A Novel Procedure for Training L1-L2 Support Vector Machine Classifiers
ICANN(2013)
摘要
In this work we propose a novel algorithm for training L1-L2 Support Vector Machine (SVM) classifiers. L1-L2 SVMs allow to combine the effectiveness of L2 models and the feature selection characteristics of L1 solutions. The proposed training approach for L1-L2 SVM requires a minimal effort for its implementation, relying on the exploitation of well-known and widespread tools already developed for conventional L2 SVMs. Moreover, the proposed method is flexible, as it allows to train L1, L1-L2 and L2 SVMs, as well as to fine tune the trade-off between dimensionality reduction and classification accuracy. This scope is of clear importance in applications on resource-limited devices, such as smartphones, like the one we consider to verify the main advantages of the proposed approach: the UCI Human Activity Recognition real-world dataset.
更多查看译文
关键词
support vector machine
AI 理解论文
溯源树
样例
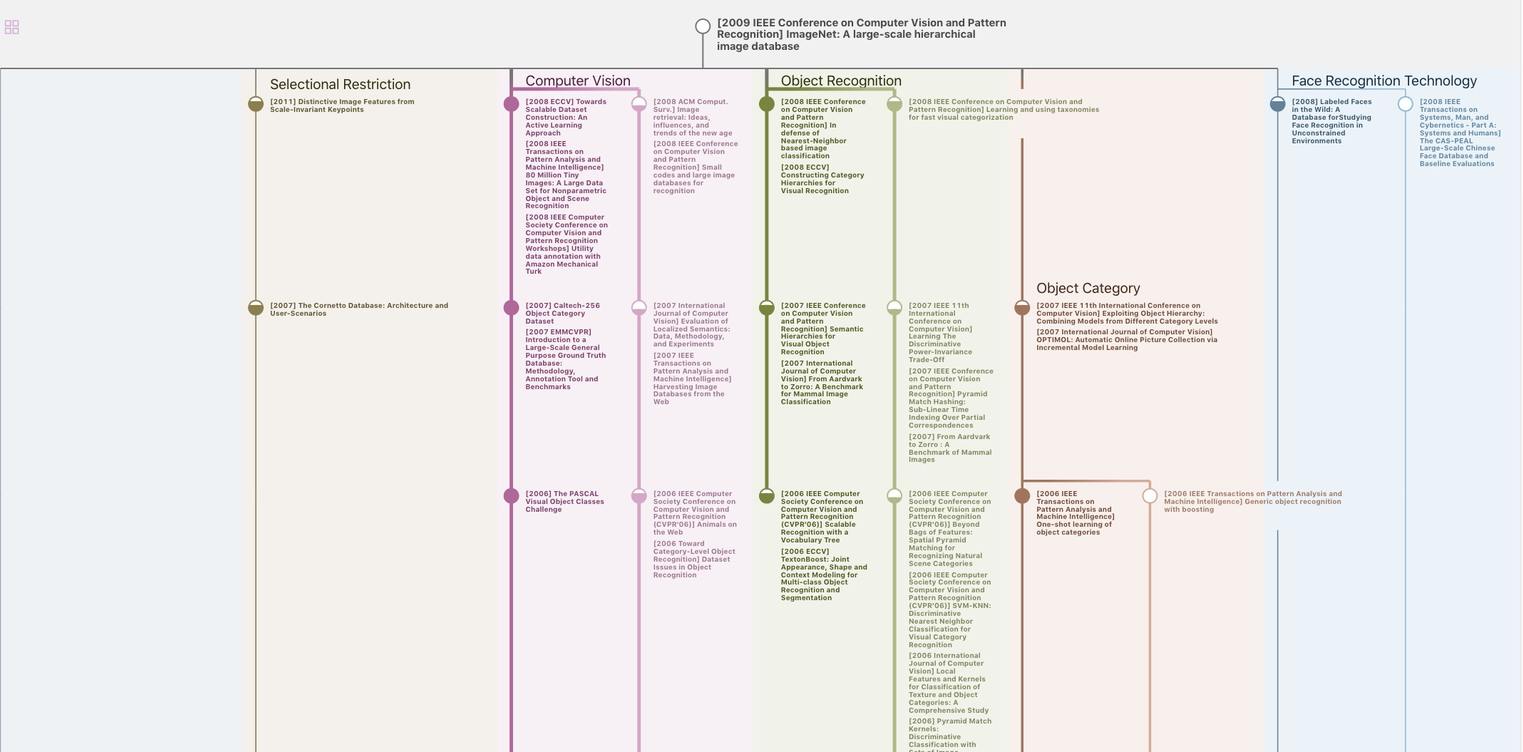
生成溯源树,研究论文发展脉络
Chat Paper
正在生成论文摘要