Learning person-person interaction in collective activity recognition.
IEEE Transactions on Image Processing(2015)
摘要
Collective activity is a collection of atomic activities (individual person's activity) and can hardly be distinguished by an atomic activity in isolation. The interactions among people are important cues for recognizing collective activity. In this paper, we concentrate on modeling the person-person interactions for collective activity recognition. Rather than relying on hand-craft description of the person-person interaction, we propose a novel learning-based approach that is capable of computing the class-specific person-person interaction patterns. In particular, we model each class of collective activity by an interaction matrix, which is designed to measure the connection between any pair of atomic activities in a collective activity instance. We then formulate an interaction response (IR) model by assembling all these measurements and make the IR class specific and distinct from each other. A multitask IR is further proposed to jointly learn different person-person interaction patterns simultaneously in order to learn the relation between different person-person interactions and keep more distinct activity-specific factor for each interaction at the same time. Our model is able to exploit discriminative low-rank representation of person-person interaction. Experimental results on two challenging data sets demonstrate our proposed model is comparable with the state-of-the-art models and show that learning person-person interactions plays a critical role in collective activity recognition.
更多查看译文
关键词
learning-based approach,class-specific person-person interaction patterns,image representation,learning (artificial intelligence),interaction matrix,matrix algebra,action analysis,interaction response model,ir model,object recognition,activity-specific factor,discriminative low-rank representation,collective activity recognition,interaction modeling,person-person interaction,atomic activity collection,feature extraction,graphical models,computational modeling,learning artificial intelligence,optimization
AI 理解论文
溯源树
样例
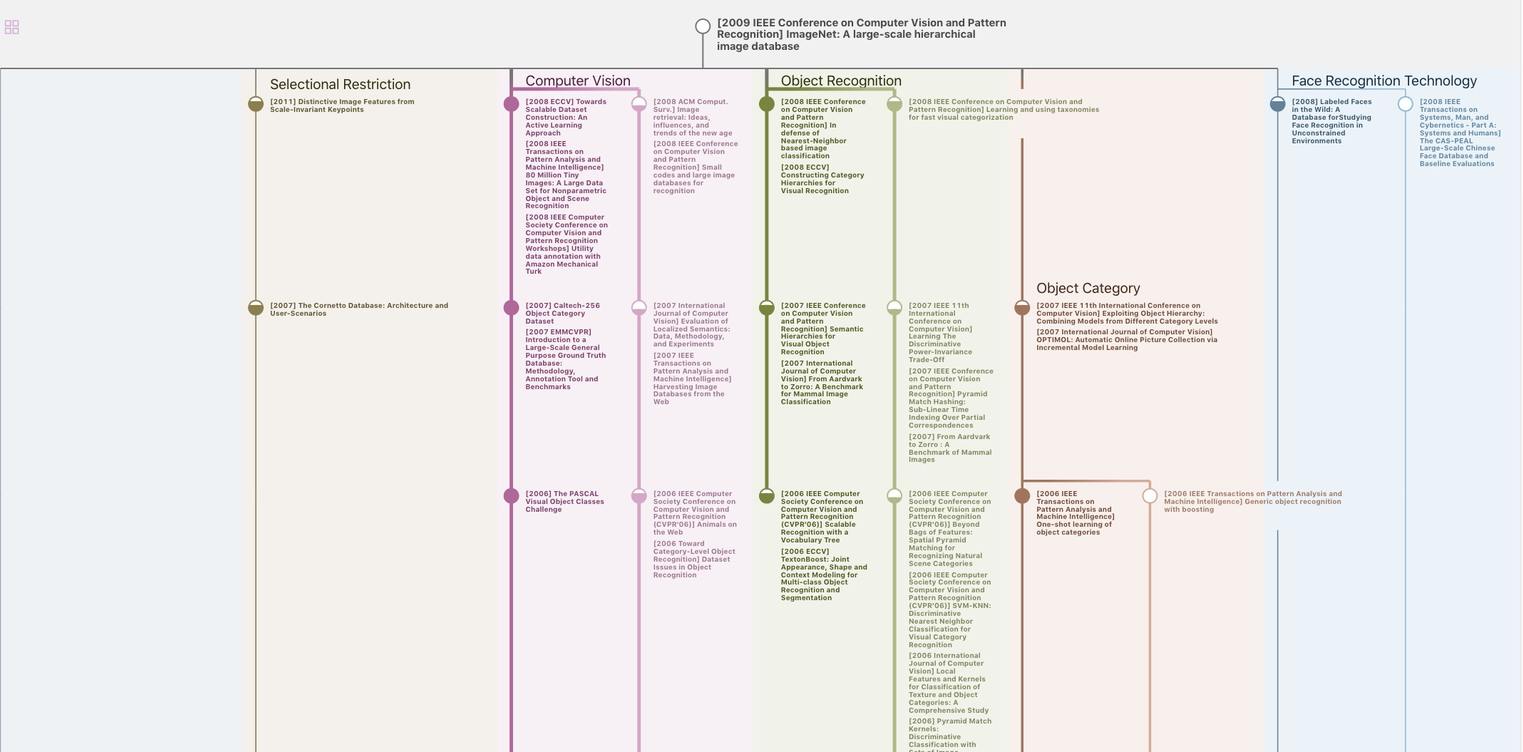
生成溯源树,研究论文发展脉络
Chat Paper
正在生成论文摘要