Multiview self-learning
Neurocomputing(2015)
摘要
In many applications, observations are available with different views. This is, for example, the case with image-text classification, multilingual document classification or document classification on the web. In addition, unlabeled multiview examples can be easily acquired, but assigning labels to these examples is usually a time consuming task. We describe a multiview self-learning strategy which trains different voting classifiers on different views. The margin distributions over the unlabeled training data, obtained with each view-specific classifier are then used to estimate an upper-bound on their transductive Bayes error. Minimizing this upper-bound provides an automatic margin-threshold which is used to assign pseudo-labels to unlabeled examples. Final class labels are then assigned to these examples, by taking a vote on the pool of the previous pseudo-labels. New view-specific classifiers are then trained using the labeled and pseudo-labeled training data. We consider applications to image-text classification and to multilingual document classification. We present experimental results on the NUS-WIDE collection and on Reuters RCV1-RCV2 which show that despite its simplicity, our approach is competitive with other state-of-the-art techniques.
更多查看译文
关键词
image annotation
AI 理解论文
溯源树
样例
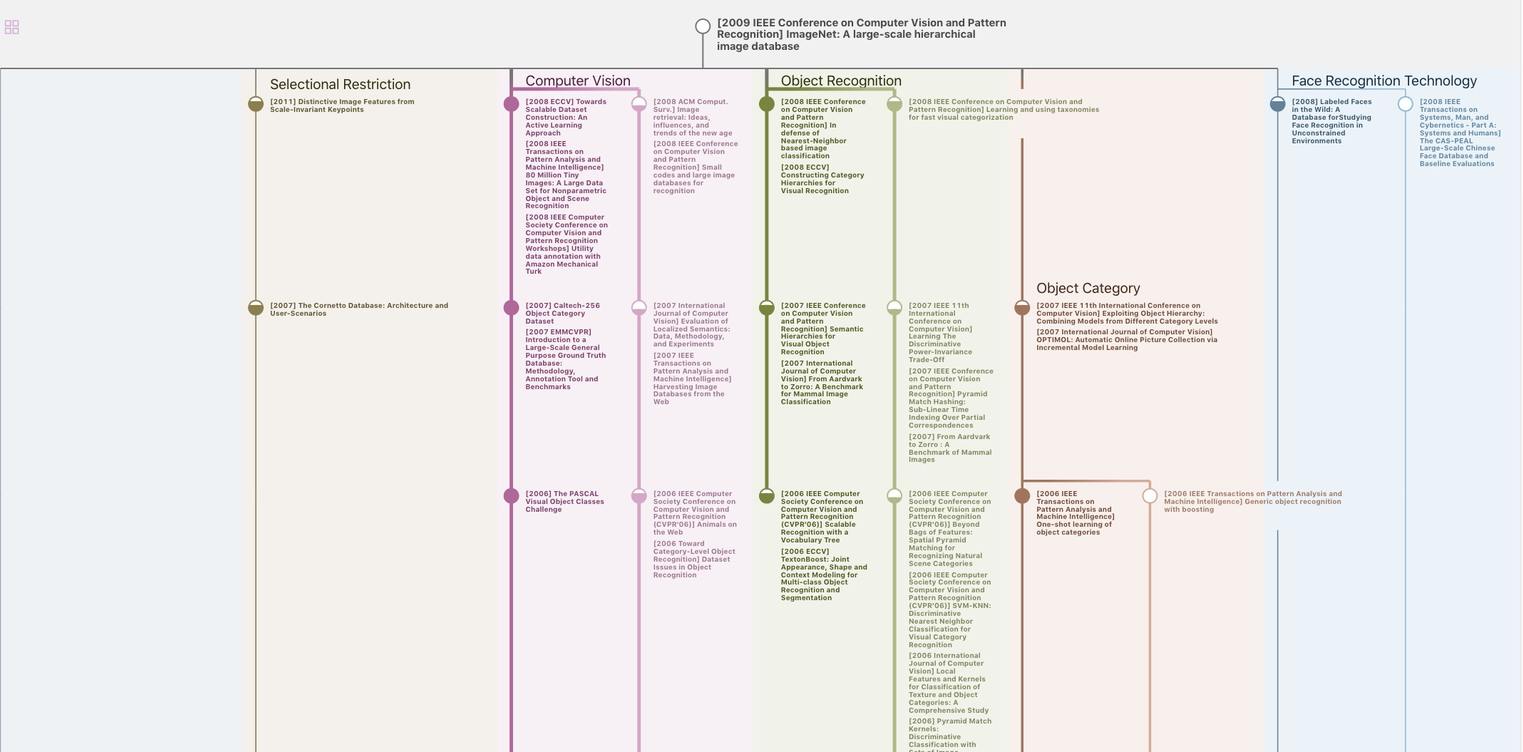
生成溯源树,研究论文发展脉络
Chat Paper
正在生成论文摘要