Understanding Image Representations by Measuring Their Equivariance and Equivalence
computer vision and pattern recognition(2018)
摘要
Despite the importance of image representations such as histograms of oriented gradients and deep Convolutional Neural Networks (CNN), our theoretical understanding of them remains limited. Aimed at filling this gap, we investigate two key mathematical properties of representations: equivariance and equivalence. Equivariance studies how transformations of the input image are encoded by the representation, invariance being a special case where a transformation has no effect. Equivalence studies whether two representations, for example two different parameterizations of a CNN, two different layers, or two different CNN architectures, share the same visual information or not. A number of methods to establish these properties empirically are proposed, including introducing transformation and stitching layers in CNNs. These methods are then applied to popular representations to reveal insightful aspects of their structure, including clarifying at which layers in a CNN certain geometric invariances are achieved and how various CNN architectures differ. We identify several predictors of geometric and architectural compatibility, including the spatial resolution of the representation and the complexity and depth of the models. While the focus of the paper is theoretical, direct applications to structured-output regression are demonstrated too.
更多查看译文
关键词
Image representations,Geometric equivariance,Equivalent representations,Convolutional neural networks
AI 理解论文
溯源树
样例
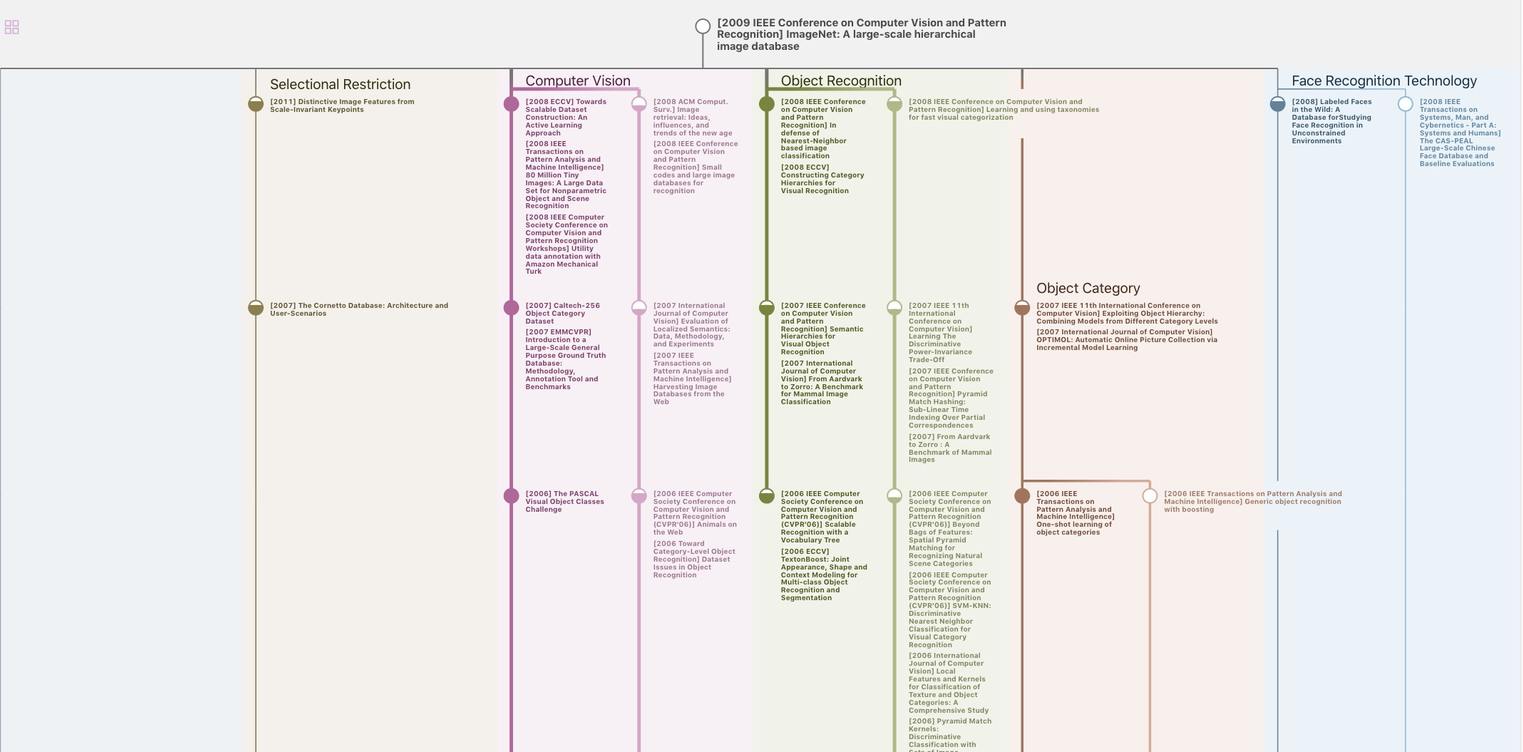
生成溯源树,研究论文发展脉络
Chat Paper
正在生成论文摘要