Sequential multi-sensor change-point detection
Annals of Statistics(2013)
摘要
We develop a mixture procedure to monitor parallel streams of data for a change-point that affects only a subset of them, without assuming a spatial structure relating the data streams to one another. Observations are assumed initially to be independent standard normal random variables. After a change-point the observations in a subset of the streams of data have non-zero mean values. The subset and the post-change means are unknown. The procedure we study uses stream specific generalized likelihood ratio statistics, which are combined to form an overall detection statistic in a mixture model that hypothesizes an assumed fraction p0 of affected data streams. An analytic expression is obtained for the average run length (ARL) when there is no change and is shown by simulations to be very accurate. Similarly, an approximation for the expected detection delay (EDD) after a change-point is also obtained. Numerical examples are given to compare the suggested procedure to other procedures for unstructured problems and in one case where the problem is assumed to have a well defined geometric structure. Finally we discuss sensitivity of the procedure to the assumed value of p0 and suggest a generalization.
更多查看译文
关键词
parallel processing,expected detection delay approximation,random processes,approximation theory,mixture procedure,average run length,statistical analysis,procedure sensitivity,independent standard normal random variables,subset means,nonzero mean values,sequential multisensor change-point detection,post-change means,geometric structure,overall detection statistic,arl,parallel data streams,generalized likelihood ratio statistics,data handling,edd,mixture model,sensor fusion
AI 理解论文
溯源树
样例
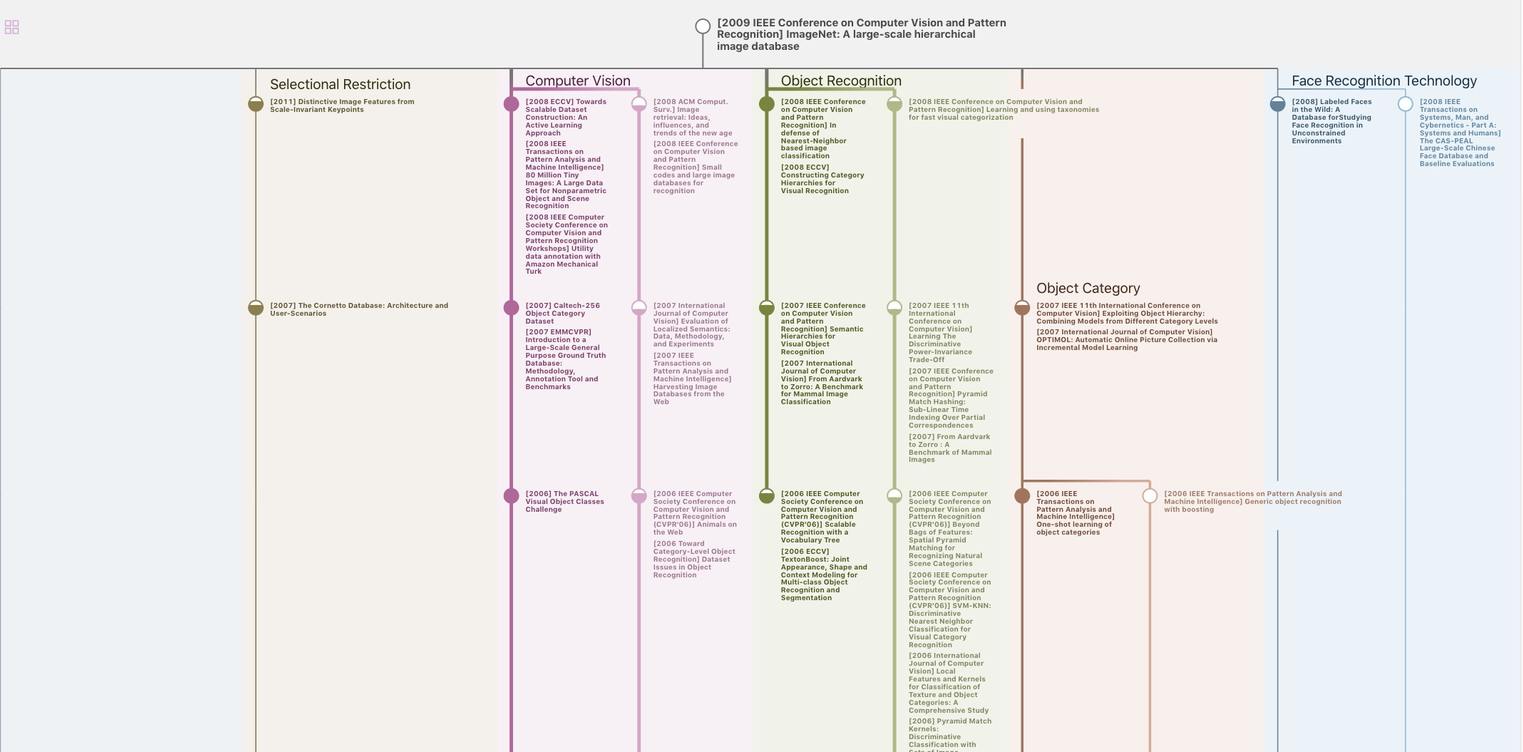
生成溯源树,研究论文发展脉络
Chat Paper
正在生成论文摘要