aTGV-SF: Dense Variational Scene Flow through Projective Warping and Higher Order Regularization
3DV), 2014 2nd International Conference (2014)
摘要
In this paper we present a novel method to accurately estimate the dense 3D motion field, known as scene flow, from depth and intensity acquisitions. The method is formulated as a convex energy optimization, where the motion warping of each scene point is estimated through a projection and back-projection directly in 3D space. We utilize higher order regularization which is weighted and directed according to the input data by an anisotropic diffusion tensor. Our formulation enables the calculation of a dense flow field which does not penalize smooth and non-rigid movements while aligning motion boundaries with strong depth boundaries. An efficient parallelization of the numerical algorithm leads to runtimes in the order of 1s and therefore enables the method to be used in a variety of applications. We show that this novel scene flow calculation outperforms existing approaches in terms of speed and accuracy. Furthermore, we demonstrate applications such as camera pose estimation and depth image super resolution, which are enabled by the high accuracy of the proposed method. We show these applications using modern depth sensors such as Microsoft Kinect or the PMD Nano Time-of-Flight sensor.
更多查看译文
关键词
convex programming,image resolution,image sensors,image sequences,motion estimation,pose estimation,tensors,Microsoft Kinect,PMD nano time-of-flight sensor,aTGV-SF,anisotropic diffusion tensor,back-projection,camera pose estimation,convex energy optimization,dense 3D motion field estimation,dense variational scene flow,depth acquisition,depth image super resolution,depth sensors,higher order regularization,intensity acquisition,numerical algorithm,projective warping,scene point estimation,Camera Pose Estiamtion,Convex Optimization,RGB-D Sensor,Scene Flow,Superresolution,Time-of-Flight
AI 理解论文
溯源树
样例
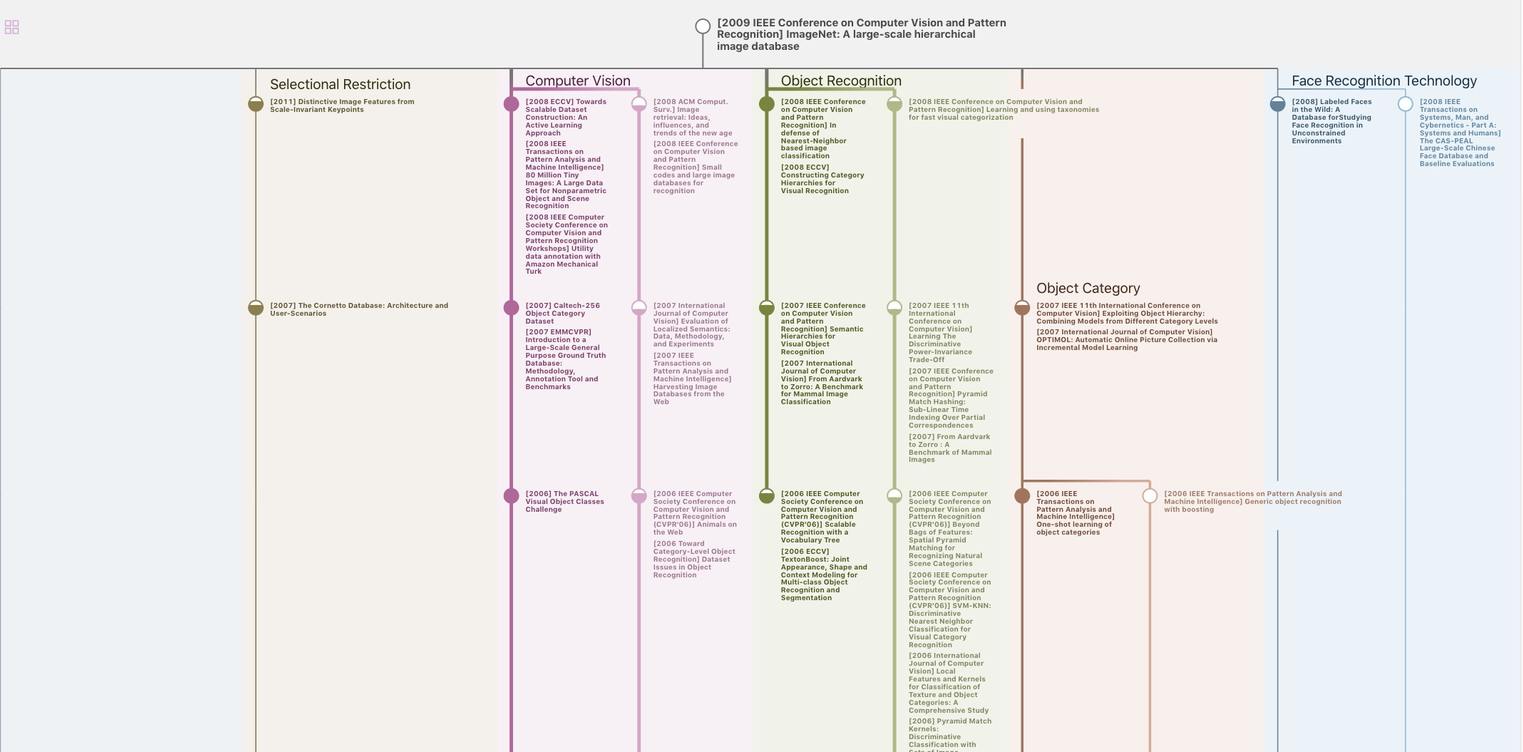
生成溯源树,研究论文发展脉络
Chat Paper
正在生成论文摘要