Semi-Supervised Nonlinear Distance Metric Learning via Forests of Max-Margin Cluster Hierarchies.
IEEE Transactions on Knowledge and Data Engineering(2016)
摘要
Metric learning is a key problem for many data mining and machine learning applications, and has long been dominated by Mahalanobis methods. Recent advances in nonlinear metric learning have demonstrated the potential power of non-Mahalanobis distance functions, particularly tree-based functions. We propose a novel nonlinear metric learning method that uses an iterative, hierarchical variant of semi-supervised max-margin clustering to construct a forest of cluster hierarchies, where each individual hierarchy can be interpreted as a weak metric over the data. By introducing randomness during hierarchy training and combining the output of many of the resulting semi-random weak hierarchy metrics, we can obtain a powerful and robust nonlinear metric model. This method has two primary contributions: first, it is semi-supervised, incorporating information from both constrained and unconstrained points. Second, we take a relaxed approach to constraint satisfaction, allowing the method to satisfy different subsets of the constraints at different levels of the hierarchy rather than attempting to simultaneously satisfy all of them. This leads to a more robust learning algorithm. We compare our method to a number of state-of-the-art benchmarks on $k$ -nearest neighbor classification, large-scale image retrieval and semi-supervised clustering problems, and find that our algorithm yields results comparable or superior to the state-of-the-art.
更多查看译文
关键词
Measurement,Training,Semantics,Data models,Clustering algorithms,Data mining
AI 理解论文
溯源树
样例
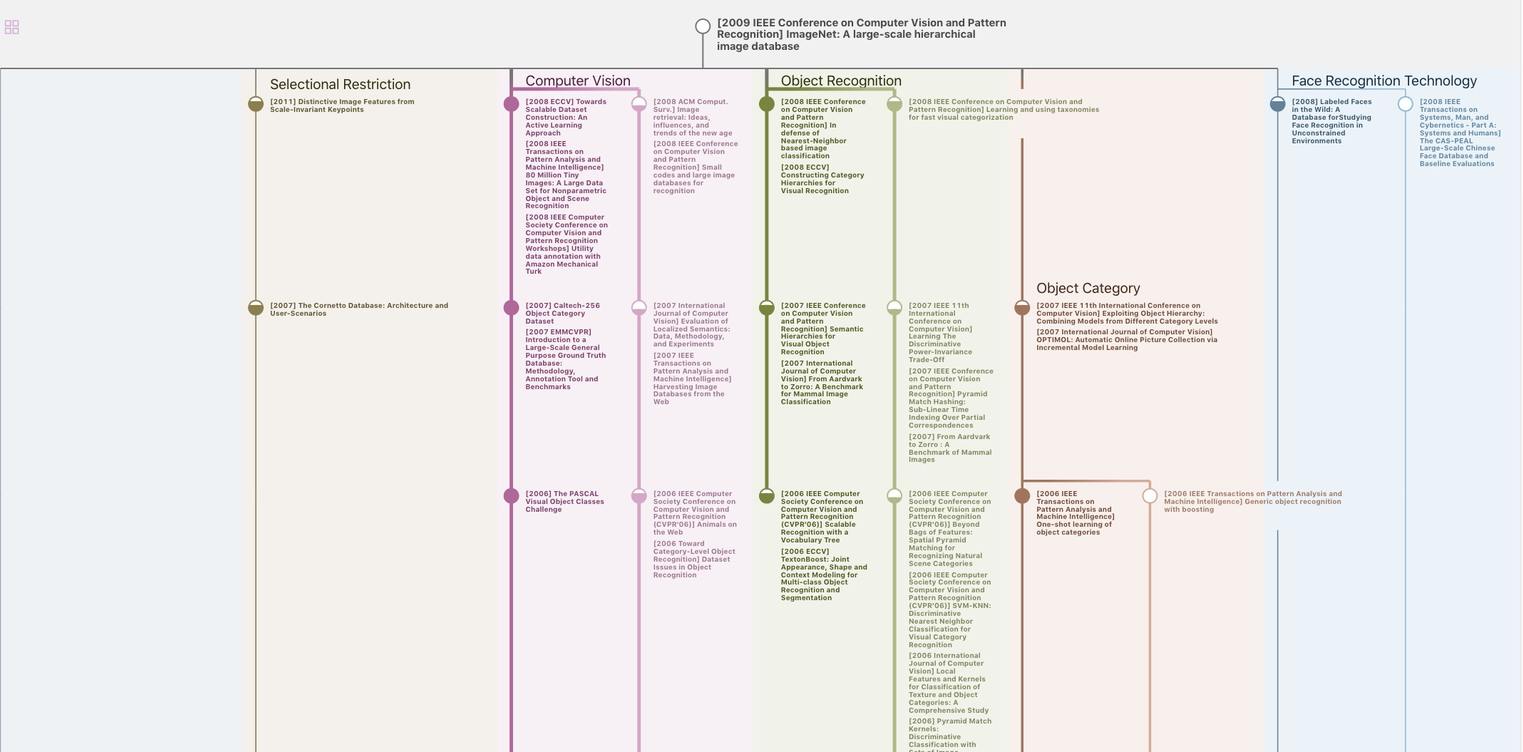
生成溯源树,研究论文发展脉络
Chat Paper
正在生成论文摘要