Hardware Implementation of a 32-In 32-Out Nonlinear Model of Hippocampal Circuitry for Cortical Implants
msra(2007)
摘要
Biomimetic prostheses for restoring/augmenting functions in damaged hippocampal regions require real-time evaluation of nonlinear multiple-input multiple-output (MIMO) models of hippocampal circuitry. This paper describes a prostheses suitable hardware implementation of a 32In-32Out model of the spike train activity between CA3 and CA1 sub-regions of the hippocampus. The 32x32 MIMO system is constructed using a series of multiple-input, single-output (MISO) compartments each of which have their input-output relationship described by Volterra kernels expanded using Laguerre polynomials as basis functions. First, second and third order kernels describe the nonlinearity within each input and second order cross kernel captures the interactions between inputs. The hardware flow features simple finite state machines (FSM) for control, memory bank for kernel coefficients and an elegant microcode based addressing for cross kernel coefficients. The model has been implemented and tested in FPGA. Current work to realize a system-on-chip (SoC) for implantable prostheses based on this model is in progress. The (SoC) will include the analog front-end blocks viz. Low Noise Amplifier (LNA) and Analog-to-Digital Converter (ADC) and a real-time spike sorter which provides the input to the MIMO model. Modeling spike train activity between CA3 and CA1 sub- regions of the hippocampus with a multiple-input multiple- output (MIMO) system has been described in (1). The MIMO system is realized as a combination of series of multiple-input single-output (MISO) compartments as shown in Figure 1. Each MISO system accounts for nonlinearities in the feedforward (K in Figure 1) and feedback (H) paths associated with the activity of a specific neuron. In addition, a noise term is also added to model intrinsic neuronal noise and other indirect contributions. The nonlinearities of the feedforward and feedback paths are modeled by Volterra kernels expanded using Laguerre polynomials as basis functions. Second and third order kernels are required to successfully predict CA1 output spike activity based on CA3 input spike trains. The feedforward block accounts for the contributions to 'u' from nonlinearities within each input and contributions to 'u' from the nonlinear interaction between a pair of inputs. The feedback term ('a') is implemented as a first order kernel and the noise term ('n') added is Gaussian with zero mean. The sum ('w') of contributions from each of
更多查看译文
关键词
input output,first order,laguerre polynomial,system on chip,second order,finite state machine,low noise amplifier,real time
AI 理解论文
溯源树
样例
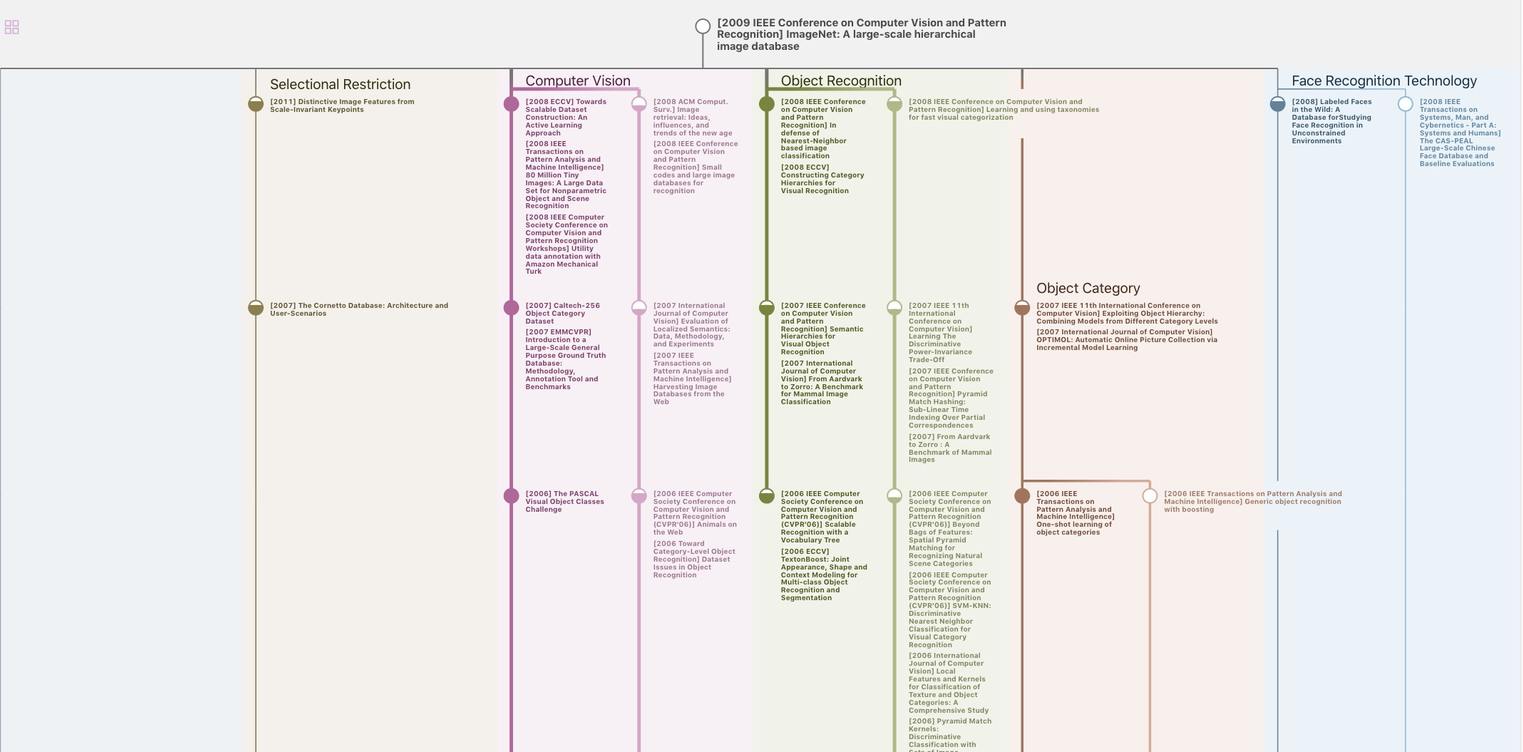
生成溯源树,研究论文发展脉络
Chat Paper
正在生成论文摘要