Listwise Approach For Rank Aggregation In Crowdsourcing
WSDM 2015: Eighth ACM International Conference on Web Search and Data Mining Shanghai China February, 2015(2015)
摘要
Inferring a gold-standard ranking over a set of objects, such as documents or images, is a key task to build test collections for various applications like Web search and recommender systems. Crowdsourcing services provide an efficient and inexpensive way to collect judgments via labeling by sets of annotators. We thus study the problem of finding a consensus ranking from crowdsourced judgments. In contrast to conventional rank aggregation methods which minimize the distance between predicted ranking and input judgments from either pointwise or pairwise perspective, we argue that it is critical to consider the distance in a listwise way to emphasize the position importance in ranking. Therefore, we introduce a new listwise approach in this paper, where ranking measure based objective functions are utilized for optimization. In addition, we also incorporate the annotator quality into our model since the reliability of annotators can vary significantly in crowdsourcing. For optimization, we transform the optimization problem to the Linear Sum Assignment Problem, and then solve it by a very efficient algorithm named CrowdAgg guaranteeing the optimal solution. Experimental results on two benchmark data sets from different crowdsourcing tasks show that our algorithm is much more effective, efficient and robust than traditional methods.
更多查看译文
关键词
Crowdsourced Labeling,Rank Aggregation,Evaluation Measures
AI 理解论文
溯源树
样例
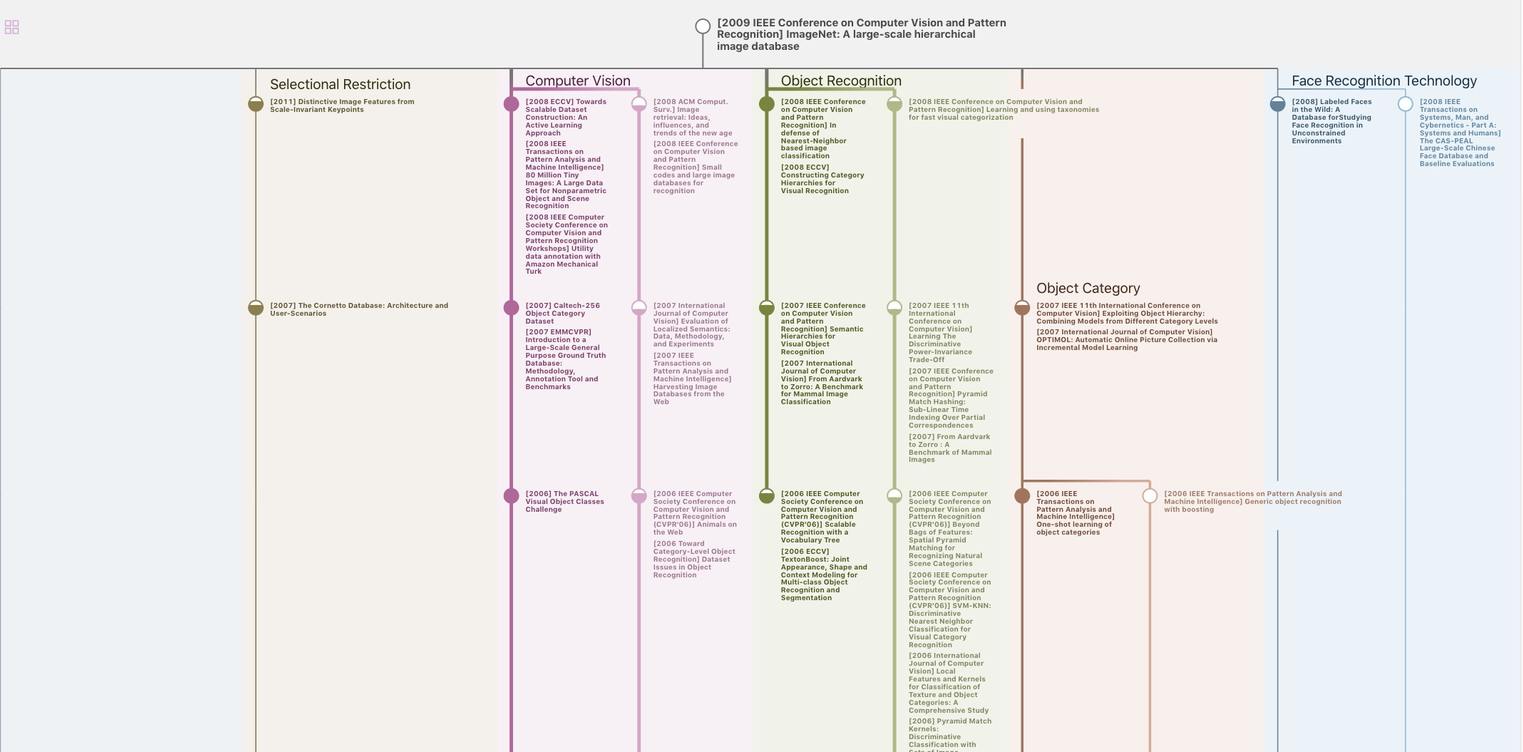
生成溯源树,研究论文发展脉络
Chat Paper
正在生成论文摘要