Conversational Speech Transcription Using Context-Dependent Deep Neural Networks
12TH ANNUAL CONFERENCE OF THE INTERNATIONAL SPEECH COMMUNICATION ASSOCIATION 2011 (INTERSPEECH 2011), VOLS 1-5(2012)
摘要
We apply the recently proposed Context-Dependent Deep-Neural-Network HMMs, or CD-DNN-HMMs, to speech-to-text transcription. For single-pass speaker-independent recognition on the RT03S Fisher portion of phone-call transcription benchmark (Switchboard), the word-error rate is reduced from 27.4%, obtained by discriminatively trained Gaussian-mixture HMMs, to 18.5%-a 33% relative improvement.CD-DNN-HMMs combine classic artificial-neural-network HMMs with traditional tied-state triphones and deep-belief-network pre-training. They had previously been shown to reduce errors by 16% relatively when trained on tens of hours of data using hundreds of tied states. This paper takes CD-DNN-HMMs further and applies them to transcription using over 300 hours of training data, over 9000 tied states, and up to 9 hidden layers, and demonstrates how sparseness can be exploited.On four less well-matched transcription tasks, we observe relative error reductions of 22-28%.
更多查看译文
关键词
speech recognition, deep belief networks, deep neural networks
AI 理解论文
溯源树
样例
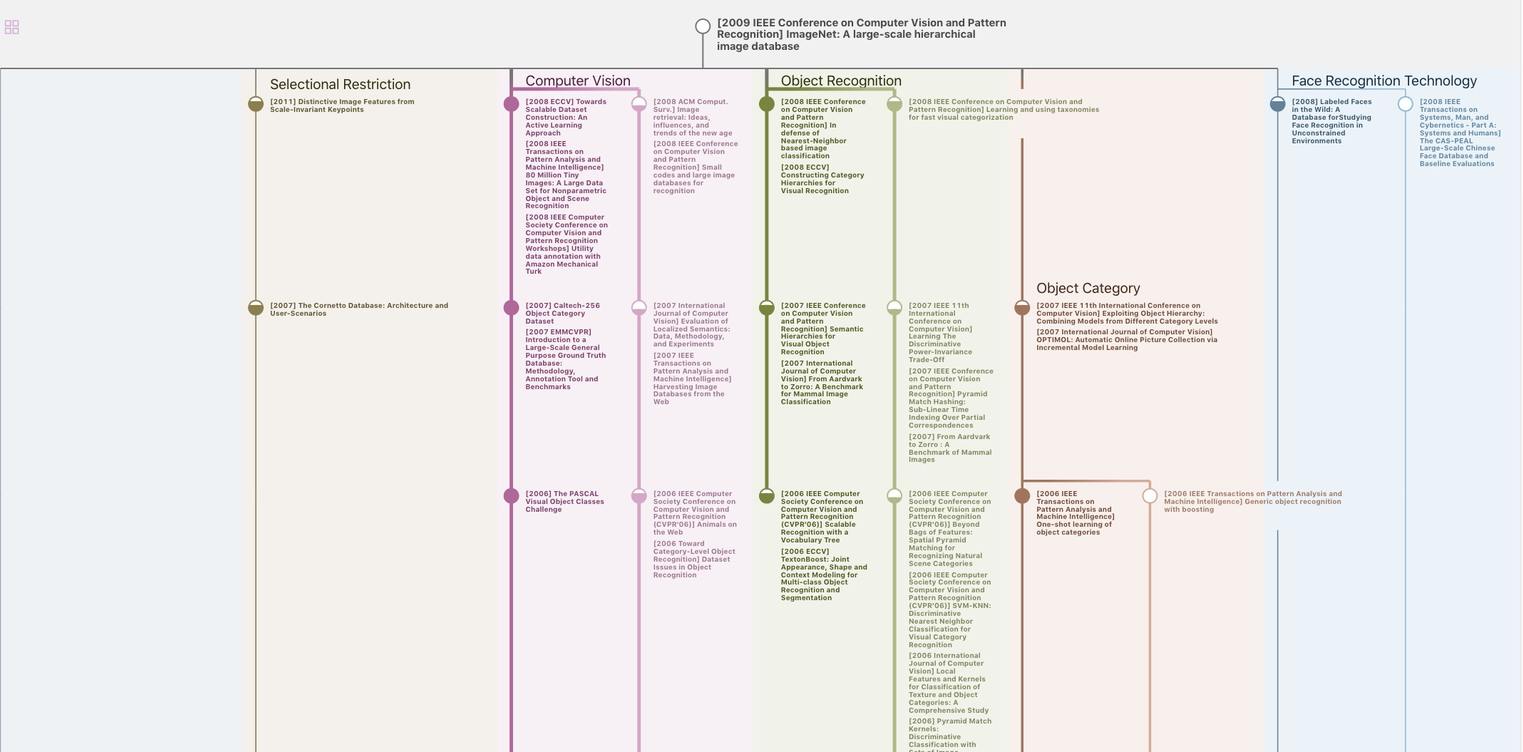
生成溯源树,研究论文发展脉络
Chat Paper
正在生成论文摘要