Improved Practical Matrix Sketching With Guarantees
IEEE Transactions on Knowledge and Data Engineering(2014)
摘要
Matrices have become essential data representations for many large-scale problems in data analytics, and hence matrix sketching is a critical task. Although much research has focused on improving the error/size tradeoff under various sketching paradigms, we find a simple heuristic iSVD, with no guarantees, tends to outperform all known approaches. In this paper we adapt the best performing guaranteed algorithm, FrequentDirections, in a way that preserves the guarantees, and nearly matches iSVD in practice. We also demonstrate an adversarial dataset for which iSVD performs quite poorly, but our new technique has almost no error. Finally, we provide easy replication of our studies on APT, a new testbed which makes available not only code and datasets, but also a computing platform with fixed environmental settings.
更多查看译文
关键词
Covariance matrices,Measurement uncertainty,Matrix decomposition,Approximation algorithms,Runtime,Data analysis,Singular value decomposition
AI 理解论文
溯源树
样例
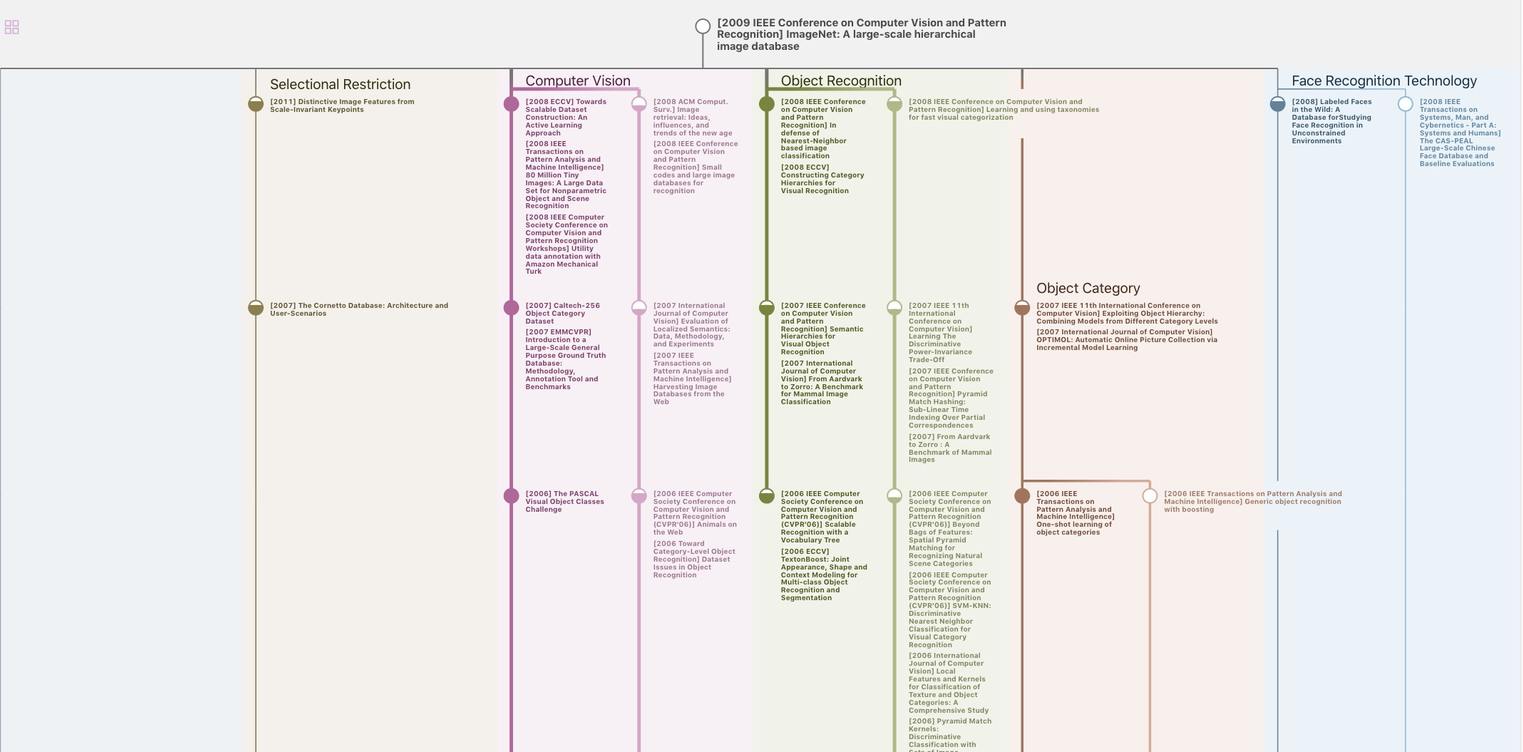
生成溯源树,研究论文发展脉络
Chat Paper
正在生成论文摘要