Convolutional Fusion Network for Face Verification in the Wild
IEEE Trans. Circuits Syst. Video Techn.(2016)
摘要
Part-based methods have seen popular applications for face verification in the wild, since they are more robust to local variations in terms of pose, illumination and so on. However, most of the part-based approaches are built on hand-crafted features, which may be not suitable for the specific face verification purpose. In this work, we propose to learn a part-based feature representation under the supervision of face identities through a deep model, which ensures the generated representations are more robust and suitable for face verification. The proposed framework consists of following two deliberate components: a Deep Mixture Model (DMM) to find accurate patch correspondence and a Convolutional Fusion Network (CFN) to extract the part based facial features. Specifically, DMM robustly depicts the spatialappearance distribution of patch features over the faces via several Gaussian mixtures, which provide more accurate patch correspondence even in the presence of local distortions. Then, DMM only feeds the patches which preserve the identity information to the following CFN. The proposed CFN is a twolayer cascade of Convolutional Neural Networks (CNN): 1) a local layer built on face patches to deal with local variations and 2) a fusion layer integrating the responses from the local layer. CFN jointly learns and fuses multiple local responses to optimize the verification performance. The composite representation obtained possesses certain robustness to pose and illumination variations and shows comparable performance with the state-of-the-arts on two benchmark data sets.
更多查看译文
关键词
deep learning,feature learning,part-based representation
AI 理解论文
溯源树
样例
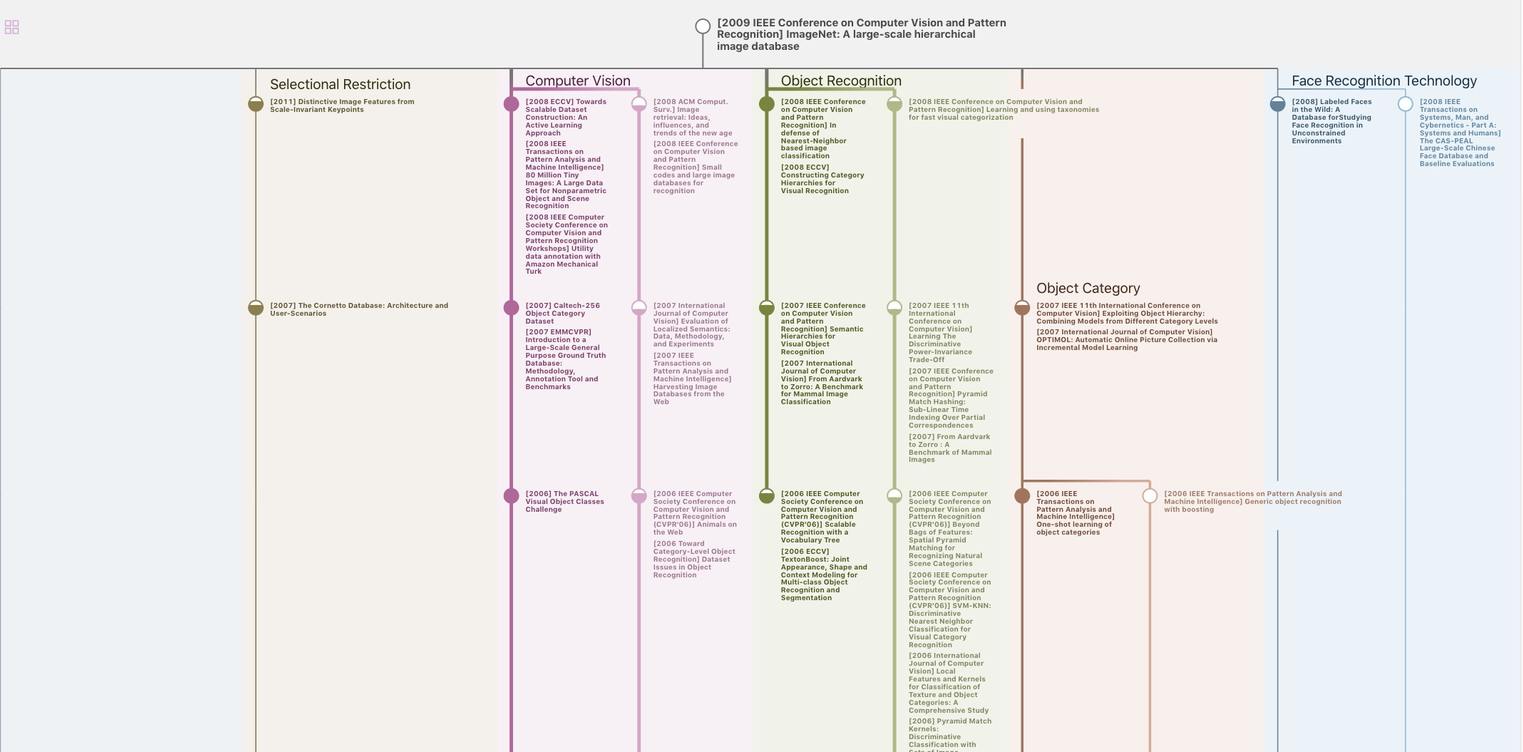
生成溯源树,研究论文发展脉络
Chat Paper
正在生成论文摘要