Fall detection in homes of older adults using the Microsoft Kinect.
IEEE journal of biomedical and health informatics(2015)
摘要
A method for detecting falls in the homes of older adults using the Microsoft Kinect and a two-stage fall detection system is presented. The first stage of the detection system characterizes a person's vertical state in individual depth image frames, and then segments on ground events from the vertical state time series obtained by tracking the person over time. The second stage uses an ensemble of decision trees to compute a confidence that a fall preceded on a ground event. Evaluation was conducted in the actual homes of older adults, using a combined nine years of continuous data collected in 13 apartments. The dataset includes 454 falls, 445 falls performed by trained stunt actors and nine naturally occurring resident falls. The extensive data collection allows for characterization of system performance under real-world conditions to a degree that has not been shown in other studies. Cross validation results are included for standing, sitting, and lying down positions, near (within 4 m) versus far fall locations, and occluded versus not occluded fallers. The method is compared against five state-of-the-art fall detection algorithms and significantly better results are achieved.
更多查看译文
关键词
real-world condition,biomechanics,two-stage fall detection system,time 9 year,lying down position,microsoft kinect,home fall detection,biomedical optical imaging,vertical state time series,system performance characterization,not occluded faller,geriatrics,kinect,sitting position,fall precedence,person tracking,near fall location,person vertical state characterization,continuous data collection,biomedical equipment,accidents,data acquisition,older adults fall detection,naturally occurring resident fall,image classification,individual depth image frame,object tracking,cross validation,ground event segmentation,home computing,confidence computation,fall detection algorithm,fall detection,decision tree,decision trees,time series,older adults,standing position,medical image processing,far fall location,image motion analysis,time series analysis,human factors,occupational safety,injury prevention,suicide prevention,informatics,sensors,ergonomics,feature extraction
AI 理解论文
溯源树
样例
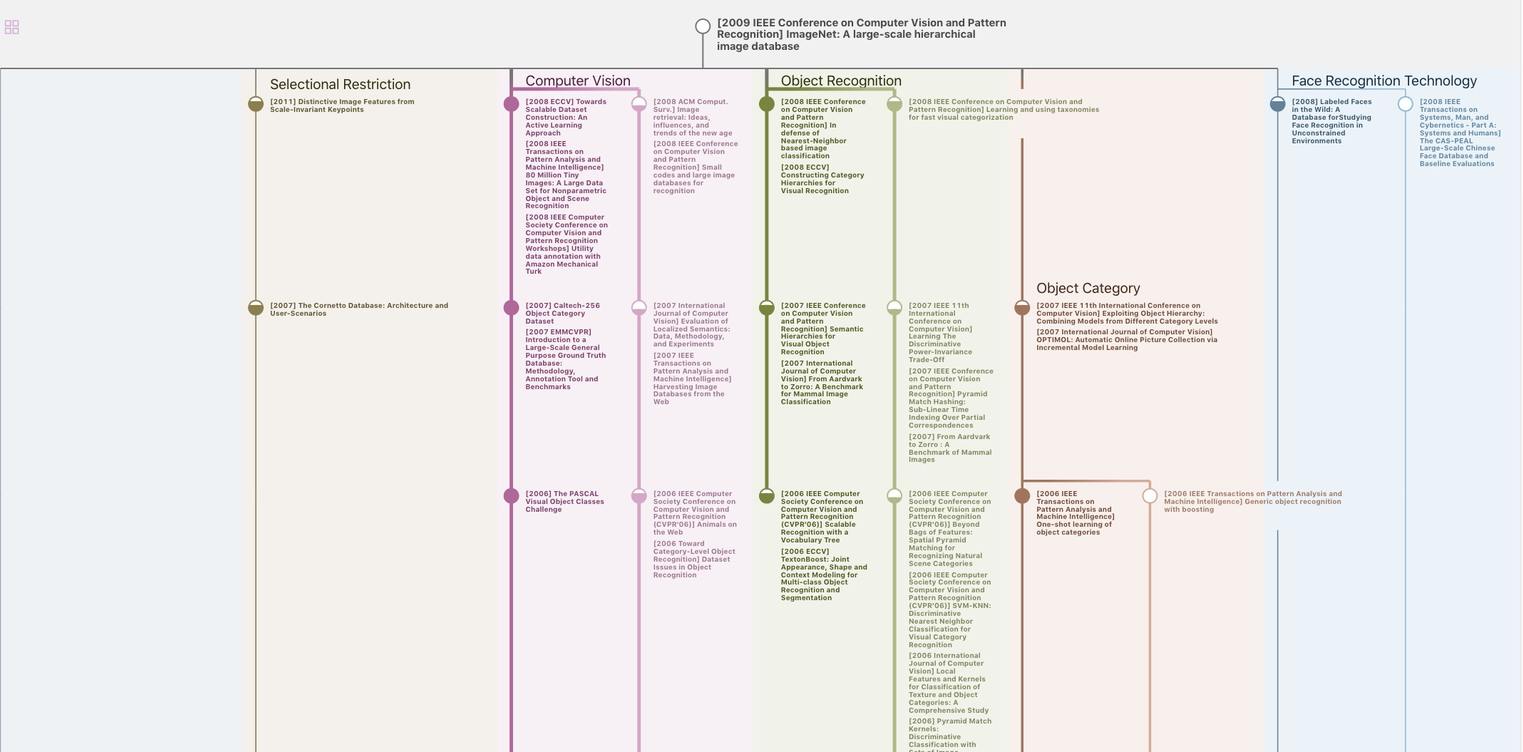
生成溯源树,研究论文发展脉络
Chat Paper
正在生成论文摘要