Evaluation of semi-supervised learning method on action recognition
Multimedia Tools Appl.(2014)
摘要
Action recognition is one of the most difficult problems in computer vision and multimedia areas, since both spatial information and spatiotemporal semantic meaning should be taken into consideration. Moreover, the noisy and weakly annotated information make this task even harder. Nowadays, instead of the traditional features and classifiers, a lot of new attempts have made the task of action recognition promising. Noticing that there is no work on comparison of different combination of pooling and semi-supervised learning method under the same experiment setting, it would be interesting to apply different combination of pooling and semi-supervised learning method on both the synthetic and realistic action recognition datasets to see which combination or method performs better. In summary, we can obtain the following conclusions based on our experiments. Firstly, Second Order Pooling (Carreira et al. 2012 ) is worse than the traditional Bag of Words (Schmid and Mohr 1997 ; Dance et al. 2004 ) regarding to the overall performance in some dataset, but is a good way to speed up the coding stage of video classification with little sacrifice of performance. Secondly, Semi-supervised Hierarchical Regression Algorithm (MLHR) and Manifold Regularized Least Square Regression (MRLS) (Belkin et al. J Mach Learn Res 12:2399–2434, 2006 ) is better than some of the supervised learning methods ( χ 2 -SVM, SVM-2K (Farquhar et al. 2006 )) in the real world action recognition problems which shares little available annotated information. Thirdly, for KTH, UCF50 and HMDB dataset, late fusion doesn’t necessarily improve the performance. In comparison, MLHR, SVM-2K and Multi-kernel Learning is a more natural way to deal with multi-feature problems.
更多查看译文
关键词
Semi-supervised learning,Multi-feature fusion,Second order pooling,Video concept annotation,Action recognition
AI 理解论文
溯源树
样例
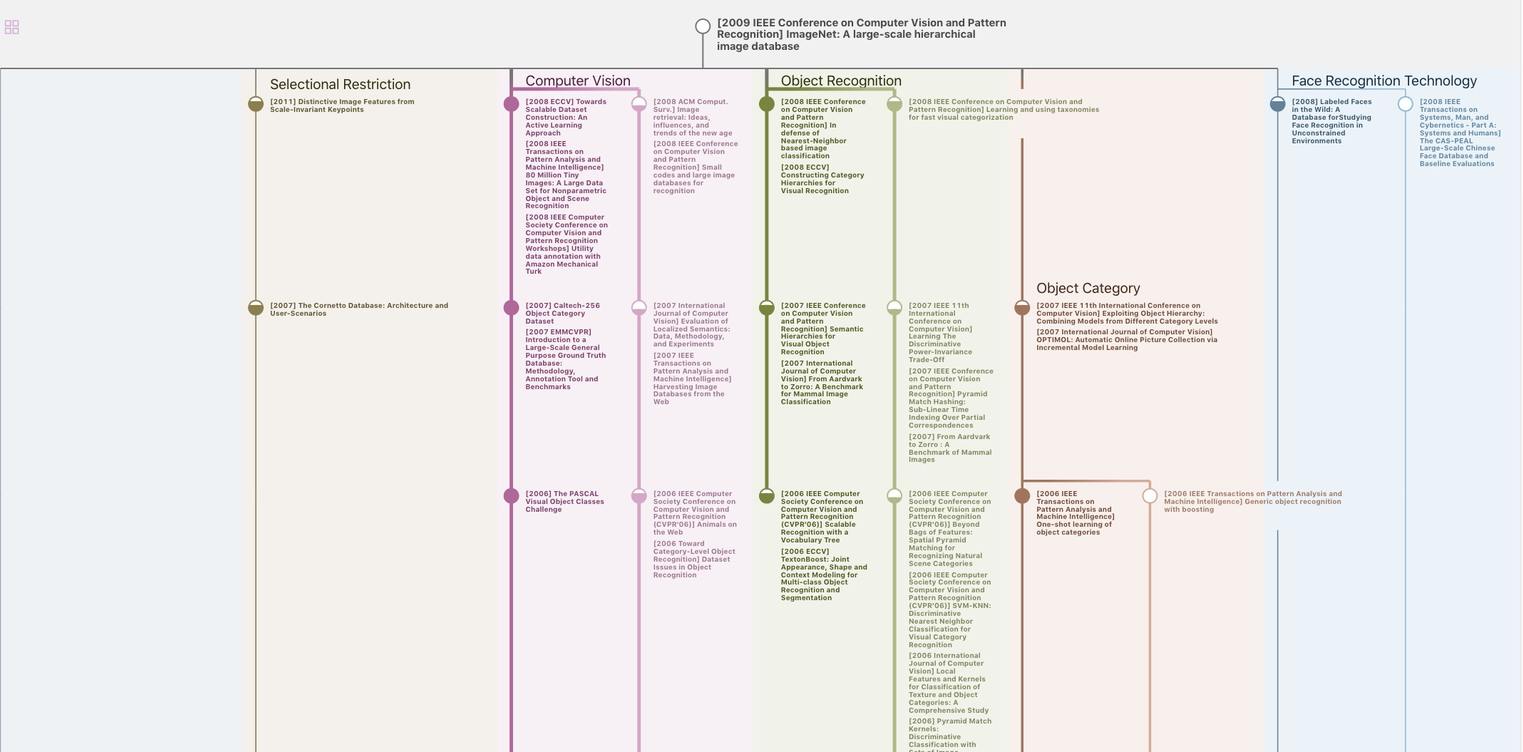
生成溯源树,研究论文发展脉络
Chat Paper
正在生成论文摘要