Training Computationally Efficient SmartphoneBased Human Activity Recognition Models
ARTIFICIAL NEURAL NETWORKS AND MACHINE LEARNING - ICANN 2013(2013)
摘要
The exploitation of smartphones for Human Activity Recognition (HAR) has been an active research area in which the development of fast and efficient Machine Learning approaches is crucial for preserving battery life and reducing computational requirements. In this work, we present a HAR system which incorporates smartphone-embedded inertial sensors and uses Support Vector Machines (SVM) for the classification of Activities of Daily Living (ADL). By exploiting a publicly available benchmark HAR dataset, we show the benefits of adding smartphones gyroscope signals into the recognition system against the traditional accelerometer-based approach, and explore two feature selection mechanisms for allowing a radically faster recognition: the utilization of exclusively time domain features and the adaptation of the L1 SVM model which performs comparably to non-linear approaches while neglecting a large number of non-informative features.
更多查看译文
关键词
svm,feature selection
AI 理解论文
溯源树
样例
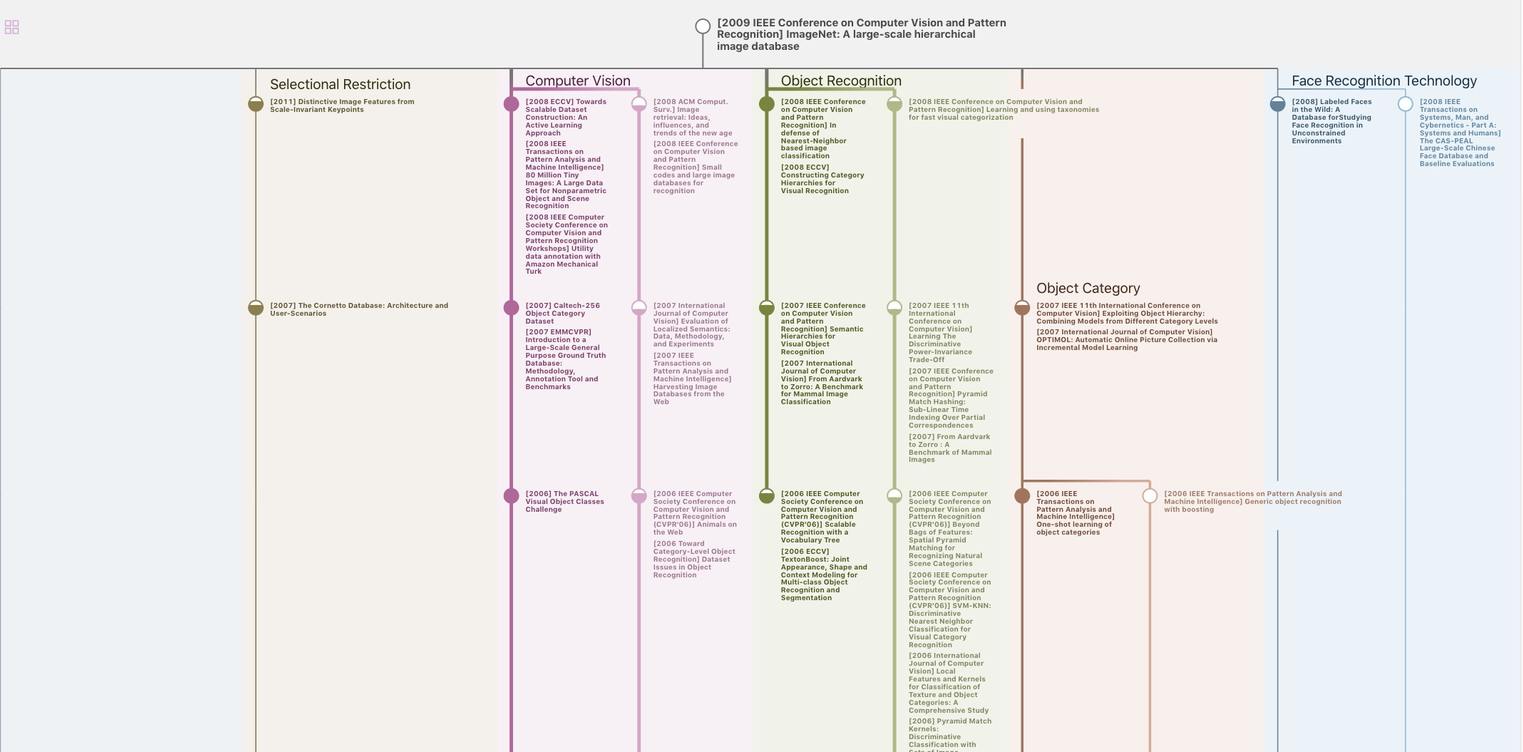
生成溯源树,研究论文发展脉络
Chat Paper
正在生成论文摘要