Reconciling malware labeling discrepancy via consensus learning
ICDE Workshops(2014)
摘要
Anti-virus systems developed by different vendors often demonstrate strong discrepancy in the labels they assign to given malware, which significantly hinders threat intelligence sharing. The key challenge of addressing this discrepancy stems from the difficulty of re-standardizing already-in-use systems. In this paper we explore a non-intrusive alternative. We propose to leverage the correlation between the malware labels of different anti-virus systems to create a “consensus” classification system, through which different systems can share information without modifying their own labeling conventions. To this end, we present a novel classification integration framework Latin which exploits the correspondence between participating anti-virus systems as reflected in heterogeneous information at instance-instance, instance-class, and class-class levels. We provide results from extensive experimental studies using real datasets and concrete use cases to verify the efficacy of Latin in reconciling the malware labeling discrepancy.
更多查看译文
关键词
computer viruses,consensus learning,anti-virus systems,learning (artificial intelligence),pattern classification,instance-class levels,latin classification integration framework,threat intelligence sharing,consensus classification system,malware labeling discrepancy,class-class levels,instance-instance levels,heterogeneous information
AI 理解论文
溯源树
样例
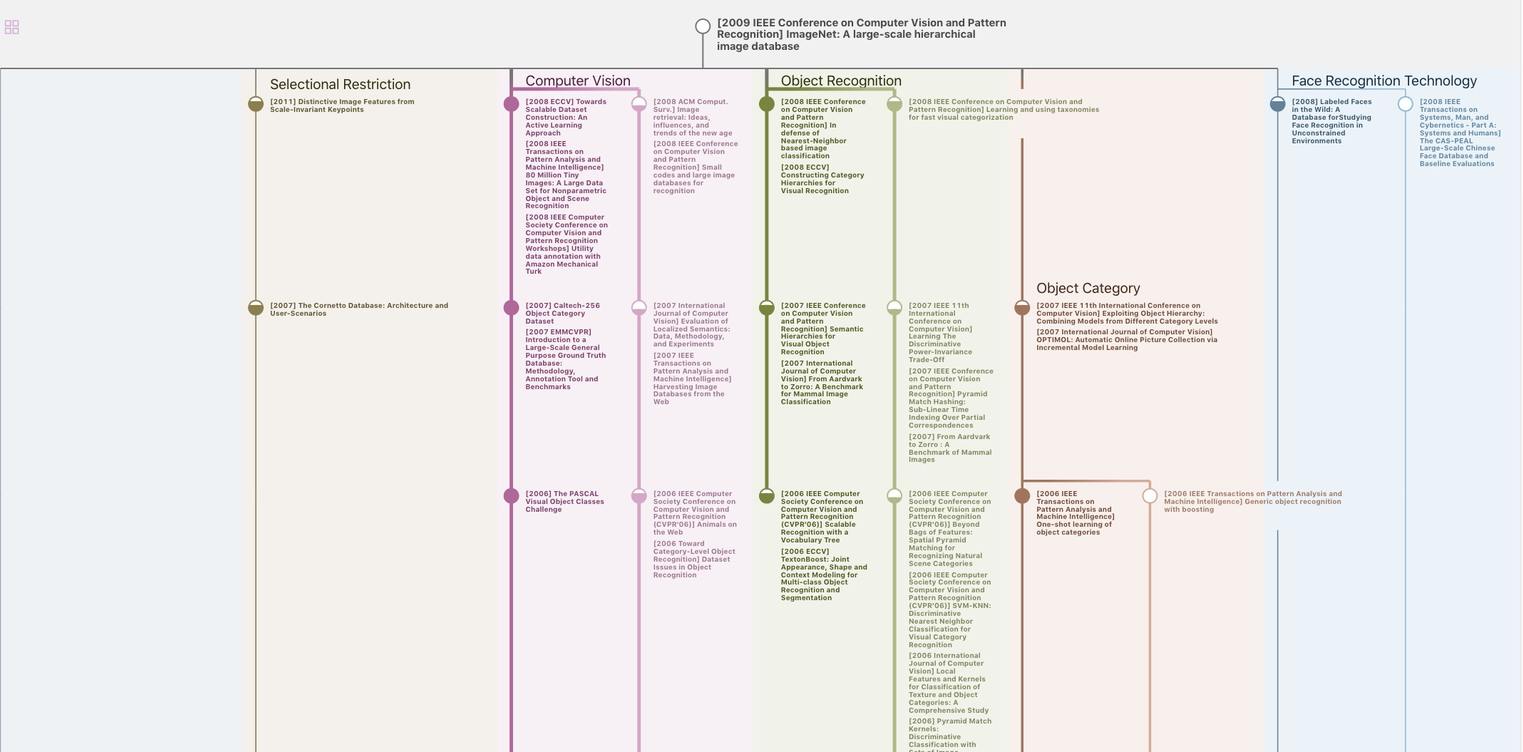
生成溯源树,研究论文发展脉络
Chat Paper
正在生成论文摘要