ApproxANN: an approximate computing framework for artificial neural network
DATE(2015)
摘要
Artificial Neural networks (ANNs) are one of the most well-established machine learning techniques and have a wide range of applications, such as Recognition, Mining and Synthesis (RMS). As many of these applications are inherently error-tolerant, in this work, we propose a novel approximate computing framework for ANN, namely ApproxANN. When compared to existing solutions, ApproxANN considers approximation for both computation and memory accesses, thereby achieving more energy savings. To be specific, ApproxANN characterizes the impact of neurons on the output quality in an effective and efficient manner, and judiciously determine how to approximate the computation and memory accesses of certain less critical neurons to achieve the maximum energy efficiency gain under a given quality constraint. Experimental results on various ANN applications with different datasets demonstrate the efficacy of the proposed solution.
更多查看译文
关键词
artificial neural networks,neural nets,cache,artificial neural network,hardware,degradation
AI 理解论文
溯源树
样例
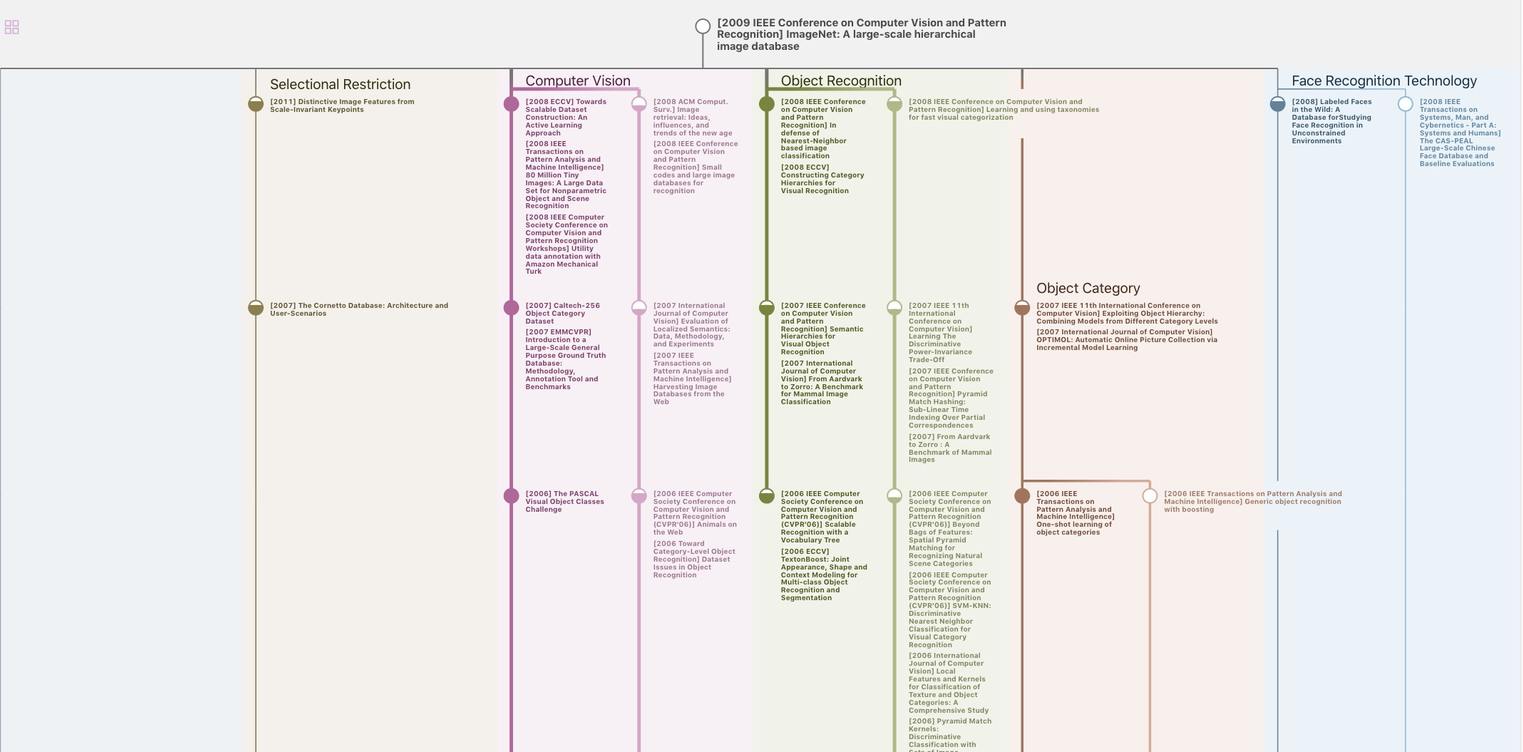
生成溯源树,研究论文发展脉络
Chat Paper
正在生成论文摘要