Spotting Fake Reviews via Collective Positive-Unlabeled Learning
Data Mining(2014)
摘要
Online reviews have become an increasingly important resource for decision making and product designing. But reviews systems are often targeted by opinion spamming. Although fake review detection has been studied by researchers for years using supervised learning, ground truth of large scale datasets is still unavailable and most of existing approaches of supervised learning are based on pseudo fake reviews rather than real fake reviews. Working with Dianping, the largest Chinese review hosting site, we present the first reported work on fake review detection in Chinese with filtered reviews from Dianping's fake review detection system. Dianping's algorithm has a very high precision, but the recall is hard to know. This means that all fake reviews detected by the system are almost certainly fake but the remaining reviews (unknown set) may not be all genuine. Since the unknown set may contain many fake reviews, it is more appropriate to treat it as an unlabeled set. This calls for the model of learning from positive and unlabeled examples (PU learning). By leveraging the intricate dependencies among reviews, users and IP addresses, we first propose a collective classification algorithm called Multi-typed Heterogeneous Collective Classification (MHCC) and then extend it to Collective Positive and Unlabeled learning (CPU). Our experiments are conducted on real-life reviews of 500 restaurants in Shanghai, China. Results show that our proposed models can markedly improve the F1 scores of strong baselines in both PU and non-PU learning settings. Since our models only use language independent features, they can be easily generalized to other languages.
更多查看译文
关键词
supervised learning,multityped heterogeneous collective classification,opinion spamming,collective pu learning,dianping fake review detection system,online review,learning (artificial intelligence),pattern classification,cpu learning,internet,spam detection,collective positive and unlabeled learning,mhcc,data mining,data models,nickel,testing,training data,reliability
AI 理解论文
溯源树
样例
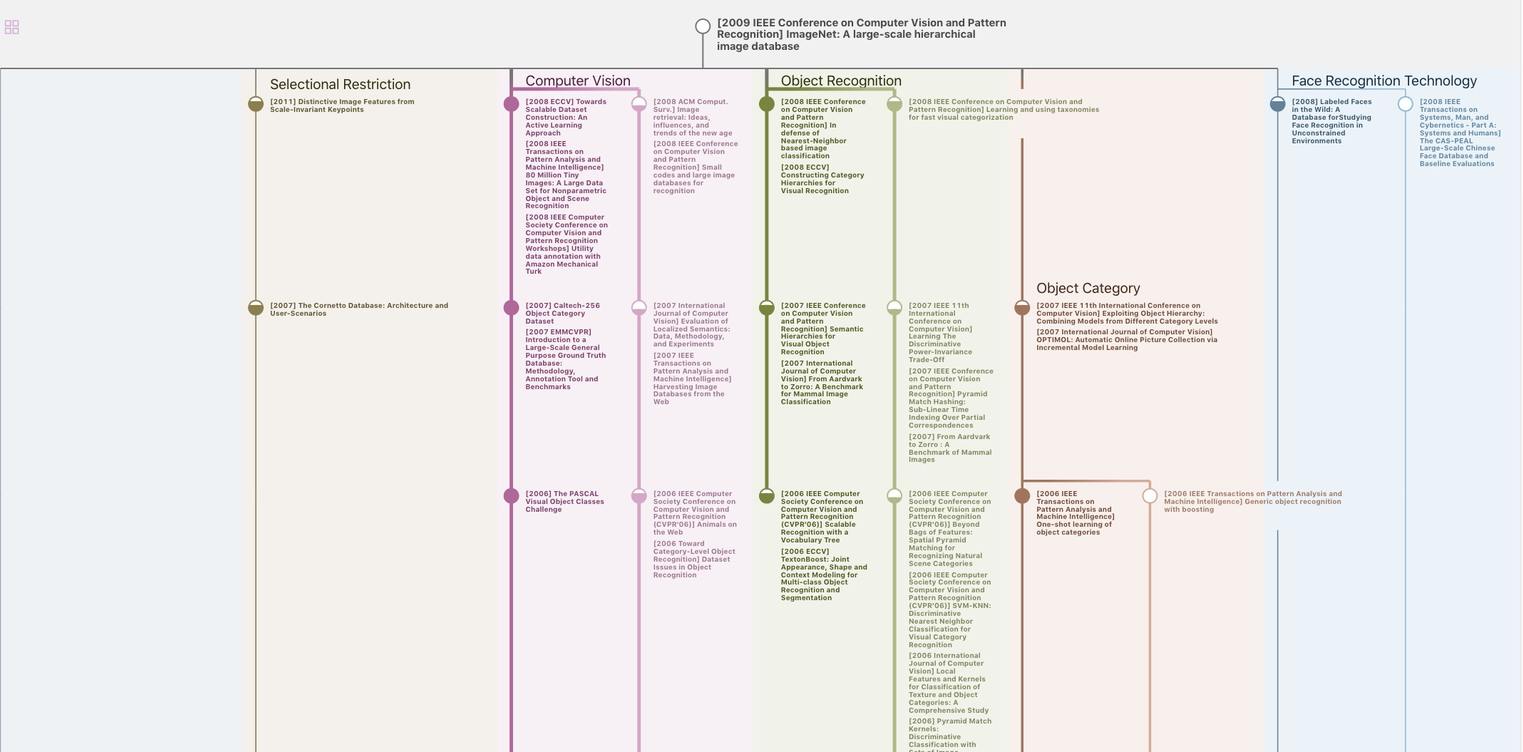
生成溯源树,研究论文发展脉络
Chat Paper
正在生成论文摘要