Reverse Nearest Neighbors in Unsupervised Distance-Based Outlier Detection
Knowledge and Data Engineering, IEEE Transactions (2015)
摘要
Outlier detection in high-dimensional data presents various challenges resulting from the “curse of dimensionality.” A prevailing view is that distance concentration, i.e., the tendency of distances in high-dimensional data to become indiscernible, hinders the detection of outliers by making distance-based methods label all points as almost equally good outliers. In this paper, we provide evidence supporting the opinion that such a view is too simple, by demonstrating that distance-based methods can produce more contrasting outlier scores in high-dimensional settings. Furthermore, we show that high dimensionality can havea different impact, by reexamining the notion of reverse nearest neighbors in the unsupervised outlier-detection context. Namely, it was recently observed that the distribution of points’ reverse-neighbor counts becomes skewed in high dimensions, resulting in the phenomenon known as hubness. We provide insight into how some points (antihubs) appear very infrequently in k-NN lists of other points, and explain the connection between antihubs, outliers, and existing unsupervised outlier-detection methods. By evaluating the classic k-NN method, the angle-based technique designed for high-dimensional data, the density-based local outlier factor and influenced outlierness methods, and anti hub-based methods on various synthetic and real-world data sets, we offer novel insight into the usefulness of reverse neighbor counts in unsupervised outlier detection
更多查看译文
关键词
data analysis,pattern classification,unsupervised learning,data dimensionality,distance concentration,k-nn method,reverse nearest neighbor,unsupervised outlier detection,outlier detection,high-dimensional data,reverse nearest neighbors,noise measurement,high dimensional data,correlation,euclidean distance,histograms
AI 理解论文
溯源树
样例
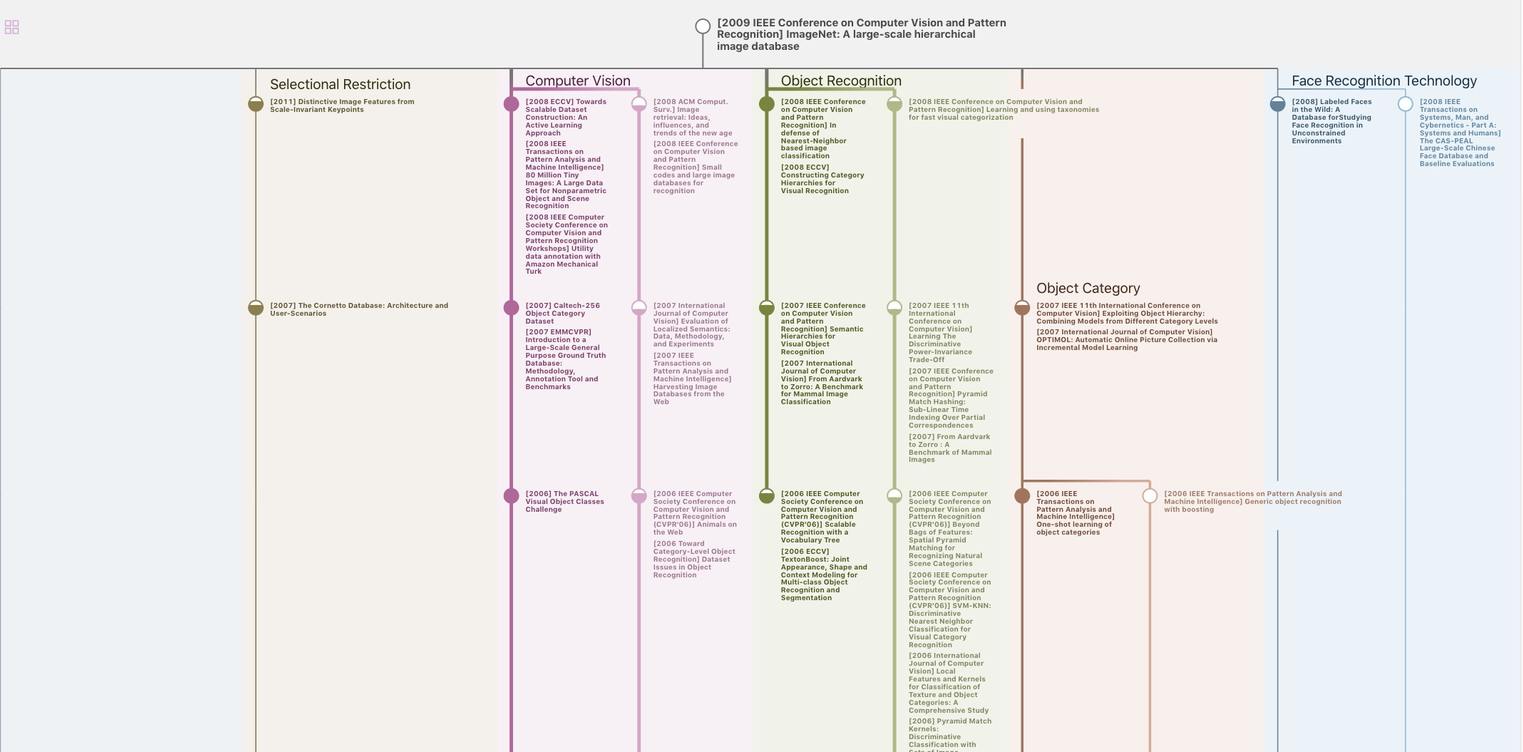
生成溯源树,研究论文发展脉络
Chat Paper
正在生成论文摘要