Leveraging the Error Resilience of Neural Networks for Designing Highly Energy Efficient Accelerators
IEEE Trans. on CAD of Integrated Circuits and Systems(2015)
摘要
In the recent years, inexact computing has been increasingly regarded as one of the most promising approaches for slashing energy consumption in many applications that can tolerate a certain degree of inaccuracy. Driven by the principle of trading tolerable amounts of application accuracy in return for significant resource savings–the energy consumed, the (critical path) delay and the (silicon) area–this approach has been limited to ASICs so far. These ASIC realizations have a narrow application scope and are often rigid in their tolerance to inaccuracy, as currently designed; the latter often determining the extent of resource savings we would achieve. In this paper, we propose to improve the application scope, error resilience and the energy savings of inexact computing by combining it with hardware neural networks. These neural networks are fast emerging as popular candidate accelerators for future heterogeneous multi-core platforms and have flexible error resilience limits owing to their ability to be trained. Our results in 65nm technology demonstrate that the proposed inexact neural network accelerator could achieve 1.78x-2.67x savings in energy consumption (with corresponding delay and area savings being 1.23x and 1.46x respectively) when compared to the existing baseline neural network implementation, at the cost of a small accuracy loss (MSE increases from 0.14 to 0.20 on average).
更多查看译文
关键词
accelerator architectures,energy efficient,hardware neuron network,inexact computing
AI 理解论文
溯源树
样例
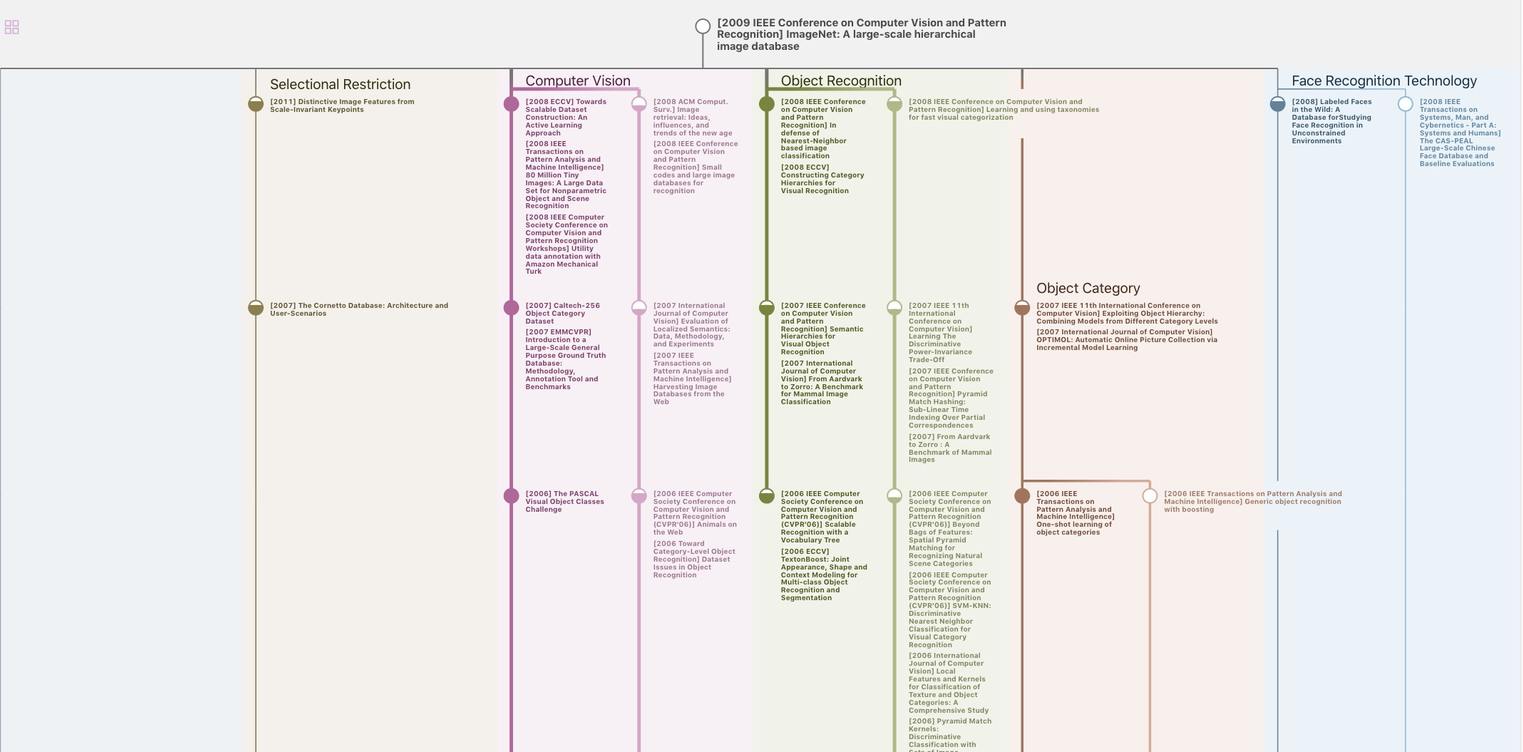
生成溯源树,研究论文发展脉络
Chat Paper
正在生成论文摘要