Network-scale traffic modeling and forecasting with graphical lasso and neural networks
JOURNAL OF TRANSPORTATION ENGINEERING(2018)
摘要
Traffic flow forecasting, especially the short-term case, is an important topic in intelligent transportation systems (ITS). This paper researches network-scale modeling and forecasting of short-term traffic flows. First, the concepts of single-link and multilink models of traffic flow forecasting are proposed. Secondly, four prediction models are constructed by combining the two models with single-task learning (STL) and multitask learning (MTL). The combination of the multilink model and multitask learning not only improves the experimental efficiency but also improves the prediction accuracy. Moreover, a new multilink, single-task approach that combines graphical lasso (GL) with neural network (NN) is proposed. GL provides a general methodology for solving problems involving lots of variables. Using L1 regularization, GL builds a sparse graphical model, making use of the sparse inverse covariance matrix. Gaussian process regression (GPR) is a classic regression algorithm in Bayesian machine learning. Although there is wide research on GPR, there are few applications of GPR in traffic flow forecasting. In this paper, GPR is applied to traffic flow forecasting, and its potential is shown. Through sufficient experiments, all of the proposed approaches are compared, and an overall assessment is made. DOI: 10.1061/(ASCE)TE.1943-5436.0000435. (C) 2012 American Society of Civil Engineers.
更多查看译文
关键词
Traffic flow forecasting,Graphical lasso (GL),Neural network (NN),Gaussian process regression (GPR)
AI 理解论文
溯源树
样例
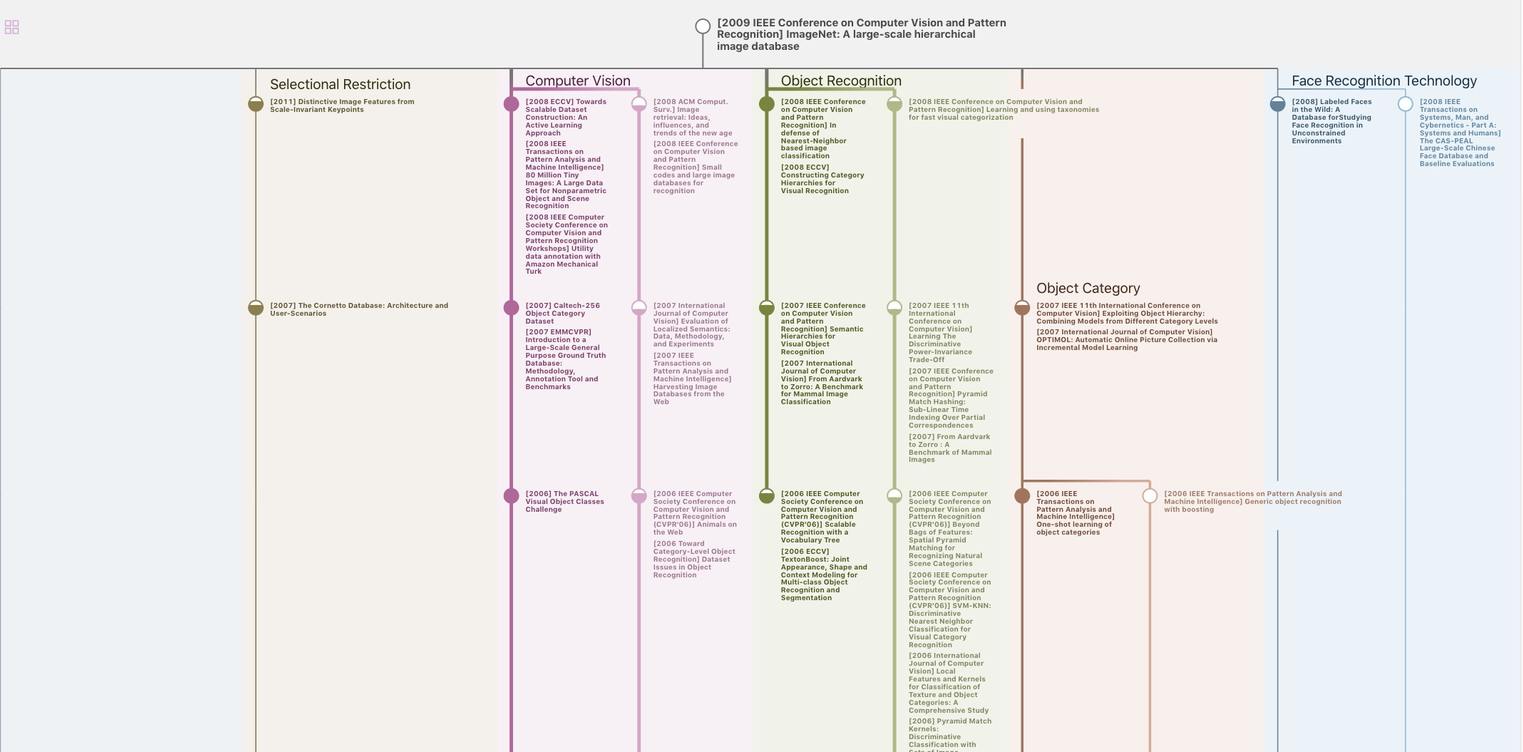
生成溯源树,研究论文发展脉络
Chat Paper
正在生成论文摘要