A robust realtime reading-skimming classifier.
ETRA(2012)
摘要
ABSTRACTDistinguishing whether eye tracking data reflects reading or skimming already proved to be of high analytical value. But with a potentially more widespread usage of eye tracking systems at home, in the office or on the road the amount of environmental and experimental control tends to decrease. This in turn leads to an increase in eye tracking noise and inaccuracies which are difficult to address with current reading detection algorithms. In this paper we propose a method for constructing and training a classifier that is able to robustly distinguish reading from skimming patterns. It operates in real time, considering a window of saccades and computing features such as the average forward speed and angularity. The algorithm inherently deals with distorted eye tracking data and provides a robust, linear classification into the two classes read and skimmed. It facilitates reaction times of 750ms on average, is adjustable in its horizontal sensitivity and provides confidence values for its classification results; it is also straightforward to implement. Trained on a set of six users and evaluated on an independent test set of six different users it achieved a 86% classification accuracy and it outperformed two other methods.
更多查看译文
AI 理解论文
溯源树
样例
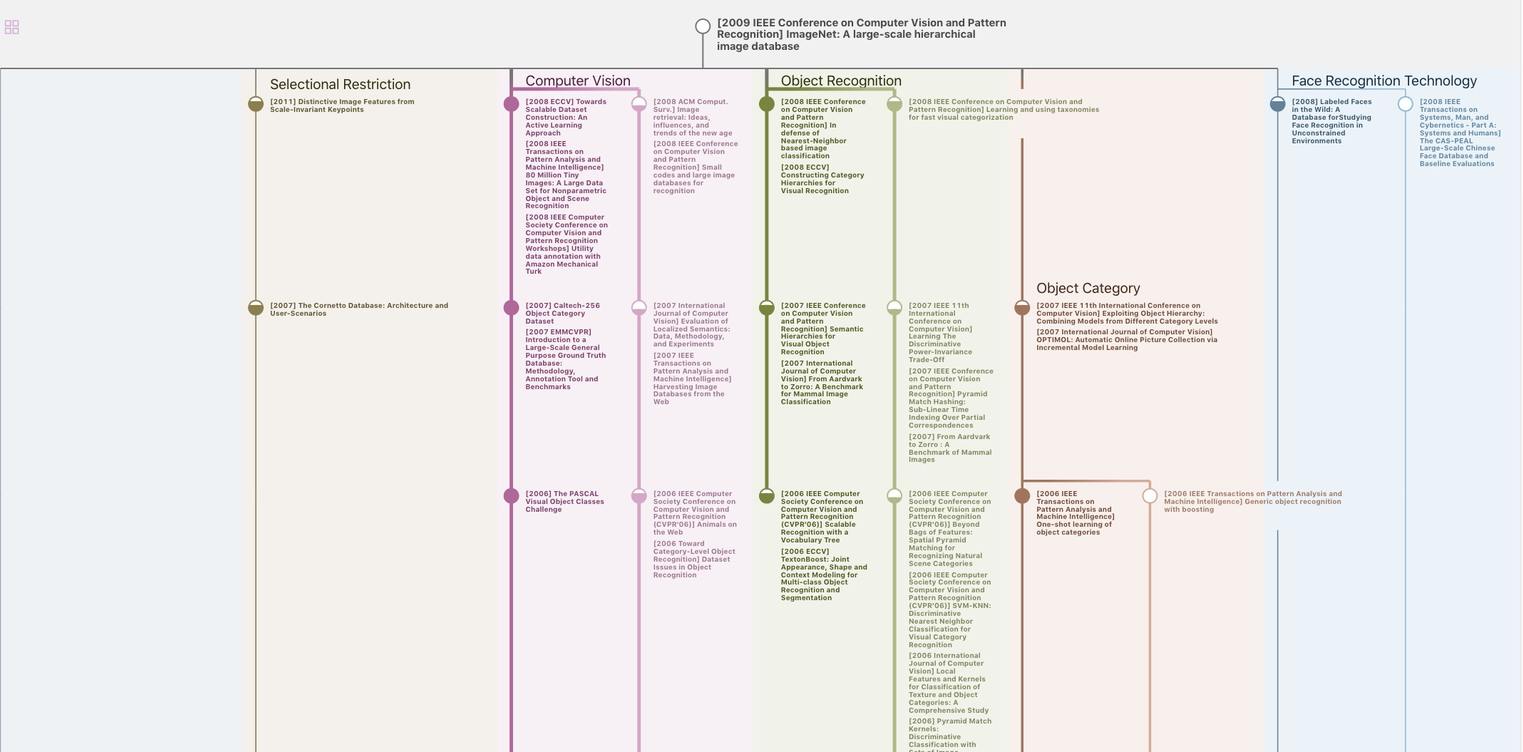
生成溯源树,研究论文发展脉络
Chat Paper
正在生成论文摘要