An inexact perturbed path-following method for Lagrangian decomposition in large-scale separable convex optimization
SIAM Journal on Optimization(2011)
摘要
In this paper, we propose an inexact perturbed path-following algorithm in the framework of Lagrangian dual decomposition for solving large-scale structured convex optimization problems. Unlike the exact versions considered in literature, we allow one to solve the primal problem inexactly up to a given accuracy. The inexact perturbed algorithm allows to use both approximate Hessian matrices and approximate gradient vectors to compute Newton-type directions for the dual problem. The algorithm is divided into two phases. The first phase computes an initial point which makes use of inexact perturbed damped Newton-type iterations, while the second one performs the path-following algorithm with inexact perturbed full-step Newton-type iterations. We analyze the convergence of both phases and estimate the worst-case complexity. As a special case, an exact path- following algorithm for Lagrangian relaxation is derived and its worst-case complexity is estimated. This variant possesses some differences compared to the previously known methods. Implementation details are discussed and numerical results are reported.
更多查看译文
关键词
smoothing technique,self-concordant barrier,Lagrangian decomposition,inexact perturbed Newton-type method,separable convex optimization,parallel algorithm
AI 理解论文
溯源树
样例
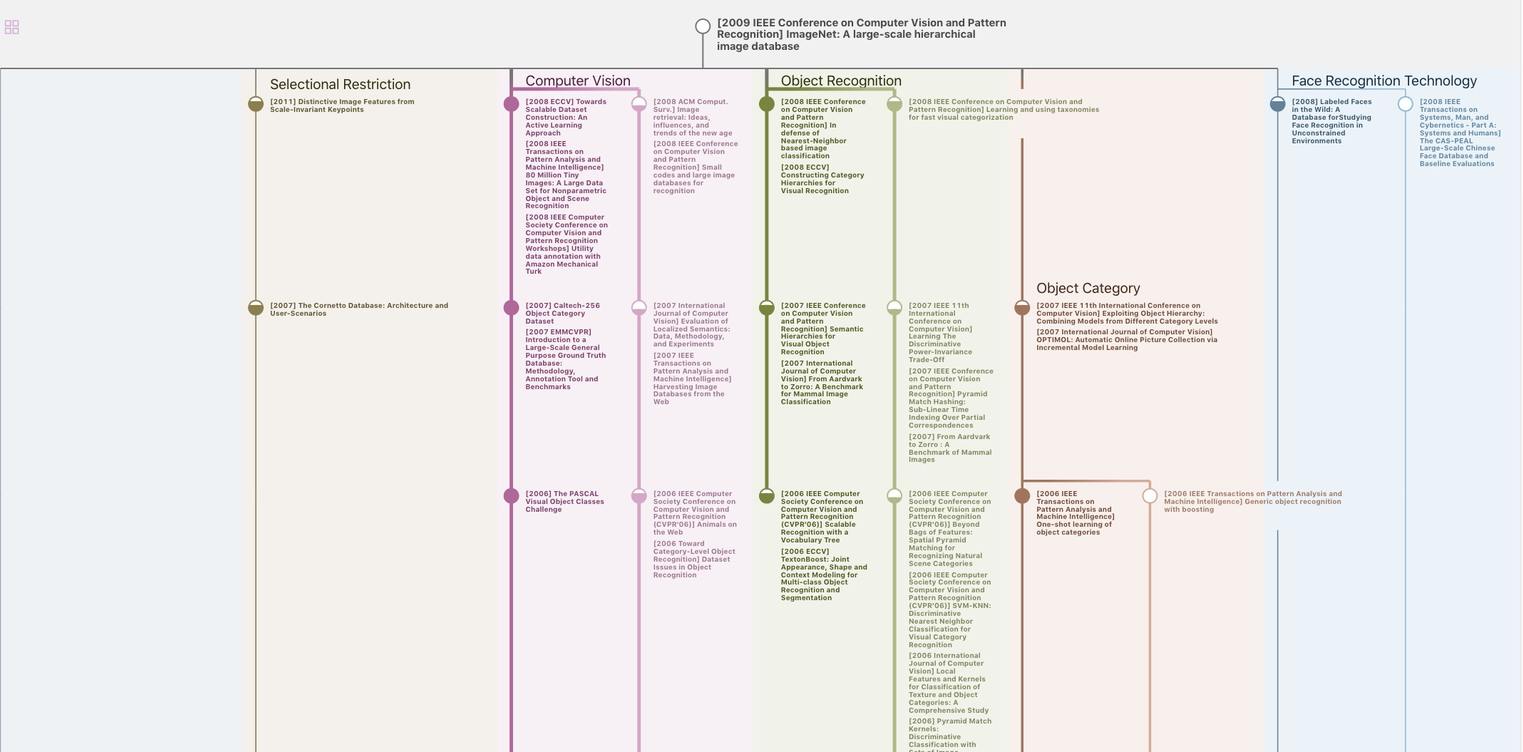
生成溯源树,研究论文发展脉络
Chat Paper
正在生成论文摘要