Minimizing data consumption with sequential online feature selection
International Journal of Machine Learning and Cybernetics(2012)
摘要
In most real-world information processing problems, data is not a free resource. Its acquisition is often expensive and time-consuming. We investigate how such cost factors can be included in supervised classification tasks by deriving classification as a sequential decision process and making it accessible to reinforcement learning. Depending on previously selected features and the internal belief of the classifier, a next feature is chosen by a sequential online feature selection that learns which features are most informative at each time step. Experiments on toy datasets and a handwritten digits classification task show significant reduction in required data for correct classification, while a medical diabetes prediction task illustrates variable feature cost minimization as a further property of our algorithm.
更多查看译文
关键词
Reinforcement learning,Feature selection,Classification
AI 理解论文
溯源树
样例
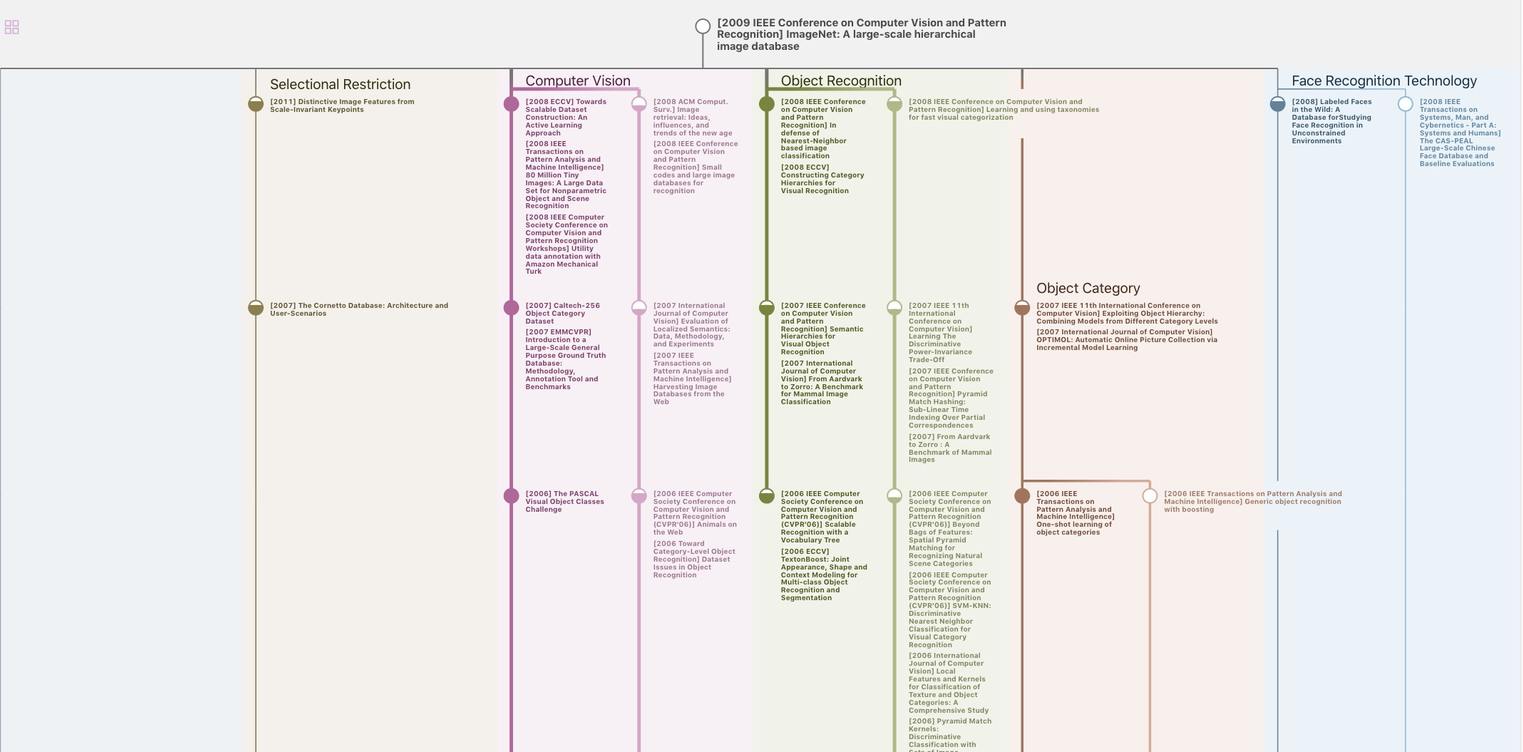
生成溯源树,研究论文发展脉络
Chat Paper
正在生成论文摘要