Fast point feature histograms (FPFH) for 3D registration
ICRA(2009)
摘要
In our recent work [1], [2], we proposed Point Feature Histograms (PFH) as robust multi-dimensional features which describe the local geometry around a point p for 3D point cloud datasets. In this paper, we modify their mathematical expressions and perform a rigorous analysis on their robustness and complexity for the problem of 3D registration for overlapping point cloud views. More concretely, we present several optimizations that reduce their computation times drastically by either caching previously computed values or by revising their theoretical formulations. The latter results in a new type of local features, called Fast Point Feature Histograms (FPFH), which retain most of the discriminative power of the PFH. Moreover, we propose an algorithm for the online computation of FPFH features for realtime applications. To validate our results we demonstrate their efficiency for 3D registration and propose a new sample consensus based method for bringing two datasets into the convergence basin of a local non-linear optimizer: SAC-IA (SAmple Consensus Initial Alignment).
更多查看译文
关键词
local geometry,fast point feature histograms,local non-linear optimizer,fpfh feature,overlapping point cloud view,computation time,point cloud datasets,point feature histograms,fast point feature histogram,point p,local feature,meteorology,intelligent systems,optimization problem,computational complexity,point cloud,robustness,optimization,convergence,histograms,feature extraction,metric space,iterative closest point,stochastic optimization,data mining,genetic algorithm
AI 理解论文
溯源树
样例
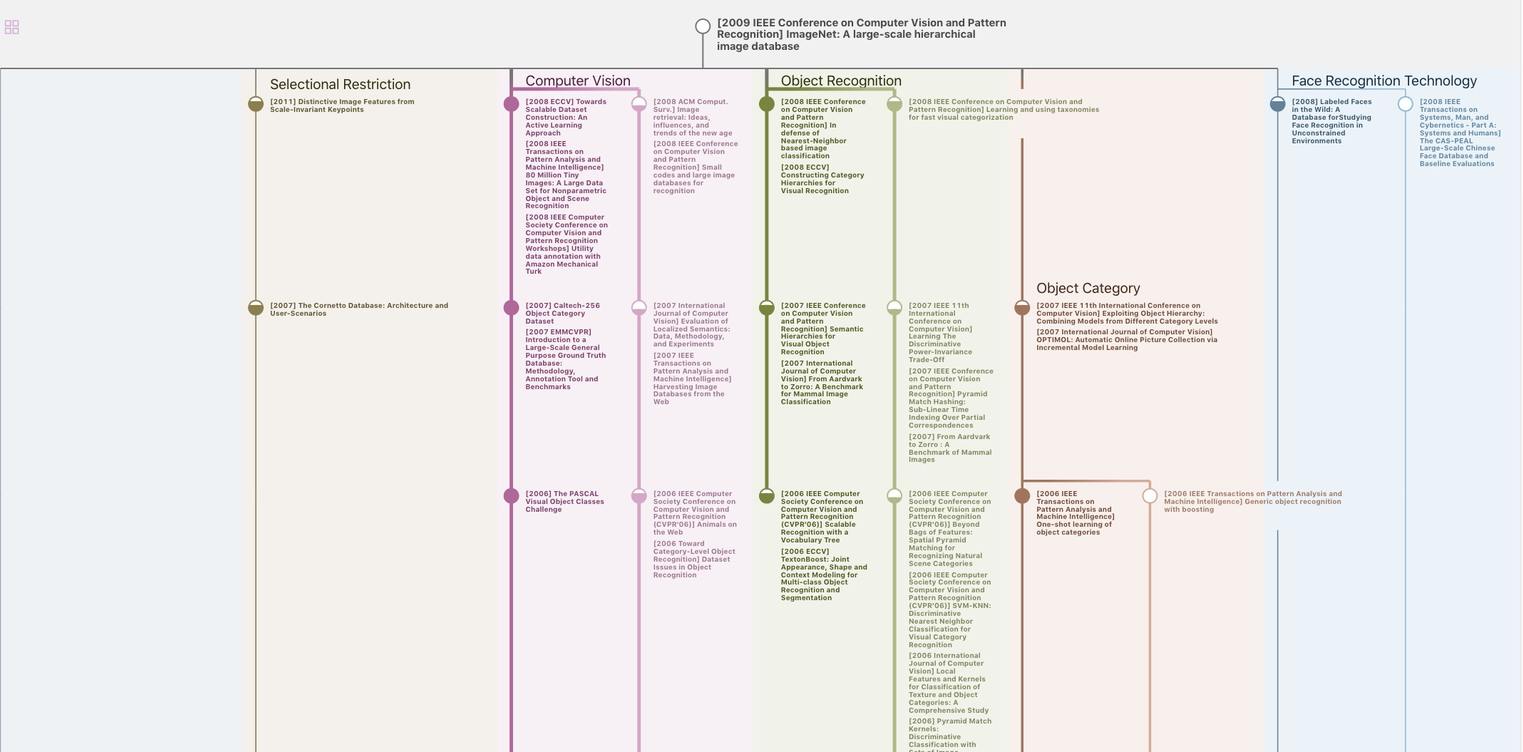
生成溯源树,研究论文发展脉络
Chat Paper
正在生成论文摘要