Object Class Recognition By Unsupervised Scale-Invariant Learning
CVPR (2)(2003)
摘要
We present a method to learn and recognize object class models from unlabeled and unsegmented cluttered scenes in a scale invariant manner. Objects are modeled as flexible constellations of parts. A probabilistic representation is used for all aspects of the object: shape, appearance, occlusion and relative scale. An entropy-based feature detector is used to select regions and their scale within the image. In learning the parameters of the scale-invariant object model are estimated. This is done using expectation-maximization in a maximum-likelihood setting. In recognition, this model is used in a Bayesian manner to classify images. The flexible nature of the model is demonstrated by excellent results over a range of datasets including geometrically constrained classes (e.g. faces, cars) and flexible objects (such as animals).
更多查看译文
关键词
Bayes methods,feature extraction,image classification,image representation,learning (artificial intelligence),maximum entropy methods,maximum likelihood estimation,object recognition,optimisation,Bayesian classification,entropy-based feature detection,expectation-maximization,flexible model,flexible object,geometrically constrained class,image classification,image region selection,maximum-likelihood setting,object appearance,object aspect,object class model,object class recognition,object modeling,object occlusion,object shape,parameter learning,probabilistic representation,relative scale,scale invariant manner,scale-invariant object model estimation,unlabeled cluttered scene,unsegmented cluttered scene,unsupervised scale-invariant learning,
AI 理解论文
溯源树
样例
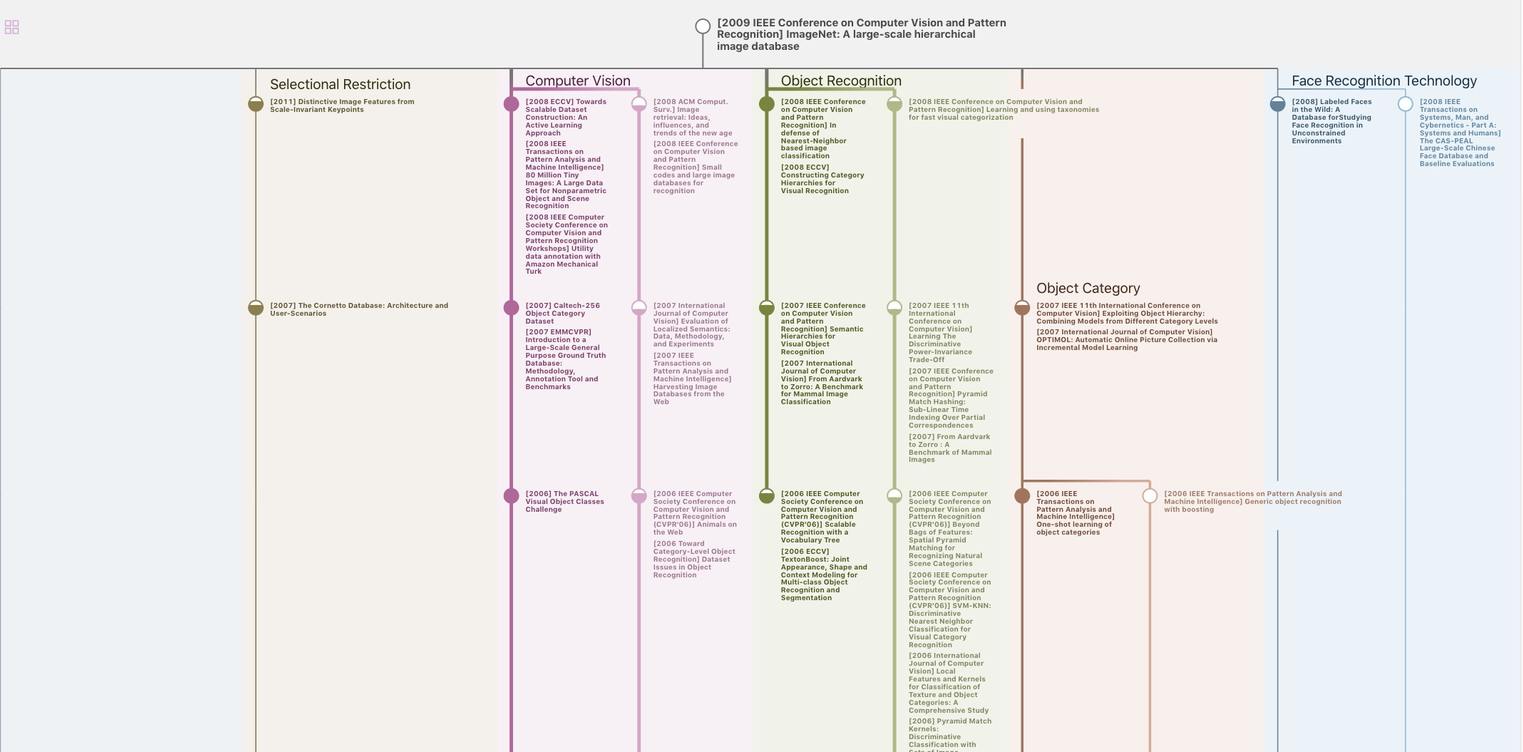
生成溯源树,研究论文发展脉络
Chat Paper
正在生成论文摘要