Population Model-Based Inter-Diplotype Similarity Measure For Accurate Diplotype Clustering
Journal of computational biology : a journal of computational molecular cell biology(2012)
摘要
Classification of the individuals' genotype data is important in various kinds of biomedical research. There are many sophisticated clustering algorithms, but most of them require some appropriate similarity measure between objects to be clustered. Hence, accurate interdiplo-type similarity measures are always required for classification of diplotypes. In this article, we propose a new accurate inter-diplotype similarity measure that we call the population model-based distance (PMD), so that we can cluster individuals with diplotype SNPs data (i.e., unphased-diplotypes) with higher accuracies. For unphased-diplotypes, the allele sharing distance (ASD) has been the standard to measure the genetic distance between the diplotypes of individuals. To achieve higher clustering accuracies, our new measure PMD makes good use of a given appropriate population model which has never been utilized in the ASD. As the population model, we propose to use an hidden Markov model (HMM)-based model. We call the PMD based on the model the HHD (HIT HMM-based Distance). We demonstrate the impact of the HHD on the diplotype classification through comprehensive large-scale experiments over the genome-wide 8930 data sets derived from the HapMap SNPs database. The experiments revealed that the HHD enables significantly more accurate clustering than the ASD.
更多查看译文
关键词
algorithms,statistics,strings,suffix trees
AI 理解论文
溯源树
样例
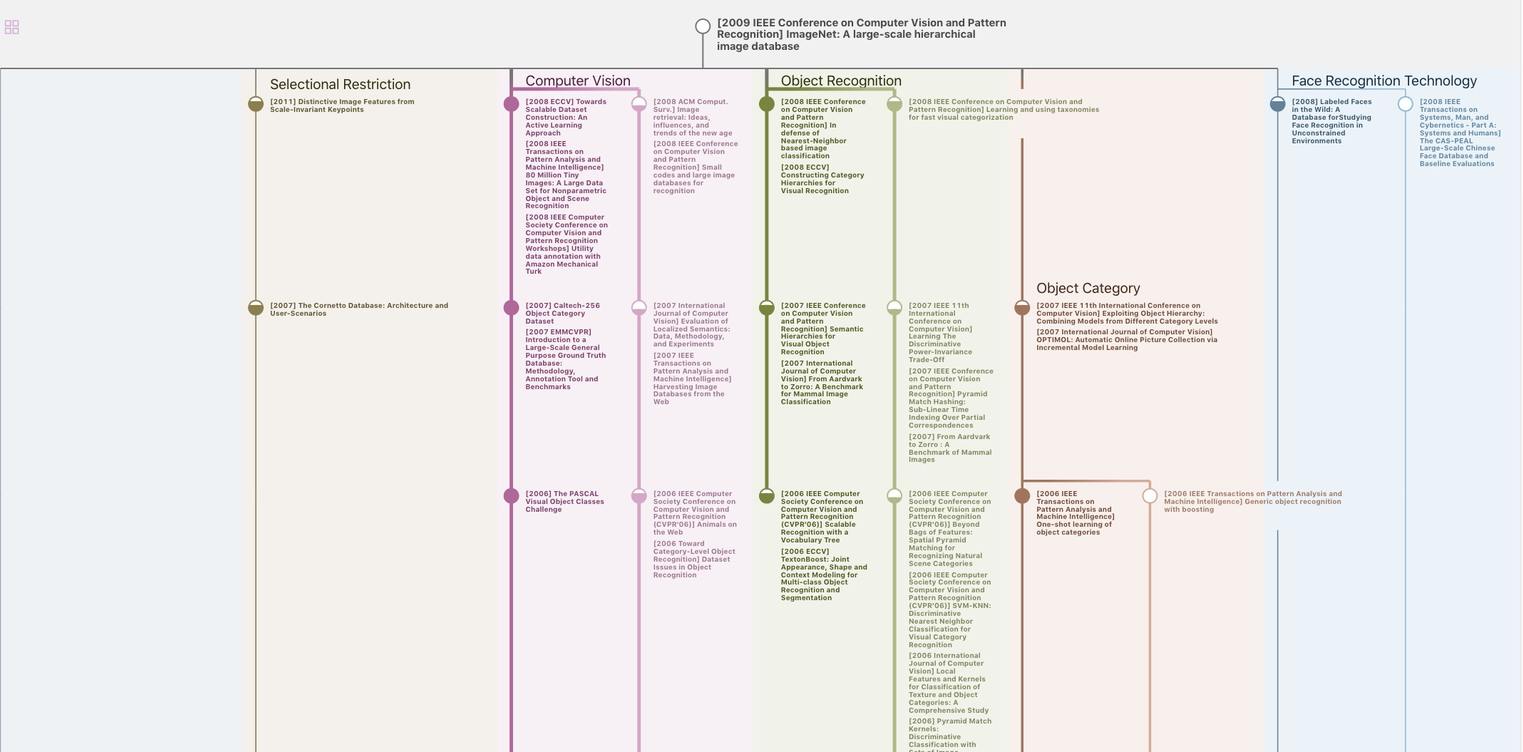
生成溯源树,研究论文发展脉络
Chat Paper
正在生成论文摘要