Mining temporal lag from fluctuating events for correlation and root cause analysis
CNSM(2014)
摘要
The importance of mining time lags of hidden temporal dependencies from sequential data is highlighted in many domains including system management, stock market analysis, climate monitoring, and more. Mining time lags of temporal dependencies provides useful insights into understanding the sequential data and predicting its evolving trend. Traditional methods mainly utilize the predefined time window to analyze the sequential items or employ statistic techniques to identify the temporal dependencies from the sequential data. However, it is a challenging task for existing methods to find time lag of temporal dependencies in the real world, where time lags are fluctuating, noisy, and tend to be interleaved with each other. This paper introduces a parametric model to describe noisy time lags. Then an efficient expectation maximization approach is proposed to find the time lag with maximum likelihood. This paper also contributes an approximation method for learning time lag to improve the scalability without incurring significant loss of accuracy. Extensive experiments on both synthetic and real data sets are conducted to demonstrate the effectiveness and efficiency of proposed methods.
更多查看译文
关键词
noisy time lags,stock market analysis,expectation-maximisation algorithm,real data sets,approximation theory,system management,parametric model,expectation maximization approach,delays,time lag mining,root cause analysis,hidden temporal dependencies,maximum likelihood,data mining,climate monitoring,approximation method,synthetic data sets,time lag learning
AI 理解论文
溯源树
样例
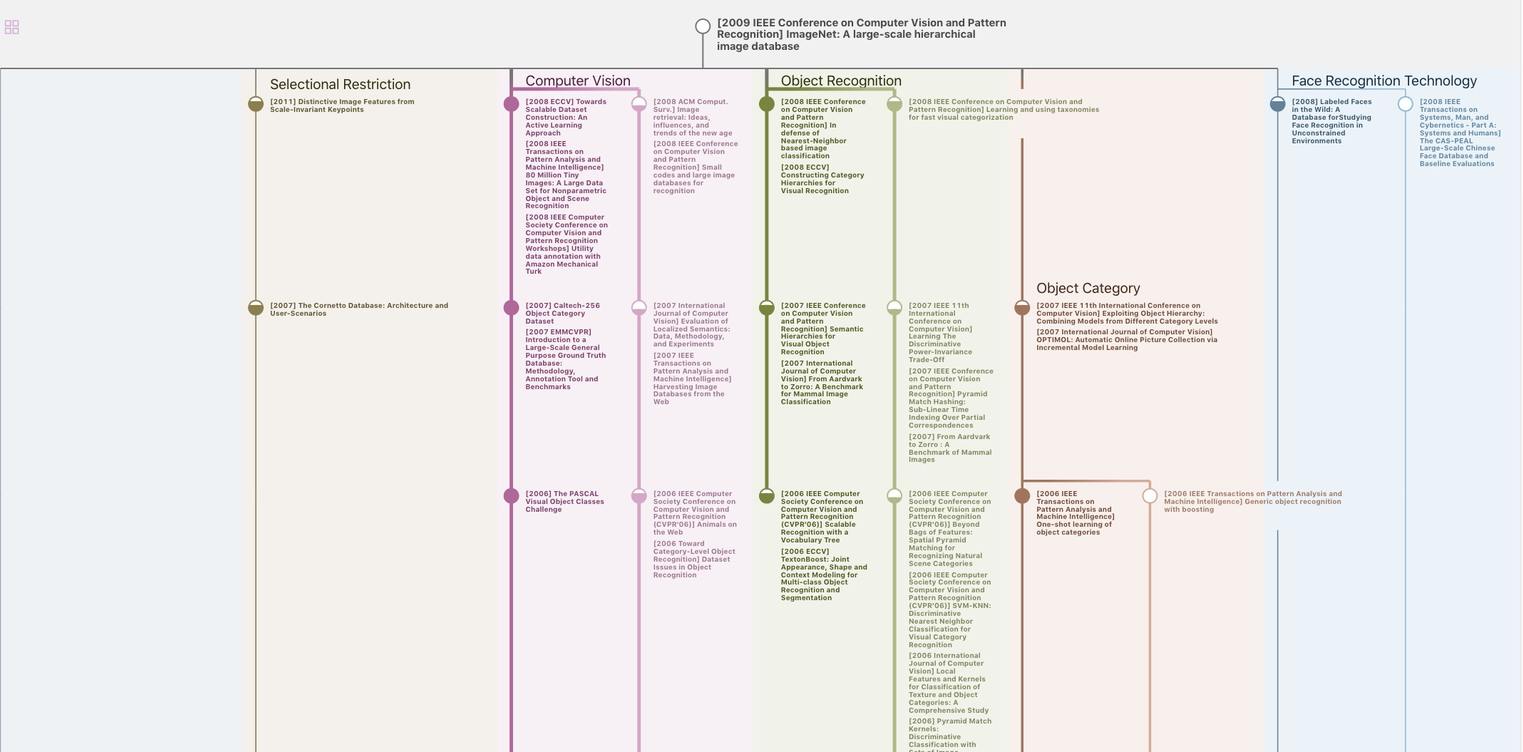
生成溯源树,研究论文发展脉络
Chat Paper
正在生成论文摘要