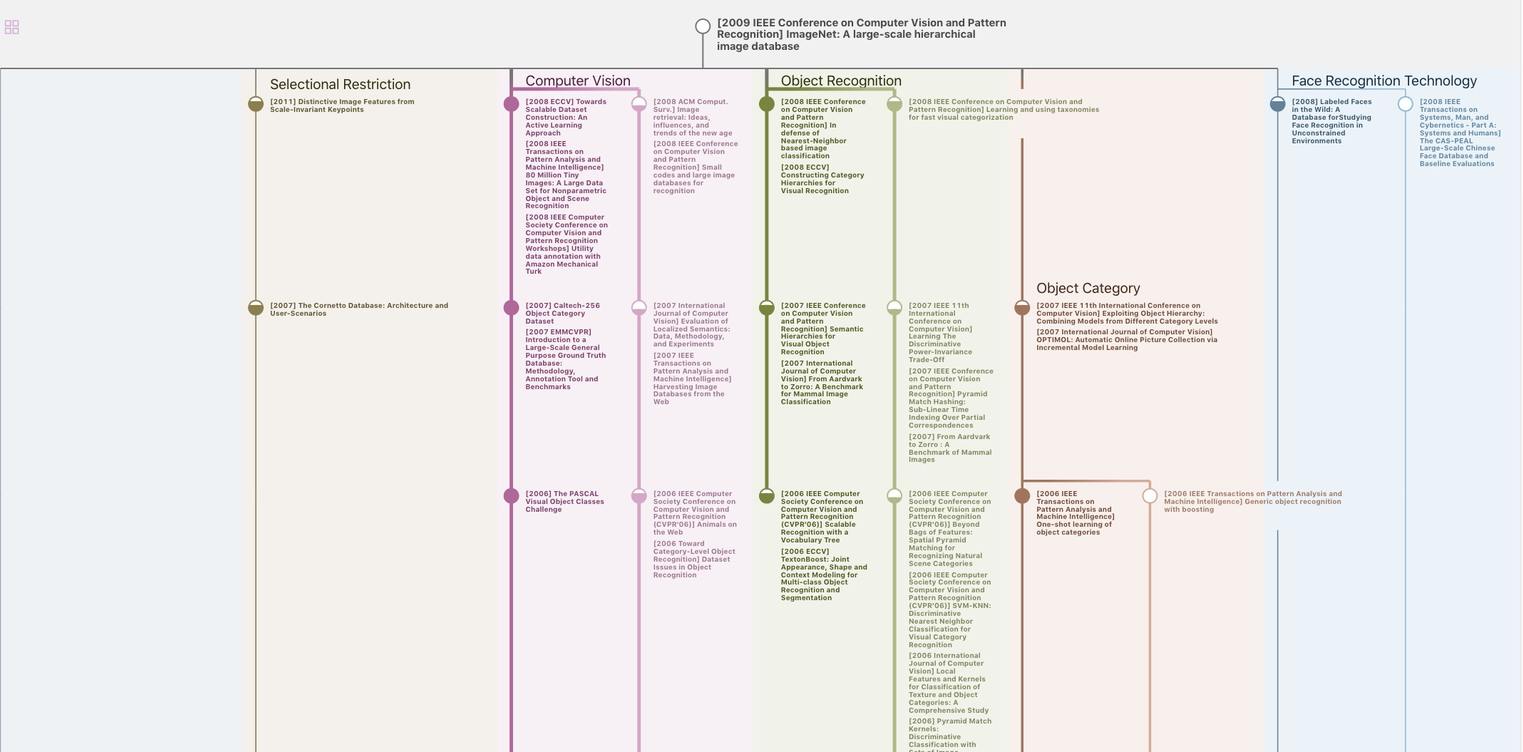
生成溯源树,研究论文发展脉络
Chat Paper
正在生成论文摘要