Reducing Multi-Hop Calibration Errors In Large-Scale Mobile Sensor Networks
IPSN '15: The 14th International Conference on Information Processing in Sensor Networks Seattle Washington April, 2015(2015)
摘要
Frequent sensor calibration is essential in sensor networks with low-cost sensors. We exploit the fact that temporally and spatially close measurements of different sensors measuring the same phenomenon are similar. Hence, when calibrating a sensor, we adjust its calibration parameters to minimize the differences between co-located measurements of previously calibrated sensors. In turn, freshly calibrated sensors can now be used to calibrate other sensors in the network, referred to as multi-hop calibration. We are the first to study multi-hop calibration with respect to a reference signal (micro-calibration) in detail. We show that ordinary least squares regression-commonly used to calibrate noisy sensors-suffers from significant error accumulation over multiple hops. In this paper, we propose a novel multi-hop calibration algorithm using geometric mean regression, which (i) highly reduces error propagation in the network, (ii) distinctly outperforms ordinary least squares in the multi-hop scenario, and (iii) requires considerably fewer ground truth measurements compared to existing network calibration algorithms. The proposed algorithm is especially valuable when calibrating large networks of heterogeneous sensors with different noise characteristics. We provide theoretical justifications for our claims. Then, we conduct a detailed analysis with artificial data to study calibration accuracy under various settings and to identify different error sources. Finally, we use our algorithm to accurately calibrate 13 million temperature, ground ozone (O-3), and carbon monoxide (CO) measurements gathered by our mobile air pollution monitoring network.
更多查看译文
AI 理解论文
溯源树
样例
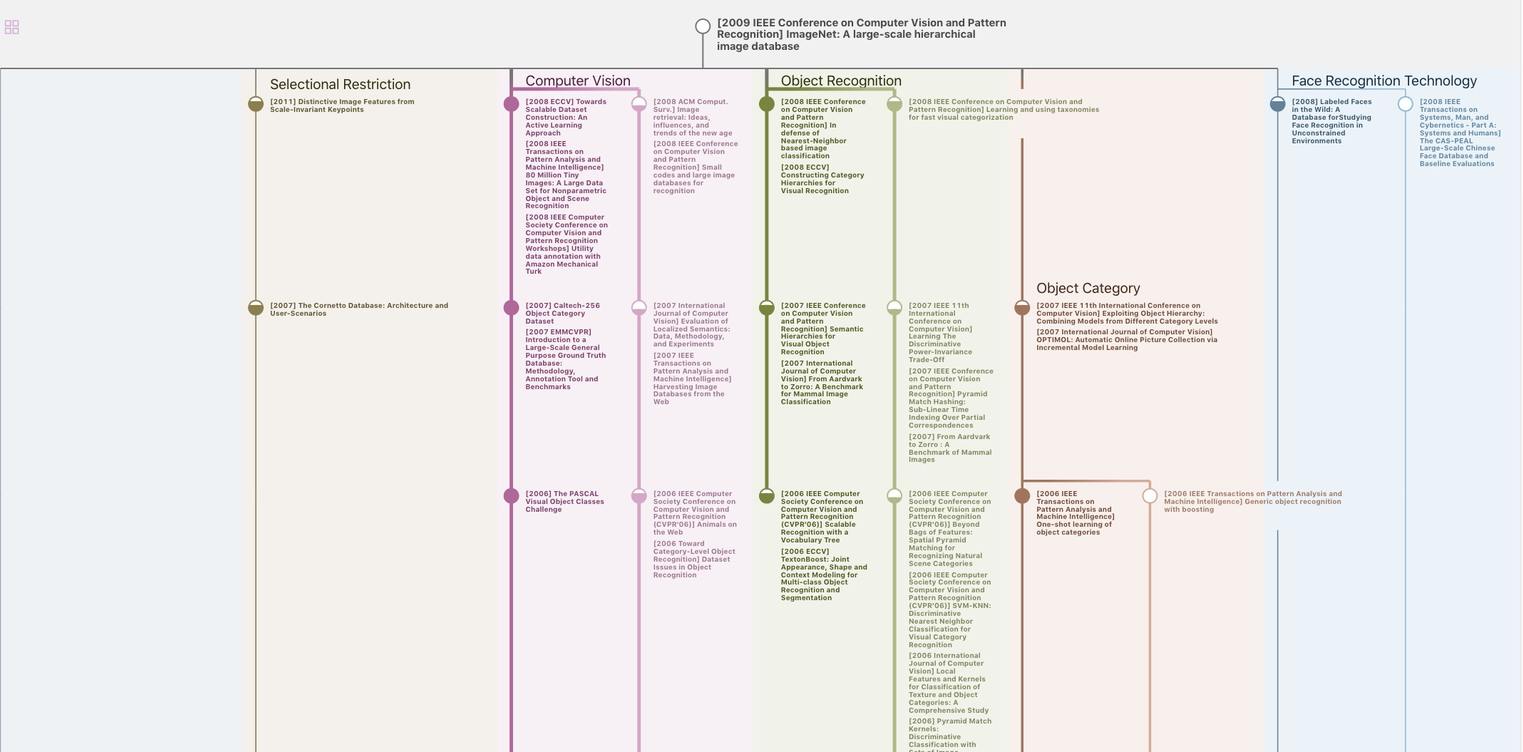
生成溯源树,研究论文发展脉络
Chat Paper
正在生成论文摘要