Protein-Protein Binding Affinity Prediction Based on an SVR Ensemble.
ICIC (1)(2012)
摘要
Accurately predicting generic protein-protein binding affinities (PPBA) is essential to analyze the outputs of protein docking and may help infer real status of cellular protein-protein interaction sub-networks. However, accurate PPBA prediction is still extremely challenging. Machine learning methods are promising to address this problem. We propose a two-layer support vector regression (TLSVR) model to implicitly capture binding contributions that are hard to explicitly model. The TLSVR circumvents both the descriptor compatibility problem and the need for problematic modeling assumptions. Input features for TLSVR in first layer are scores of 2209 interacting atom pairs within each distance bin. The base SVRs are combined by the second layer to infer the final affinities. Leave-one-out validation on our heterogeneous data shows that the TLSVR method obtains a very good result of R=0.80 and SD=1.32 with real affinities. Comparison experiment further demonstrates that TLSVR is superior to the previous state-of-art methods in predicting generic PPBA. © 2012 Springer-Verlag.
更多查看译文
关键词
machine learning,potential of mean force,protein-protein interaction affinity,two-layer support vector machine
AI 理解论文
溯源树
样例
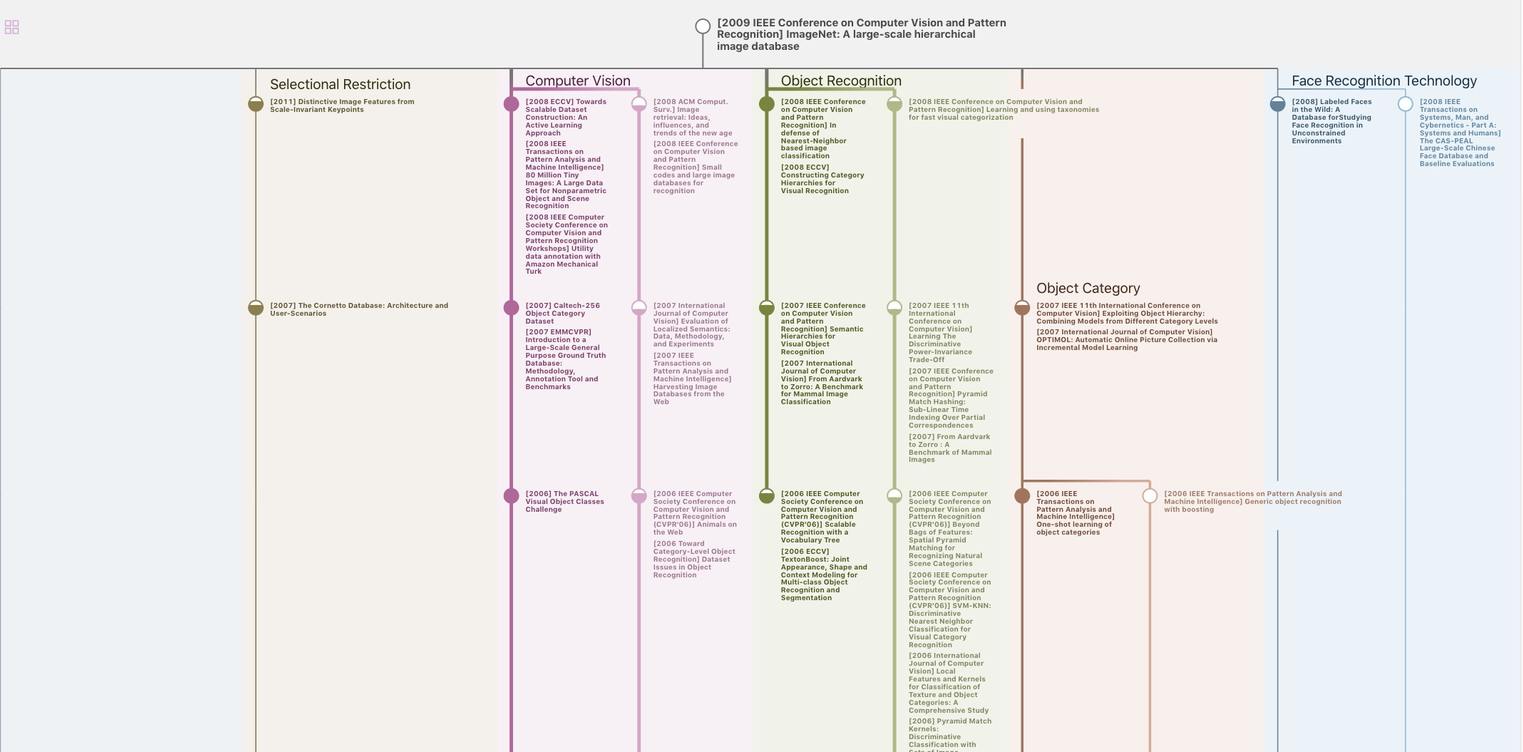
生成溯源树,研究论文发展脉络
Chat Paper
正在生成论文摘要