Combination Of Sparse Classification And Multilayer Perceptron For Noise-Robust Asr
13TH ANNUAL CONFERENCE OF THE INTERNATIONAL SPEECH COMMUNICATION ASSOCIATION 2012 (INTERSPEECH 2012), VOLS 1-3(2012)
摘要
On the AURORA-2 task good results at low SNR levels have been obtained with a system that uses state posterior estimates provided by an exemplar-based sparse classification (SC) system. At the same time, posterior estimates obtained with a multilayer perceptron (MLP) yield good results at high SNRs. In this paper, we investigate the effect of combining the estimates from the SC and MLP systems at the probability level. More precisely, the probabilities are combined by a sum or a product rule using static and inverse-entropy based dynamic weights. In addition, we investigate a modified dynamic weighting approach which enhances the contribution of the SC stream based on the information about static weights and average dynamic weights obtained on cross-validation data. Our study on the AURORA-2 task shows that in all conditions the modified dynamic weighting approach yields a dual-input system that performs better than or equal to the best stand-alone system.
更多查看译文
关键词
multiple-stream combination,noise robustness,exemplar-based system,multilayer perceptron network
AI 理解论文
溯源树
样例
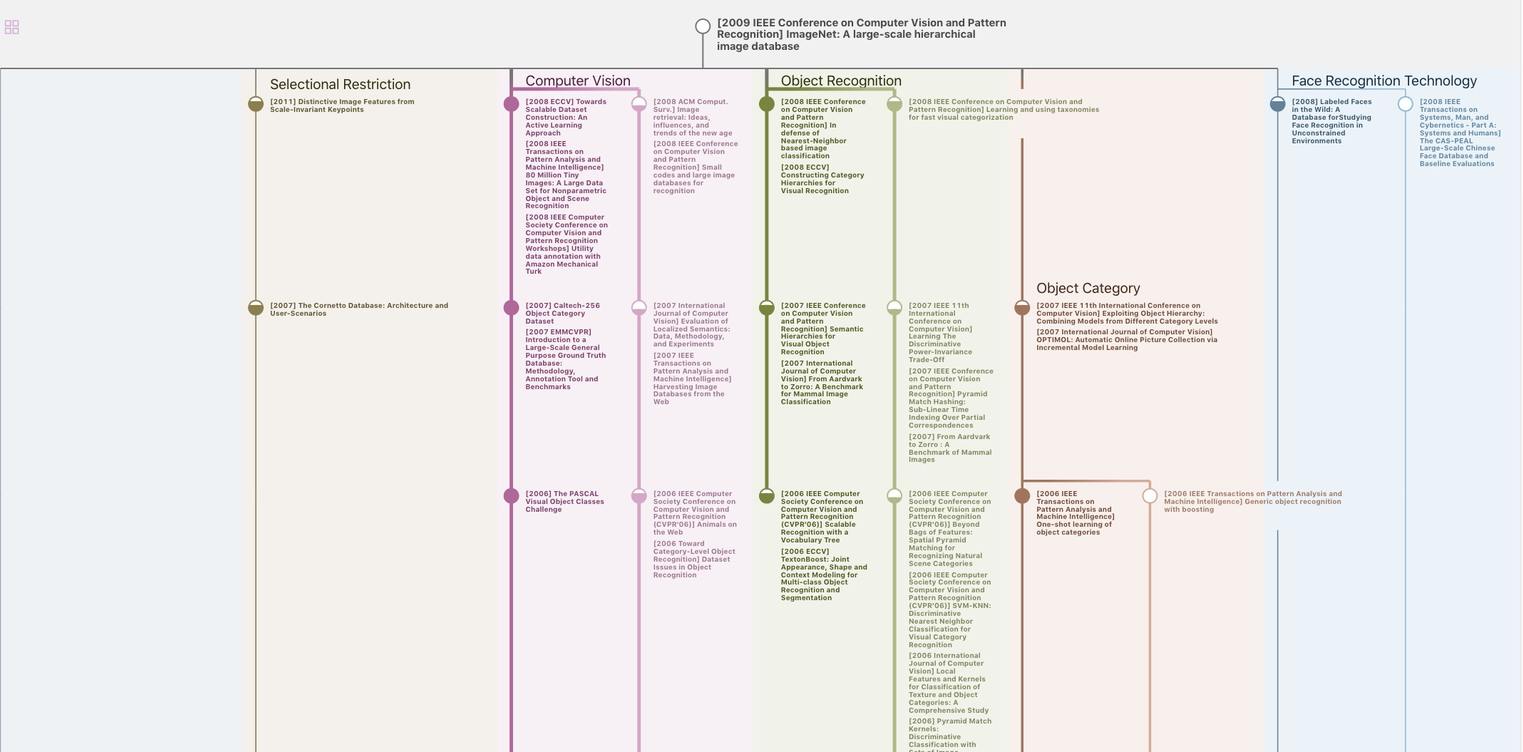
生成溯源树,研究论文发展脉络
Chat Paper
正在生成论文摘要