Image character recognition using deep convolutional neural network learned from different languages
ICIP(2014)
摘要
This paper proposes a shared-hidden-layer deep convolutional neural network (SHL-CNN) for image character recognition. In SHL-CNN, the hidden layers are made common across characters from different languages, performing a universal feature extraction process that aims at learning common character traits existed in different languages such as strokes, while the final softmax layer is made language dependent, trained based on characters from the destination language only. This paper is the first attempt to introduce the SHL-CNN framework to image character recognition. Under the SHL-CNN framework, we discuss several issues including architecture of the network, training of the network, from which a suitable SHL-CNN model for image character recognition is empirically learned. The effectiveness of the learned SHL-CNN is verified on both English and Chinese image character recognition tasks, showing that the SHL-CNN can reduce recognition errors by 16-30% relatively compared with models trained by characters of only one language using conventional CNN, and by 35.7% relatively compared with state-of-the-art methods. In addition, the shared hidden layers learned are also useful for unseen image character recognition tasks.
更多查看译文
关键词
english image character recognition,deep convolutional neural network,shl-cnn model,destination language,shared-hidden-layer deep convolutional neural network,learning (artificial intelligence),unseen image character recognition,character recognition,chinese image character recognition,image recognition,image character recognition,multi-task learning,hidden layers,feature extraction,shl-cnn framework,softmax layer,natural language processing,neural nets,universal feature extraction process,character traits,multi task learning
AI 理解论文
溯源树
样例
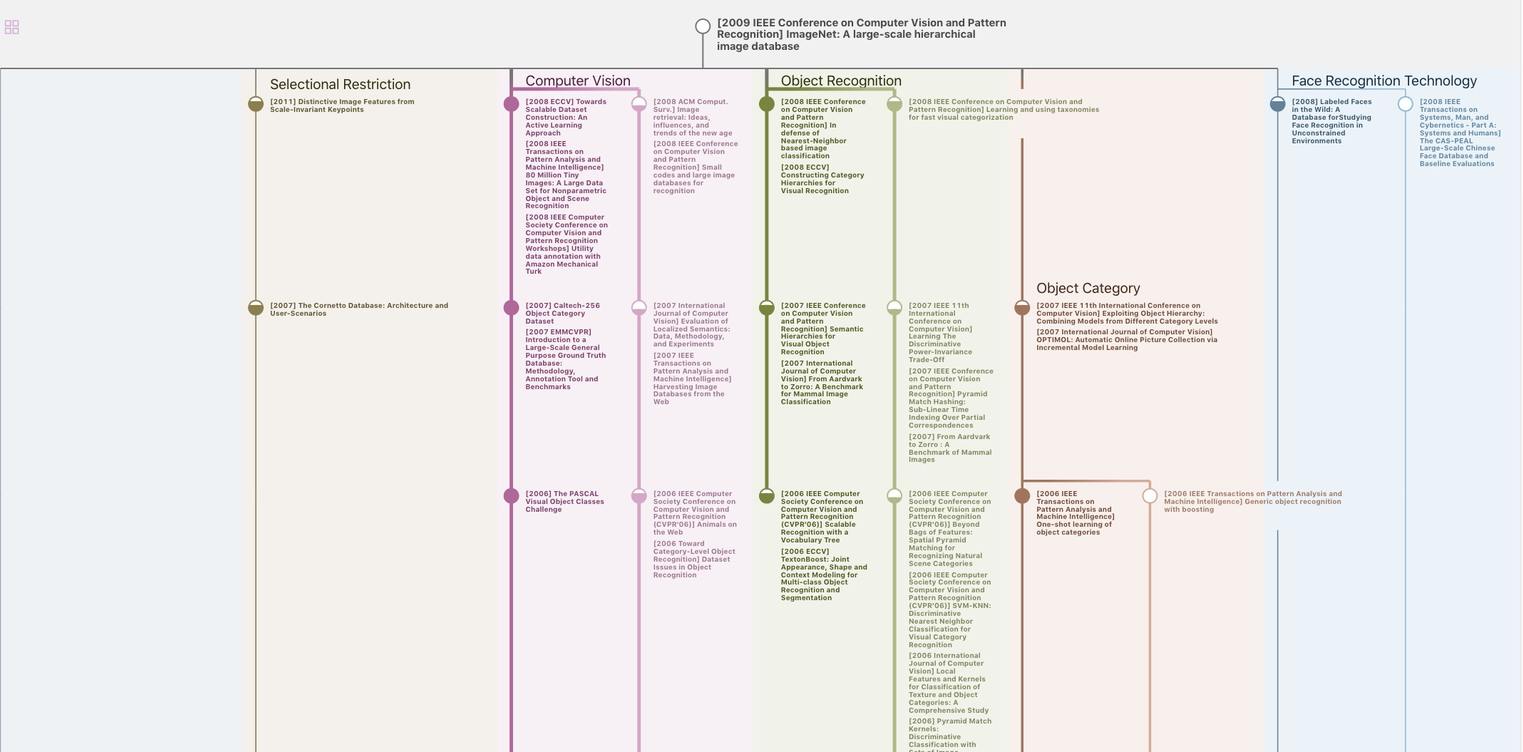
生成溯源树,研究论文发展脉络
Chat Paper
正在生成论文摘要