Conditioning and covariance on caterpillars.
Information Theory Workshop(2015)
摘要
Let X 1 , …, X n be joint {±1}-valued random variables. It is known that conditioning on a random subset of O(1/ϵ 2 ) of them reduces their average pairwise covariance to below ϵ (in expectation). We conjecture that O(1/ϵ 2 ) can be improved to O(1/ϵ). The motivation for the problem and our conjectured improvement comes from the theory of global correlation rounding for convex relaxation hierarchies. We suggest attempting the conjecture in the case that X 1 , …, X n are the leaves of an information flow tree. We prove the conjecture in the case that the information flow tree is a caterpillar graph (similar to a two-state hidden Markov model).
更多查看译文
关键词
covariance analysis,hidden Markov models,random processes,set theory,trees (mathematics),average pairwise covariance,caterpillar graph,conditioning,convex relaxation hierarchies,global correlation,information flow tree leaves,joint {±1}-valued random variables,random subset,two-state hidden Markov model
AI 理解论文
溯源树
样例
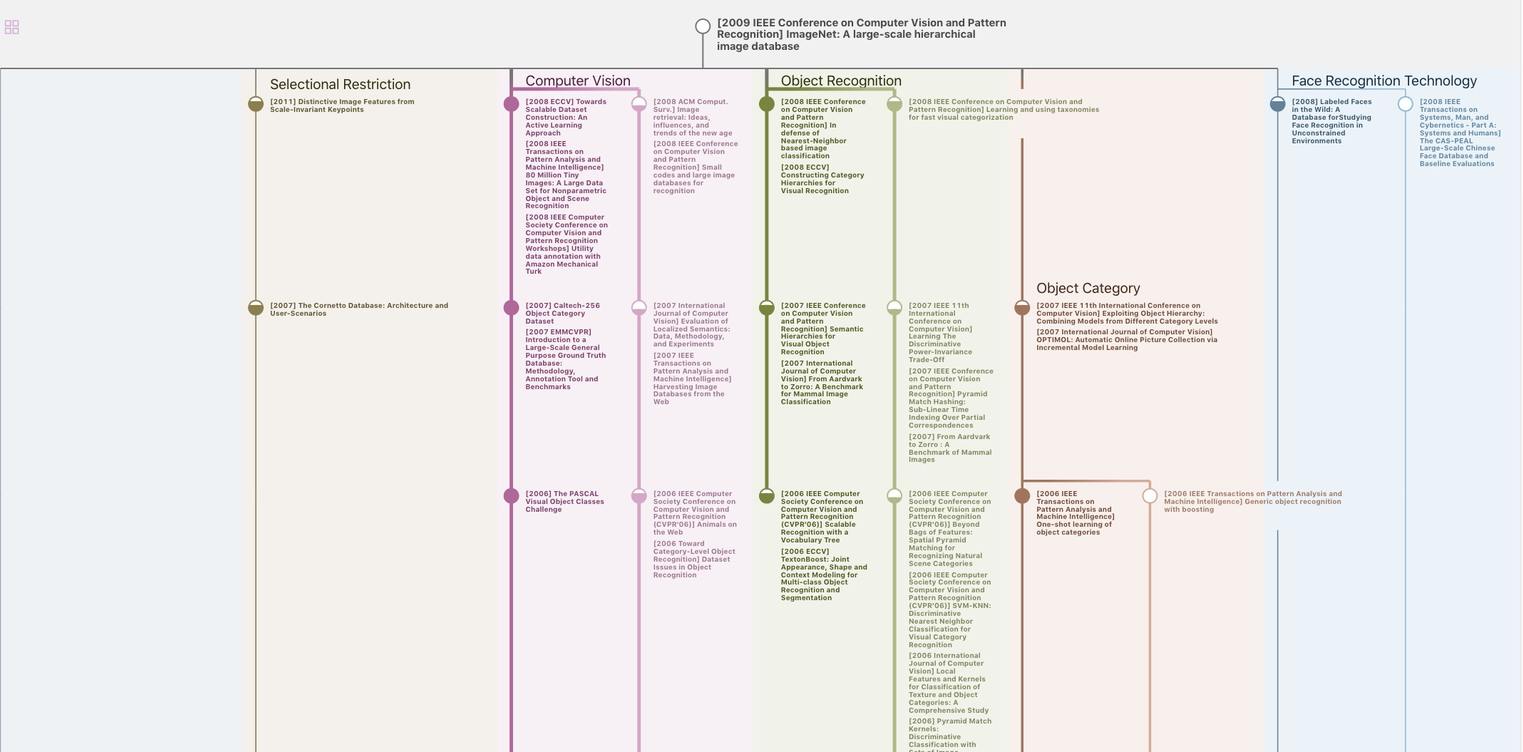
生成溯源树,研究论文发展脉络
Chat Paper
正在生成论文摘要