An Extended Isomap for Manifold Topology Learning with SOINN Landmarks
ICPR(2014)
摘要
This paper presents an extended Isomap algorithm called SL-Isomap (SOINN Landmark Isomap). We adopt SOINN (Self-Organizing Incremental Neural Network) algorithm to choose the reasonable number of landmarks automatically. SOINN landmarks are able to represent topological structure of unsupervised data in the high dimensional input space. Then L-Isomap (Landmark Isomap) algorithm is used to find low dimensional manifolds from high dimensional data based on chosen landmarks. SL-Isomap solves the problem of selecting the right number and position of landmarks automatically thus reduces short-circuit errors. It also realizes data compression and nonlinear dimensionality reduction at the same time. Experiments demonstrate its promising results compared with other variants of L-Isomap.
更多查看译文
关键词
extended isomap algorithm,unsupervised data,manifold topology learning,self-organizing incremental neural network algorithm,nonlinear dimensionality reduction,sl-isomap,data compression,l-isomap algorithm,topological structure representation,topology,soinn landmark isomap,high dimensional data,short-circuit errors,self-organising feature maps,unsupervised learning
AI 理解论文
溯源树
样例
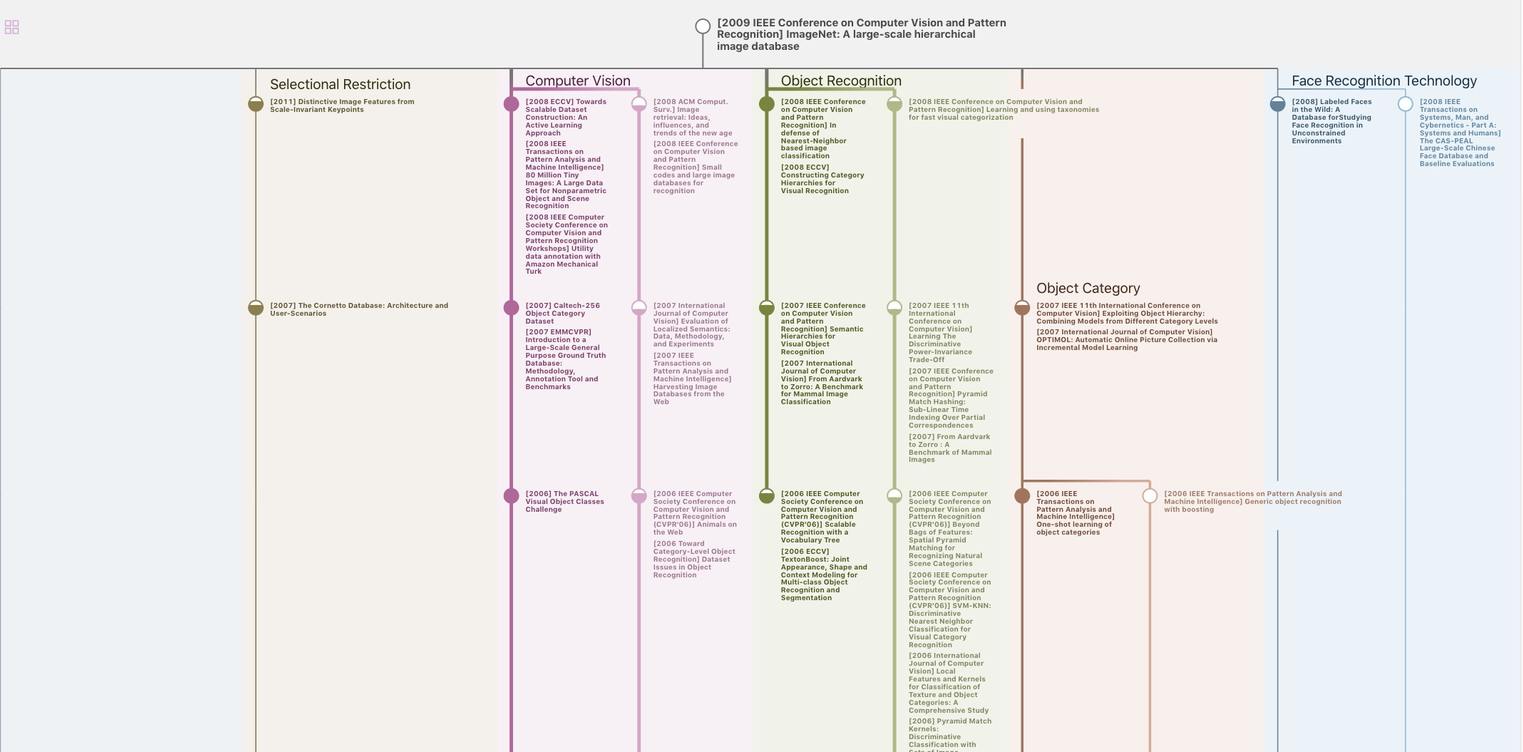
生成溯源树,研究论文发展脉络
Chat Paper
正在生成论文摘要